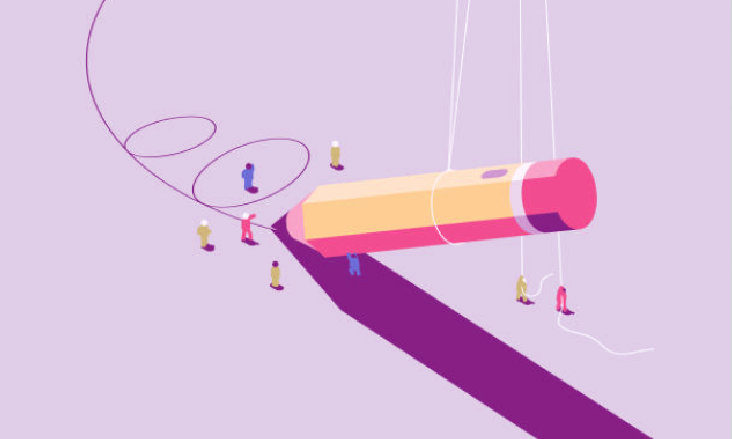
Going hand-in-hand: big data and banking
Banks are digitally transforming themselves at a fast pace with advanced branch less technology and contemporary services.
Banks are digitally transforming themselves at a fast pace with advanced branchless technology and contemporary services. The latest buzzword in the fintech industry are chatbots which have been adopted by almost all leading banks to make their customer service readily available to clients round the clock. So now, what is next? Big Data?
But banks across the world are already using data analytics to upscale their business. Hover, tech experts believe that banks are still to realize the full potential of Big Data. While the BFSI sector creates an enormous amount of data every second, is it able to mine this voluminous amount of information?
Maybe it is time, say some. Big Data that is defined by the volume of data, a variety of data and velocity of processing the data presents big opportunities for financial institutions. Many of these have even transformed themselves with the help of data mining that eventually helps in quick, easy and apt decision making. While banks have been slow in the adoption of this technology due to the confidential nature of its data, the trend is seeing a positive change. Let’s take a look at some advantages of deploying Big Data techniques in banking:
1. Risk Management
While all businesses need to engage in appropriate risk management, in banking industry this practice warrants extra attention. Big Data coupled with Business Intelligence can provide vital insights to banks on risks of approving loans to potential customers’ post evaluation of portfolios. Big Data can likewise help in early detection of fraud since it locates and presents data on a single scale making it simpler to mitigate the count of risks to a controllable number.
While improving the projecting power of risk models, big data also lowers system response times and increases effectiveness. Also, along with wide risk coverage, analytics also cause vital cost savings by generating more automated processes and precise predictive systems and less failure risk. It can positively impact fraud management, credit management, loans management, operational risks, and integrated risk management.
2. Compliance
A heavy regulatory framework dictates the working of financial services so as to form a shield of protection against frauds and misuses. Big Data can play a crucial role in conforming adherence to regulations.
It can identify and patch vulnerabilities, thereby strengthening and fortifying all materials of data governance and compliance. It can likewise help create a baseline for ‘standard’ operations, which gives organizations ahead begins in recognizing extortion and enables supervisors to spot consistency and administrative issues previously they turn into an issue.
3. Customer Experience
American worldwide management consulting firm McKinsey Company says that marketing productivity can be boosted by 15-20 per cent if companies use data and Big Data to make better marketing decisions. From ‘Product is King’, BFSI strategies now focus on ‘Customer is King’ and it has become important to focus on what they need and expect from a bank and financial institutions.
To understand this, just a few customer snapshots won’t make the deal, a data hub needs to be created with ALL information about the customer and his interaction with the brand like personal data, transaction history, browsing history, service, and so on.
These customer insights generated by data-based analytics can empower the BFSI sector to segment customers and target them with appropriate material.
4. Fraud Detection
Banks also, monetary administrations can and are already using Big Data analytics to distinguish between fraudulent activities and genuine business transactions. investigation and machine learning can both help determine standard movement in view of a client's history and differentiate it from unordinary conduct demonstrating extortion. The investigation can also suggest remedial activities, for example, blocking crooked transactions, deriving from actions taken in past. It will not only stop misrepresentation before it happens but will also improve profitability.
5. Employee Engagement
What your employees feel about working in your company has a lot to do with what your end customers will experience. A higher level of satisfaction among employees will also extend to your customers and will push business growth. Big Data can help companies look at real-time data and not just annual reviews which are usually based on human memory. With the correct tools in place, companies can measure everything from individual performance, team work, inter-departmental interaction, and the overall company culture. When the data is related to customer metrics, it can also enable employees to spend less time on manual processes and more time on higher-level tasks.
Challenge
While there are many positives to making use of Big Data analytics in the BFSI sector, the huge amount of data that is being generated by a wide variety and number of sources poses a big challenge. A study says that the digital universe is expected to reach 44 zettabytes (that's 44 trillion gigabytes) by 2020. Thus, imagine the amount of data that is going to be generated. Super software and computers will be needed to process such information that can halt legacy systems.
Conclusion
Once the sorting is done and useless data can be justifiably thrown out, the remaining crucial data can help banks grow from leaps to bounds. Besides, helping banks deliver better services to their customers, both internal and external, Big Data is also helping them improve on their active and passive security systems.
Big Data is already playing a role in the banking sector with many banks and financial institutions capturing customer related data for sentiment analysis, starting from social media websites to various market research channels.
Transactional analysis is being used to fathom spending patterns of customers, assess consumer behavior based on channel usage and consumption patterns and segment consumers depending upon the aforementioned attributes, and identify potential customers for selling financial products.
Most of these findings can be applied easily into fiscal systems of banks aiding them to reinforce data security and avoid any type of attack. A combination of many such transactional and sentimental gauges can help banks arrive at a holistic decision-making approach and thereby implement erudite machinery, a need of the hour for the banking sector.
https://www.esds.co.in/blog/category/big-data/
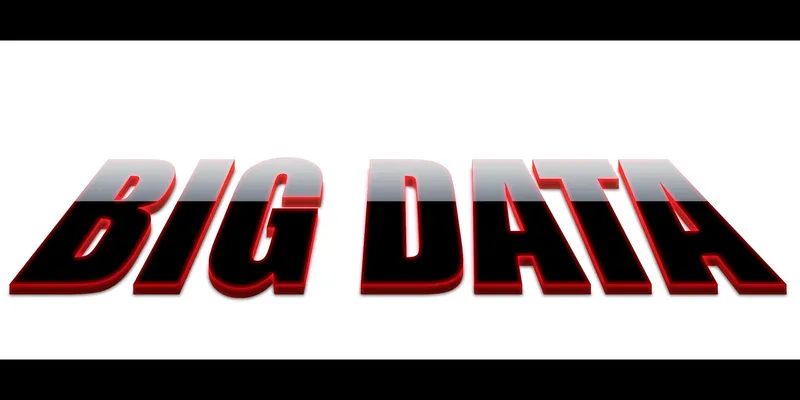
Big Data