Big Data at work: key lessons from startups and large firms
Ten years ago, Silicon Valley startups powered the rise of the Big Data movement, and a new generation of startups as well as large companies are now taking Big Data to new frontiers and applications.
Babson College professor Tom Davenport, author of bestsellers in knowledge management and analytics, provides a range of frameworks and case studies of Big Data startups and deployments in his latest book, BigData @ Work: Dispelling the Myths, Uncovering the Opportunities.
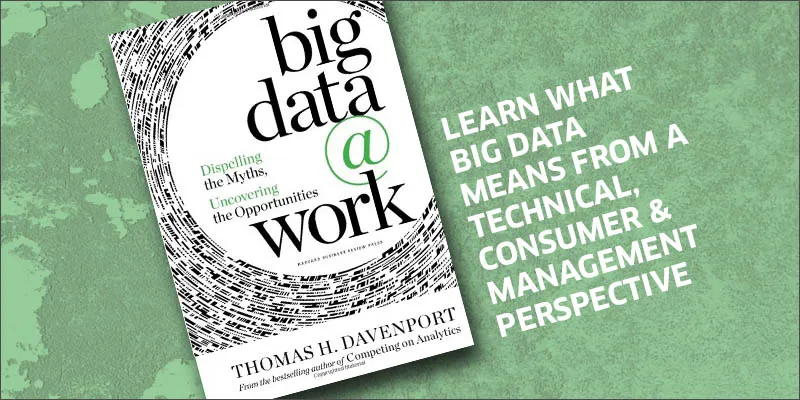
“Big Data refers to data that is too big to fit on a single server, too unstructured to fit into a row-and-column database, or too continuously flowing to fit into a static data warehouse,” defines the author. The real business benefit in harnessing Big Data is in converting it into insights, innovations and business value.The field opens up new opportunities for companies due to the explosion in external sources of data (Web, social media, mobiles, Internet of Things, customer calls) and rise of sensor networks (in industrial, warehouse and supply chain settings). It is not just the volume but variety and velocity of data that can unlock new value, and requires companies to operate in real-time in constructing models, discovering new insights and devising new market strategies in an agile manner.
The author traces the evolution of the industry from decision support systems (1970-1985), executive support (1980-1990), OLAP (1990-2000), business intelligence (1990-2005), analytics (2005-2010) and Big Data (2010 onwards).
Emerging trends to watch include auto-analytics or personal analytics as humans become more ‘sensored’ with health devices and mapping tools, such as Nike+, Garmin Connect, Zed9, CycleOps, Concept2, FitBit, MyZeo, WakeMate, BodyMedia and Me-Trics.
The material makes for an informative read and is targeted at senior managers and leaders, as well as startups looking for new insights; technical analysts will probably know most of the frameworks already. I have summarised the key takeaways from the eight chapters in Table 1 below.
Table 1: Mapping the Big Data wave
“Much of the earliest Big Data activity was in firms with online products and services – Google, eBay, Yahoo, Facebook, LinkedIn and the other usual suspects – and startups in online and related sectors. We owe these organisations considerable debt because they established the function of data science and other disciplines of Big Data,” the author explains.
The booming field has created a huge demand – and scarcity – for qualified and well-rounded data scientists. Many companies now have their own training programmes for data science, such as EMC, IBM and Deloitte. Offshore firms such as MuSigma have thousands of ‘quants’ as employees (also see earlier YourStory coverage).
Google, with over 600 data scientists, uses Big Data algorithms for search and ad placement – and even describes its self-driving car as a Big Data project. eBay has a Data Hub to better understand online and offline commerce. Amazon keeps data around for years because its value may be realised only later. “We never throw away data,” says Jeff Bezos.
LinkedIn has leveraged Big Data to create new data products and features such as People You May Know (which increased clickthroughs by 30%), Groups You May Like, and Jobs You May Be Interested In. The company’s seven thousand employees include over a hundred data scientists.
SAP generates more money from business intelligence and analytics than transactional software. IBM has spent close to $20 billion on analytics-related acquisitions. Intuit uses Big Data approaches to improve its website and build consumer loyalty.
Novartis leverages Big Data to develop new drugs. Wells Fargo uses Big Data to understand aspects of customer relationships that they could not previously unearth. Bank of America mines Big Data to come up with cash-back offers to select credit card customers; CitiBank’s targeted mobile offers to customers are a result of Big Data services.
GE has invested $2 billion in analysing data from its turbines (‘things that spin’) to improve their efficiency; it has also rolled out ‘Industrial Internet’ products based on Big Data such as Predicity and Datalandia. Schneider uses Big Data from on-board sensors in trucks to better schedule fueling stops and to promote safe driving habits.
Zynga’s data scientists target games and game-related products to its customers. The NetFlix Prize is awarded to data scientists who can optimise movie recommendations. GroupM uses Big Data to reduce its global data services costs. Macy’s uses Big Data to dynamically change prices in its stores within just an hour (down from 27 hours).
Kaplan uses data science to give advice on test preparation strategies. Amadeus has improved the ‘look to book ratio’ of airline ticket queries via Big Data. Casino Caesar uses analytics in customer loyalty; it can give meal coupons to loyal customers who are having a bad day at slot machines, and is experimenting with mobile devices to deliver better services.
Heathrow Airport, with support from BPM vendor Pegasystems, leveraged Big Data to improve the percentage of on-time departures from 60 to 85 per cent over a period of two months. Its next moves include integrating with the systems of other airports so it can get more accurate information on aircraft delays.
The United Nations’ Global Pulse Innovation Lab has started the HunchWorks project to leverage Big Data around forming hypotheses around weather predictions and global warming. Military applications include anticipating terrorist attacks, mining news media and social media for signs of public unrest, and analysis of data from drone aircraft and distributed sensors.
New startups such as Narrative Sciences offer services to build stories from data points. United Health Group spun off a business called Ingenix to sell data, insights and software. Startup Anvita Health was acquired by Humana to offer broader analytics and Big Data capabilities. Neustar started off by offering data management services but migrated into offering telecom insights and analytics.
A whole industry has been built around social sentiment analysis and brand monitoring around Big Data from social media. Customer journeys and user persona are modelling and predictive techniques that effectively leverage Big Data.
To succeed in the Big Data field, the author offers an extension of his earlier framework and readiness assessment tool called DELTA (data, enterprise, leadership, targets, technology, analysts). Another variation is the set of parameters called FORCE (fact-based decision-making, organisation of analysts, review of business assumptions, culture of test & learn, and embedded analytics).
Some companies have positions such as VP of Big Data, Social Design and Marketing (Intuit), Chief Science Officer (AIG), VP of Analytics, Insight and Loyalty (Charles Schwab), Chief Analytics Officer (University of Pittsburgh Medical Centre) and Chief Digital Officer (McGraw-Hill).
The author defines a successful Big Data culture as one with a sense of urgency, a focus on innovation and exploration, belief in disruptive technologies, and a culture commitment right from the top. “Innovation is the highest and best use of Big Data,” he says.
Looking to the future, the author identifies the emergence of Analytics 3.0 as a significant trend; it is based on prescriptive analytics, and goes beyond the first two versions of analytics (based on descriptive and predictive analytics).
In sum, Big Data and analytics help smart companies lead in the market rather than just react. Companies can start off with the data and see what insights it reveals, or start off with customer needs and see how to respond via data solutions, or both. They can be aggressive in their Big Data strategy (to be ahead of disruption), moderately aggressive (expanding on existing analytics) or conservative (rich data is not yet readily available).
The author demystifies a number of terms in the field: high-performance analytics, digital smoke signals, analytical blanket, and social analytics. He also raises concerns about transparency of data gathered from customers, and some of the legal and ethical issues that arise.
“It’s not how much data you have but what you do with it that counts,” the author concludes.
Author profile
Thomas H. Davenport is Professor of Information Technology and Management at Babson College, a fellow of the MIT Center for Digital Business, Cofounder and Director of Research at the International Institute for Analytics, and a senior adviser to Deloitte Analytics. He has led research centers at Accenture, McKinsey and Company, Ernst & Young, and CSC Index, and has taught at Harvard Business School, Dartmouth's Tuck School, the University of Texas, and the University of Chicago. He also blogs for Harvard Business Online and can be followed on Twitter at @TDav.