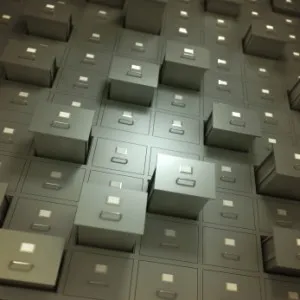
The last 2 to 3 years have seen enormous amounts of data generated from consumer interactions on the web. Corresponding market needs have evolved ranging from collecting interesting datasets to deriving analyses from it.Big players have already been part of this game in a way or the other.
Of late, the surge in cloud computing and other big data technologies like Hadoop and NoSQL have opened opportunities for newer players too.
Distinctly, there are two type of needs arising in the big data space from the perspective of an existing enterprise -
a. Consumer web companies who do brand monitoring, market research, etc. are connecting with micro-influencers in the community, this could be someone like the person who recommends you a mobile phone model, when you go to purchase one -- using data and are monitoring customer sentiments via the feedback channel.
b. Large businesses are focusing on insights from big data and ultimately using it either to identify newer avenues for business or optimizing existing solutions to cut down on costs.
When such humongous data needs to be crunched to extract meaningful information, a DaaS offering becomes extremely relevant.
So what exactly is DaaS?
It’s essentially any data, provided as a service in the B2B model. It could be the consumer footprint on the web, data from experts in pharma, energy or lot of internal data particularly in case of telecom companies, retail chain or banks. It’s similar to its other “aaS” counterparts, but instead of an infrastructure or software or any other device-based application, the provision here is data of interest. This is different from big data analytics because DaaS means facilitating the data on top of which you could do various kinds of processing/analytics. Although the entire system that facilitates this data is abstracted from clients, the complexity that’s inherent is formidable. A common question asked is “how is it different from analytics”? Simplest way to look at it is as a horizontal layer below it.
Credit to this new concept goes to cutting-edge technologies in the cloud using which even the smaller players like us (PromptCloud) have incubated in the Big Data space. DaaS is still in its infancy though where providers are growing gradually and exploring their ways with innovative offerings, apart from the standard product price feeds or user reviews. One such solution is our low-latency crawl offering, which can be used vertical agnostic-ally. This involves acquiring data from thousands of sources in near real-time, structuring it and then indexing it to allow low-latency feeds for our customers.
A DaaS model
For most DaaS providers, business is based on a recurring revenue model where clients would like to get regular data feeds to be integrated into their solutions. For example, our platform where we deal with large-scale crawls on the web and format that data in a structured format. The final deliverable to clients is structured data which gets delivered via our REST-based API in an automated manner regularly. Various other Big Data solutions could be provided on top of this, data-hosted indexing being one of them. Hosted indexing essentially means enabling search on the acquired structured data. This data could be either what we upload on our API or your own enterprise data. This is useful when say, as a brand monitoring firm, a team that’s only focused on Nike would like to query this dataset with how many said “good” and how many mentioned “bad” in their comments.
A typical day in a DaaS scenario is a mix of activities. You got to manage all your distributed systems and always keep optimizing them using most recent technologies. Also taking note that no design decision taken should be such that at some point inhibits scalability. Above that, you need to ensure all your machines are running smoothly in the cloud and in case any of those makes noise, you’d like to be alerted immediately and take action. Above all, since you’re a service provider, you should give no less than 200% in terms of support and attend to any data issue even before it arises.
DaaS Use Cases
Consumers in the DaaS space range from market research firms that collect sentiments across channels, to travel review aggregators who provide a single platform for all travel-related information, keeping off from the noisy data. But it’s interesting how use cases get invented on the fly from client requirements. Unusually enough, we had a client from Hollywood who ran a celebrity-gossip website and wanted to automate the data gathering process. The company had a good number of editors who Googled the web manually with a set of keywords that they sought gossips for. For obvious reasons, this process was not to scale. We implemented components within our existing platform and launched the mass-scale document-level crawl mechanism within our system that could look within any website and match for a set of keywords and still deliver structured data in an XML/CSV or on a web interface.
Wondering about the challenges?
- There are considerable technology barrier in this field but it is no less on the business front. It’s difficult to explain the solution to most of the prospects because everyone is so used to seeing a visual demo with wowable UI’s. Unfortunately or not, for DaaS providers, most of the processing happens in the background where multiple servers play the game of data processing and job scheduling with each other, and refuse to show much other than messages/ exceptions. So you have to be extremely creative and thoughtful if you’d like to put an interface layer on top.
- When it comes to dealing with data on the web, there’s yet no common standard and each web source is designed in its own way and changes as it likes. Standardized DaaS solutions for web is hence a difficult problem and a solution can be achieved with only approximate results. Thus, although a DaaS platform can handle different requests, there still needs to be some customization in place, especially if structured data is the deliverable. For example, our platform needed different set of components to take care of a customer who runs a search engine for used cars versus when another customer wanted to capture the hustle in the fragrance industry in a specific country. For the same reasons of differing complexities and resource requirements, costs cannot be fixed particularly for big enterprise needs (sometimes mistaken for lack of transparency in pricing).
In conclusion
When dealing with big data, DaaS helps reduce the time needed to get started by providing a layer to use below your service, considering build versus buy as apparent in any other service model. This provides a whole new way to look at your solution because your focus is on the top layer. A good analogy that comes to mind is what AWS has done to Netflix in terms of getting started in a new geography as well as facilitating day to day offerings. Larger enterprises are already clinging to DaaS providers. One of the keystones for Data as a Service is that external data can be re-used across companies. Such amounts of clean data intellectually stimulates towards some big data solutions that are yet undiscovered.
The author can be reached at @arpanjha10. She handles Product & Market Strategy at PromptCloud.com.