From patterns to profits: how Big Data is unleashing value for startups and industry leaders
Driven by startups and some savvy industry leaders, Big Data and analytics are becoming more widely accepted, and the activity of numerous practitioners and investors is showing the way to successful models.
The power of being able to gather, identify, understand and execute upon patterns of data is critical for long-term success of companies as well as for advancement of humanity, according to authors Rob Thomas and Patrick McSharry in their new book, ‘Big Data Revolution: what farmers, doctors and insurance agents teach us about discovering Big Data patterns.’
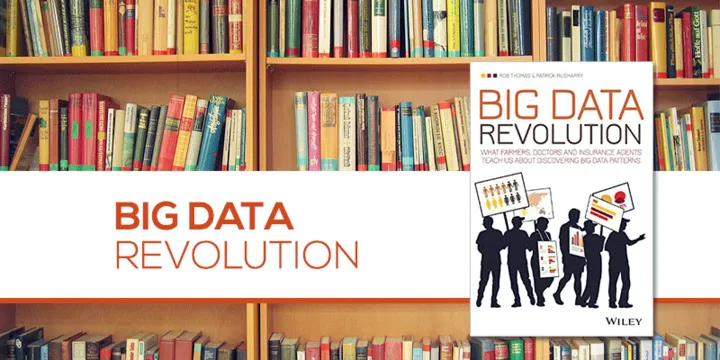
Rob Thomas is Vice President of Product Development for Big Data and Information Management at IBM. Patrick McSharry is a Senior Research Fellow at the Smith School of Enterprise and the Environment, Oxford University.
The 272-page book covers Big Data at work in vertical sectors like farming, health, insurance, retail, energy, manufacturing and government services. Each chapter covers broad historical trends, and the book ends with ethical and strategy recommendations for business leaders (some of these topics are better explained in other books).
For better insights on how to gather and use data, see my reviews of the books ‘The Code Halos’ and ‘Connected by Design,’ as well as ‘Big Data Marketing’ (for internal culture change) and ‘BigData @ Work’ (for corporate and startup strategies).
Here are my key takeaways from the case stories in the book; for more details and a range of podcasts, see the online companion of the book.
Farming has evolved through phases like subsistence farming (1700s), large for-profit farms (barns: 1800s), animal-powered farming (horses: early 1900s) and machine farming (late 1900s). We are now entering the era of data-powered farming, via large scale open data sets on weather and yields, IoT, drones and smart tractors. In future, digital tools will be as important as traditional farming tools in the agricultural sector.
For example, GroundCover uses farm imagery to map and predict potato yields; its CanopyCheck app adds geo-location information for farmers using smartphones. Monsanto’s Integrated Farming System (IFS) uses science-based analytics tools like FieldScript for planting advice, precision seeding and genetic gain; it bought the Climate Corporation in 2013 for $930 million. GrowSafe Systems uses sensors and analytics to track the movement and health of cattle and help farmers deal with disease detection and prevention.
The medical field is still strongly dominated by opinion in diagnosis – which is why it is common for patients to get a ‘second opinion’ before going in for surgery. The first medical schools were established in the 1200s in Italy; the scientific method was applied in medical research from the 1900s onwards. Investors like Vinod Khosla are now promoting Big Data approaches in the medical field, and a number of analysts are urging inclusion of more statistical and Big Data skills in medical education.
CellScope has developed a smartphone-enabled otoscope for patients to examine ears on their own and share the data with doctors. TrueColours lets users enter and track their health data and monitor their progress online, and also aggregates data across cohorts. Quantus tracks real-time cardio-vascular data to get better insights into fighting heart disease. HealthTap harnesses the power of the crowd by letting them ask experts questions, and track patterns in consumer health trends.
Insurance practices began with the Babylonians in 1700 BC (marine insurance), followed by the rise of insurance businesses (1600s), life and crop insurance (1700s), and car insurance (1900s). Today, insurance is increasingly powered by better data, quicker workflow and more informed decision making. Online channels and IoT sensors are helping companies like AllState and Progressive Insurance map, predict and manage risk for automobile policies in real-time. Governments and industry are getting together to share large data sets on natural catastrophes, such as the Global Earthquake Model and Oasis Loss Modeling Framework.
Retail has evolved through phases like local corner stores (1900s), department stores (1920s-1940s), malls (1940-1970), superstores (Walmart: 1970-1990) and e-commerce (1990s onwards). Shoppers feel that the sense of intimacy of smaller stores is being lost with scaling – but Big Data can bring back some of that personalisation and customisation. StitchFix, Keaton Row and Zara are using nimble business processes and scalable curation informed by real-time data to better serve their customers.
Energy firms like GE are riding the three waves of innovation: industrial revolution, Internet revolution and Industrial Internet. Machine data will be critical in the renewable energy sector, eg. for monitoring massive wind turbine installations which are often in remote areas. Aeryon Labs uses drones to inspect and manage energy sites like turbines and rigs. Tesla doesn’t just make electric cars: its Vehicle Management System gathers insightful data which can be used to mine patterns across all Tesla cars and fleets on the road in real-time.
The largest power outage in history occurred during the summer of 2012 in northern India – but the application of data and pattern analysis could have foreseen the risk and prevented the blackout. Data networks are as important as data sources, and will re-shape entire industries. Global utility company expenditure on Big Data analytics could reach $3.8 billion in 2020, according to GTM Research. Smart meters and smart grids could also help ‘nudge’ consumers towards better energy utilisation.
Governments can now use Big Data to come up with better evidence-based policy making, and emerging economies can leapfrog straight into mobile-driven data gathering networks for market and sentiment analysis. The open data movement, open access to content and hackathons are useful ways for governments to engage with society and industry – but concerns over security and privacy will have to be managed as well.
Examples include the Willis Research Network (risk modeling), and catastrophe insurance networks formed in the aftermath of earthquakes in Turkey and Florida. New financial tools like social impact bonds and development impact bonds are showing the way for the Childrens’ Investment Fund Foundation in India. Other firms profiled in the book are ZenDesk (providing customer service across interactive media channels) and SkyCatch (commerialising use of drones in sectors like construction).
The book also stresses the importance for companies to systematically sense data, apply feature extraction and post-processing, and ensure quality and security of data. Data does not just improve existing products, but creates new competitive advantage and even leads to the rise of data itself as a product. The chapter on data factors illustrates the wide range of data pattern advantages: building new applications, reducing subjective distortions, defining new channels, democratising data access and usage, and enabling real-time response options.
For example, Intuit has turned data gathered from its products (TurboTax, QuickBooks, Quicken) into a valuable competitive advantage that even the mighty Microsoft could not beat. Dun & Bradstreet is a good example of a company which leverages data as product; it has spun off other successful companies like Nielsen, Cognizant, Moody’s and IMS Health.
Major culture change will be needed, however, for organisations to accept the power of data-driven decisions, improve internal skillsets, speed up innovation, harness the power of algorithms and machine learning, design new processes and architectures, and especially create new business models.
“Data is much more than an opportunity; it is an imperative,” the authors conclude.