How deep learning could catch breast cancers that mammograms miss
Dr Dexter Hadley, of University of California, San Francisco, hopes to study five million mammograms to develop AI for precision imaging of breast cancer.
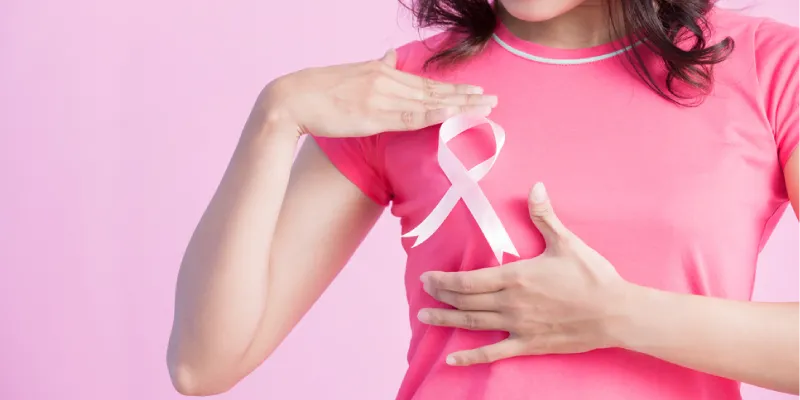
Mammograms save lives by detecting breast cancer early, except when they don’t.
Breast cancer is the most common cancer among women, and the second leading cause of cancer death.
But mammograms, the standard screening for the disease, miss one in five cancers, according to the US National Cancer Institute. Or they may indicate cancer when it’s not there — a false positive — forcing women to endure needless procedures and anxiety.
“In 20 years of collecting (digital) mammograms, we know they don’t work the way they’re supposed to,” said Dr Dexter Hadley, a professor of pediatrics, pathology, and laboratory medicine with the Institute for Computational Health Sciences at the University of California, San Francisco.
Hadley, who is an engineer as well as a physician, is working to change that. He and his colleagues at UCSF are using GPU-accelerated deep learning to improve mammogram accuracy.
When positive is negative
The UCSF researchers are training a neural network to accurately distinguish between benign and cancerous characteristics in breast X-rays.
“The idea is to catch breast cancer earlier than we can catch it now,” Hadley said.
He’s also working to reduce cases when something unusual in the mammogram triggers a cancer alarm — even though it’s actually harmless. More than half of the women in the US screened annually for 10 years experience false positives, which require further testing and often surgical biopsies.
In addition, two types of cancer seen in mammograms — ductal carcinoma in situ and small, invasive breast cancers — may not be life-threatening. For these cancers, doctors can’t easily determine those that need to be treated from those that don’t, according to the National Cancer Institute. As a result, some women undergo radiation, lumpectomies, and other treatments they don’t need.
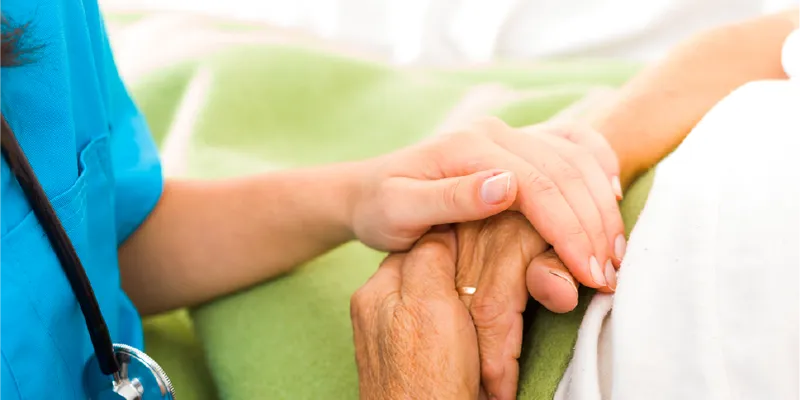
A computer reads a mammogram
To solve the problem, the UCSF team took over 30,000 written pathologist reports and used deep learning algorithms to infer how individual cancer patients fared. The researchers linked this data to more than 700,000 mammogram images.
Then, using GPUs with the cuDNN-accelerated TensorFlow deep learning framework, they trained a convolutional neural network to predict cancer diagnoses based on breast imaging.
When researchers tested the algorithm it matched radiologists’ performance on a particularly complex mammogram interpretation problem. Hadley envisions this work as a tool radiologists could use to make decisions.
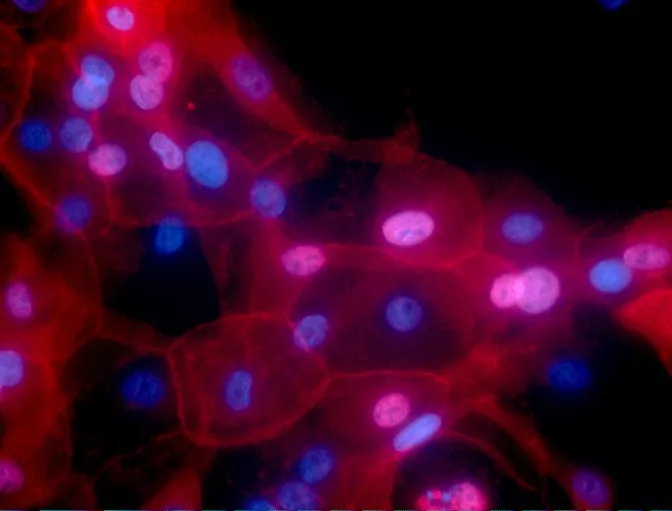
Donate your mammogram for research
Hadley next plans to develop a system that precisely identifies what it sees, such as whether it’s a benign growth or cyst, an invasive or non-invasive breast cancer, or whether it’s cancer that’s spread from other organs. He also aims to use deep learning to predict the rate of tumour growth.
That work may have to wait, Hadley said, until he gets access to the millions of mammograms needed to train a robust deep learning model. Although 40 million mammograms are collected yearly in the US, national health privacy law restricts access to them.
As a result, Hadley, UCSF, and their partners have put out a call to women to donate their mammograms or other breast imaging data for research. To participate, sign up at https://www.breastwecan.org/. Hadley’s also grateful to Western Digital, which donated petabyte-scale storage to support his work.
Hadley hopes to study five million mammograms to develop artificial intelligence for precision imaging of breast cancer.
Improve mammogram accuracy
Ultimately, Hadley’s goal is to use big data to provide more precise treatments tailored to individual patients.
“We’re trying to teach computers to identify cancer in mammograms, and then teach radiologists what features to look for in an image,” Hadley said. “The holy grail of our work is to improve the art of medicine overall.”
(Disclaimer: The views and opinions expressed in this article are those of the author and do not necessarily reflect the views of YourStory.)