Leadership teams, data scientists need to work together to build successful ML ops, Vipin Nair says at TechSparks 2021
In a Masterclass at TechSparks 2021, YourStory’s flagship event, Google Cloud’s Vipin Nair talks about how leadership and management teams can work together with data scientists to build successful machine learning (ML) models.
More than 87 percent of data science projects never make it into production, Vipin Nair, Machine Learning Specialist, Customer Engineering, Google, said, citing multiple studies, during a Masterclass ‘Building effective MLOps on Google Cloud’ at Techsparks 2021, Yourstory’s flagship event.
“Data science is more about the team as it is more about leadership as well as technology. You not only need the right algorithms but the right leadership team. Popular belief is companies, which adopt AI (artificial intelligence) and ML (machine learning) have a better edge than the companies that don’t. But only 13 percent of them are able to push it out successfully,” Vipin said.
In today’s technology-powered world, AI and ML are important for startups operating in social media, communication, and e-commerce, among other sectors, to predict patterns and customer behaviour.
An ML ops project has to deal with several hurdles. “Whenever a data scientist wants to get their work into production they need to hand over their projects to engineers. That’s where the problem starts,” says Vipin.
Initial problems include differences in coding languages. “For a typical ML implementation, R is the preferred language for data analysis and then it moves to Python and then goes on to Java or C++. When the same algorithms go into production then there is a problem because high levels of computes are required, which can also be expensive and come with their own set of challenges,” he said.
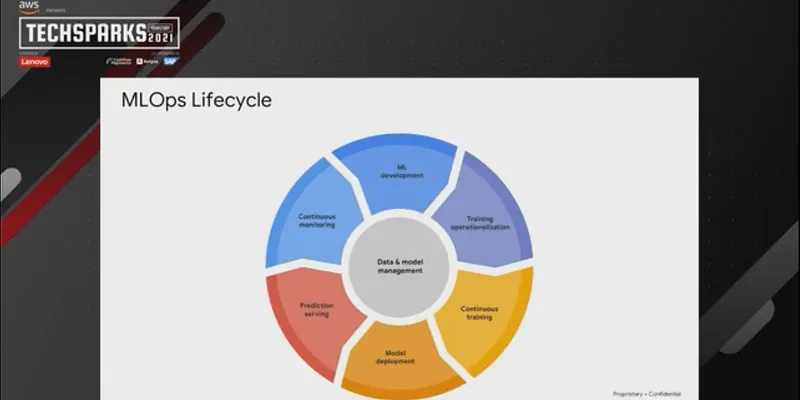
Effective ML Ops lifecycle
ML models also go through iterations and checks before they can be picked up by a business stakeholder.
“Without a robust data and ML engineering process, an organisation might not be set for success. An ML engineering task should not be performed or developed in isolation. It should be integrated with dev ops and other areas for better development,” Vipin said.
Earlier, ML ops teams followed what is called standard ML workflow or an ML pipeline, which consists of data ingestion, data cleaning, feature engineering, training model, model evaluation and deploying the model. According to Vipin, the workflow is only a part of the ML development lifecycle.
A more holistic approach involves continuous development, monitoring, and management.
An ML ops lifecycle includes ML development, training operationalisation, continuous training, model development, prediction serving and continuous monitoring. At the heart of the lifecycle lies data and model management. In the Masterclass, Vipin explained these processes in detail and how they affect the final ML outcome.
Also, ML ops teams have to keep scaling the model in mind as ML systems are moving towards automation and have to factor in new data, Vipin says. Too many manual steps in the workflow hinder ML engineering. To train models at scale, teams need to have batch predictions and online predictions.
In the Masterclass, Vipin also talks about the challenges that startups face when deploying an ML model.
“Carrying out these processes could be very expensive for an early-stage startup. And the risk is the model might not even scale if it is going to go through so many levels of iterations,” Vipin said.
If the teams are always fixing things then the ML model is never going to be ready for scale, according to Vipin.
To log in to our virtual events platform and experience TechSparks 2021 with thousands of other startup-tech enthusiasts from around the world, join here. Don't forget to tag #TechSparks2021 when you share your experience, learnings and favourite moments from TechSparks 2021.
For a line-up of all the action-packed sessions at YourStory's flagship startup-tech conference, check out TechSparks 2021 website.
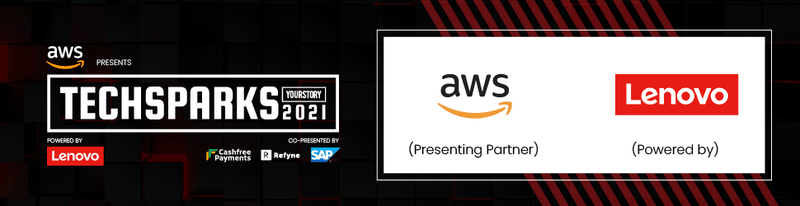
Edited by Affirunisa Kankudti