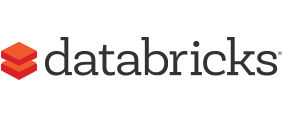
databricks
View Brand PublisherIs Gen AI helpful in making data-driven decisions?
Expert panellists discuss the potential of GenAI beyond standard use cases at YourStory’s Tech Leaders’ Conclave in Bengaluru, highlighting its prowess in making data-driven decisions.
Generative AI, or Gen AI, has taken organisations and customers by storm. While certain standard use cases of this new-age technology are much talked about, it is being used increasingly to make data-driven decisions.
However, while experiments with GenAI are in full flow, every organisation is impacted differently because of how this technology functions. Some believe it is starkly different from traditional AI.
To understand this better, expert panellists from the field of tech – Ashwin Sekar, Chief Product and Technology Officer, InCred Financial Services; Ramesh Kumar Katreddi, Vice President, Engineering, Jumbotail; and Kedar Gupte, Associate Director Engineering, Data Platforms, Myntra – were part of a session ‘Gen AI as a strategic lever for enabling data-driven decisions’ at YourStory’s Tech Leaders’ Conclave in Bengaluru on June 21. Rajesh Ramdas, Senior Director, Field Engineering, Databricks, moderated the panel discussion.
The impact of GenAI
Katreddi explained the functioning of Jumbotail, highlighting how the B2B marketplace serves as a retail platform where sellers can supply and kiranas can buy from them.
During this journey, from the customer's point of view, these kiranas are not tech-savvy and communicate in vernacular languages. Most experiences designed for them are textual. However, GenAI's multi-modality has opened up possibilities for them around vision and voice capabilities.
“This has also helped us join the bandwagon and experiment a lot more. We realised there are some click-to-rate (CTR) improvements through Generative AI,” Katreddi shared.
InCred, on the other hand, is a financial services platform that lends to small and medium businesses. Apart from standard use cases, it is using GenAI to onboard employees.
“We have been growing by 500-600 employees a year. There's an internal bot to answer questions from employees. That's helping us prevent tribal knowledge from getting locked up in people's heads. Interestingly, we are also trying to detect fraud. It's a hard problem to solve in a commercially viable manner with acceptable false positive rates,” Sekar said.
InCred has been experimenting with GenAI to synthetically create fraud lend datasets and improve its machine language (ML) models' ability to recognise and block fraud.
There are different applications of GenAI on search personalisation in Myntra, particularly in the ecommerce piece. However, Gupte explained that the easiest ones to implement and those with the highest return on investment (ROI) are on developer productivity and optimisation.
“As a data platform, we would want democratisation and maximum users to leverage our platform. But that comes at the cost of suboptimal queries being written. Optimisation is hard but where GenAI can shine is it can go through the query plan and figure out the right optimisation strategies,” he added.
Dealing with challenges
One of the biggest challenges that most companies face is trusting the large language models (LLM). On this, Sekar believes there's no replacing the hard work of sanity checks or validation of data quality. The idea is to create the lineage and definitions of the columns and tables to increase the output of LLMs.
Katreddi said his organisation is at the stage of training images and annotating them. Over time, he realised LLMs can be utilised to classify images that are required for annotation, ensuring data quality is not compromised.
On the subject of data democratisation, Gupte shared that it is an important architectural choice for the adoption of AI or GenAI. That's because it is an LLM model that is efficient at figuring out pieces of information and the relationship between those pieces of information from a vast pool of data.
“It needs to be trained on that information and, in the data-driven context, what that information is. It is essentially the set of tables, what data it contains, what is the meaning, how is the data moving between these tables, what is the volume of data, etc. We call this the information graph,” Gupte said.
This also gives LLMs the ability to query different sources of data. This has helped create more use cases and eventually translated into higher revenue generation.
Experimentation to production
Sekar shared that InCred is still in the experimentation mode. However, there's one lesson that will stand it in good stead. Initially, they were experimenting with different LLMs, but eventually realised good engineering principles are the key to success.
“Structure yourself for fast feedback loops and if you can speed those up, you can learn faster and get your LLM outputs working much faster,” he said.
There's still time to reach large-scale production deployment of LLM, revealed Katreddi of Jumbotail. From a research perspective, he has been following processes for testing the correctness of LLM.
Citing an example, Gupta explained how there are several pages and interaction points in the ecommerce space. Getting the right model and recommendation is a function of how good the data is and how well trained the model is on the data.
“The story is also about having fine-tuned models for specific customer cohorts. There should be analysis continuously to get to the final KPIs. Lastly, the pipelines fuelling these models have to be very efficient and adhere to quality constraints,” Gupte concluded.