Understanding Big Data from one of the big guys - Amr Awadallah, CTO, Cloudera
Big Data has been a popular buzzword over the last few years. But for those not directly working on it, Big Data has always been associated with big confusion.
What exactly is Big Data? What problems does it help solve? Why is it important for me?
Many have struggled to explain the concepts.
In an Accel Partners event on Big Data, co-hosted by YourStory, we heard a great outline from one of the big guys himself.
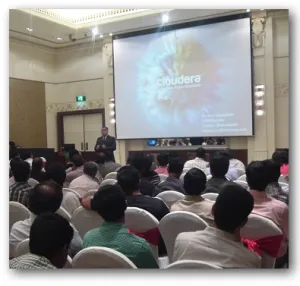
Amr Awadallah is the CTO of Cloudera, one of the most talked about companies in the Big Data space. Cloudera was founded in 2008 by three top engineers from Google, Yahoo and Facebook (Christophe Bisciglia, Amr Awadallah and Jeff Hammerbacher, respectively) and a former Oracle executive (Mike Olsen). Accel Partners invested $5mn in 2009. Cloudera raised further funding in two rounds from Ignition Partners, Accel Partners, Greylock Partners, Meritech Capital Partners, and In-Q-Tel in 2011 and 2012 to the tune of $140mn, valuing the company at $700mn. Cloudera boasts of an impressive list of customers using its services: AOL, CBS, eBay, Expedia, J.P. Morgan Chase, Monsanto, Nokia, Research In Motion and the Walt Disney Company.
"Think of a cooking process", said Amr, drawing an analogy to what Big Data is about.
"You could have two ways of preparing food.
"In one, you have a guy who’s very fast at chopping meat, vegetables and choosing ingredients. Before your chef comes in, he keeps everything ready. The chef now can cook up a recipe with the chopped items and ingredients.
"This is how regular data processing works. You know the formats that data is available in, and can come up with analyses based on the structure." said Amr.
Taking the analogy further, Amr explained, ‘The alternate, and more interesting case, is when the the first guy just leaves all the meat, raw vegetables and ingredients for the chef. The chef has a larger base of ingredients to choose from, but also more complexity in deciding what to cook. The dish is not determined by the ingredients someone else has chosen. He can now explore. As he experiments, he finds his favorites. He can then ask the first guy to chop vegetables just for those to make the process faster.
"Similarly, Big data works on unstructured data sets. It helps find interesting patterns in a mesh of data. There’s more innovation, as the questions are not predetermined. If there are patterns that emerge, they can then be handled by structured data sets. Big data lets you discover patterns by querying multiple data sets.
Agility/Flexibility of Big Data
Think of the admission form you would have filled while joining college. There would be fields for name, age, marks for previous exams and a variety of other forms. Each data field is structured, and a clerk would then input them into a database. This lets him query for any of the structured fields.
This is a typical schema-on-write or RDBMS (relational database management system) field, otherwise known as prescriptive data modeling. To build such a system, you would create a static DB schema that can hold fields, capture the data, transform it into a format for the RDBMS, and then be able to run specific queries on it.
If you add a new field or change a field, the RDBMS has to be changed to incorporate this. So you might end up having multiple databases over the years that may not be compatible.
In contrast, think of the application letter you write to get in. You probably talk through some of your achievements in school, competitions you have won, etc.
There is no defined schema for this. In older days, the college clerk would have to read each of the application forms and then copy or highlight info for the admission committee to read. They would then have to sift through the data. If they wanted to check which of the applicants played cricket in school, the poor clerk would have to run through the applications again, highlight the ones where the candidate has played cricket (and leave out candidates who just like cricket).
This is a typical unstructured query problem; one that Hadoop tries to solve.
Apache Hadoop is a schema-on-read system; also called descriptive data modeling. You can build a system where you copy data to the system and create schemas and parsers on the fly (e.g.: find people who have scored over 70% in their XII exam and who play cricket). Data can be queried in its native format. Any new data can start flowing in; schemas and parsers can be written to query it retroactively.
Structured data sets (RDBMS) are great for finding the known unknowns, i.e. get answers for items you know are in the data in a structured form.
Big data is great for finding the unknown unknowns (pardon us, Mr. Rumsfeld!). The analysis is exploratory in nature. If there are interesting patterns that emerge, we could check if they could be queried by structured data queries (e.g.: if we find there are many cricket players from the application letters, we could add a field in the admission form that asks if the candidate plays cricket).
Scalability benefits
Big data lets your data analyses scale flexibly. Facebook stores over 150 petabytes of data in a single Hadoop instance.
Big data systems like Hadoop take away a lot of pain from managing infrastructure: if you need more space for storing data, or more processing power, you can dynamically plug in additional machines for this: no need to rewrite software later.
This also allows for lesser number of system administrators. A single system administrator can manage a cluster of hundreds of servers.
Systems are also intelligent enough to signal when nodes fail, transfer the data to other machines, or shift the processing of data to other systems. A lot of manual work of identifying failure points is thus saved.
Benefits of economics
A key metric that data storage industries use is Return on Byte: the ratio of Value of Data to the Cost of saving data.
To optimize this metric, you would traditionally keep fresh data on your active systems, and move the older data to archives.
Here’s an analogy.
Say you love clicking pictures using your phone.
For a minute, think of the time when your phone could not upload data directly, but could only connect to your computer.
As your memory card fills up, you realize that you will need to buy a larger card to store more pictures (additional cost), or move the files to your computer hard disk (archive).
If you have archived data, you lose opportunities of showing off earlier photos when you are with a friend (remember, no cloud syncing yet!). That’s value lost.
Businesses face similar problems.
Being able to mine historical data has huge value, but as costs in storing data in traditional systems are too high, most organizations archive older data. Once data is archived, it is usually extremely difficult and/or expensive to run structured queries on it.
"Data goes to tape archives to die", joked Amr. "Only an act of God or act of government can rescue it".
Hadoop turns this on its head.
With Hadoop, you can maintain active archives, so you can query historical trends just as easily. The question becomes not what you can store, but what you can do with the data. It facilitates a culture of abundance about data, and not one of scarcity.
With Hadoop, the cost of transactions on data is approximately a tenth of what it would take to build traditional systems. Hadoop is also designed to bring down query times significantly.
Hadoop also integrates with many of the traditional data warehousing apps, allowing companies to mine information they already hold without having to reformat this.
Applications and future of Big Data
With these benefits, Hadoop has opened up significant new use cases for various industries. Financial companies can run complex risk and portfolio analyses; online services and social media companies can try matching people and careers, or optimize websites based on data; oil and gas companies can improve drilling exploration using sensor analysis, and much more.
As an example, consider how Big Data helped one of the larger credit card companies detect fraud. The company had a lot of historical transaction data, but was running out of capacity on their structured database. Using Big Data they were able to store many years worth of historical data. The new system also helped add a lot of new attributes to the data, without having to worry about the overheads of maintaining or changing previous data systems.
In addition, the firm was able to run queries on massive amounts of current and historical data that helped it discover a huge credit card breach of someone siphoning off extremely small amounts from millions of transactions.
As organizations increasingly turn to Big Data, there is a huge demand for help in this section.
Cloudera makes its money in a similar manner to Red Hat. The core software is all open source and freely shareable. However, they make money from enterprises that need SLAs, training, pilots or need their deployments certified.
It’s probably fair to say that we’re just at the tip of what Big Data enables. The future looks exciting, as we get more intelligent about our systems. Big Data is surely here to stay!