Can we teach people the way we teach machines?
We have been sitting pretty with the knowledge that machines are only able to do the routine, predictable, and rule-based work. That is what an algorithm is essentially, right? And we are seeing the effects of that. The Economist tells us that in 1996, 25 percent of the US workforce was employed in routine office jobs. In 2015, that percentage had dropped to 21 percent. Why are machines closing the lead with humans?
Learn like Google
We learn new ways of behaving when we reflect on the experiences we have. That means listening to feedback from a variety of sources; gathering data and listening to our critics. Once we gather data, we need quiet time to be able to process the many strands of insight and decide to make changes in our behaviour. We then need to make changes in real time the way Google does.
Each year, Google changes its search algorithm around 500–600 times. That means making almost two amendments a day. This includes minor tweaks to its algorithm. Several minor tweaks when combined make the case for a major rethink of the algorithm. Each time we perform a search, we contribute to .000000003 percent of improvement in the search algorithm. With more than three billion searches per day, Google is continuously improving its algorithm in real time.
Humans resist change – machines don’t
Last year Danny Lange, the Head of Machine Learning at Uber, made an insightful observation. He describes what it means to live in a world of uncertainty and probability. He said,
“Basically, we’re now using experience, using data, to have learning algorithms build and use these models and get results that are really predictions with probabilities, rather than having finite deterministic programmes. And as the world changes the data changes and we rebuild the models. This allows us to continuously have a software system that is more in line with the real world.”
Machines don’t get tired. They can parse through mountains of data and keep refining their understanding. Affectiva is using computer vision and deep learning to gather data and building the world’s largest emotion data repository. This massive database of human emotions is being used to teach machines how to perceive human emotions by recognising their expressions.
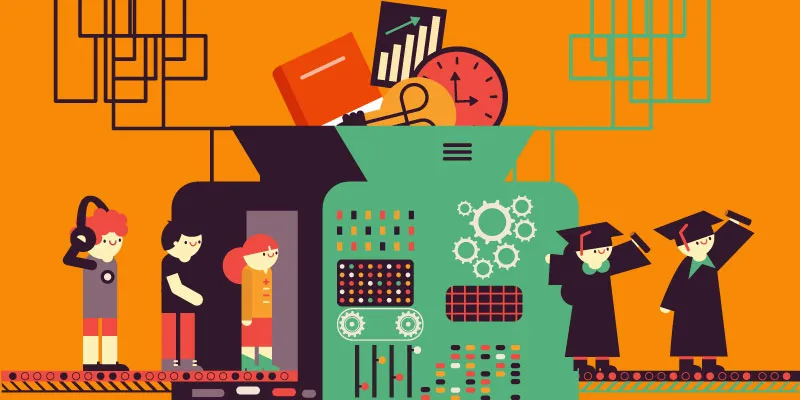
The result is that the machine is able to differentiate between cultural nuances of human emotions. It knows how a Japanese smile and a Brazilian smile are different. It is learning how women express emotions in ways that are distinct from emotional expressions of males.
Softer emotions need more data points
Affectiva has gathered more than a billion data points to learn how human emotions differ based on the device, the content and of course, the individual viewer. These data points are then analysed based on inputs from psychologists and experts. The machine tries to identify emotions in real time. The human experts coach the machine and tweak its algorithm and help it improve.
Imagine trying to learn a new skill or improve one’s levels of emotional intelligence in the same way. What one needs is an enormous database of variants and time to practice and make corrections. This builds awareness of where one is going wrong. Then comes the difficult part of change – being able to change one’s behavior. That means forming new habits and world views.
When organisations send employees to a day-long or even week-long training programme in soft skills, what they need to do is to provide many more examples and provide a coach who can give feedback as the person attempts to change behaviour. The machine is a novice. It is happy to revisit and repeat examples. Humans get bored with repetition. Maybe we need to adopt the mind of a novice when it comes to lifelong learning.
(Disclaimer: The views and opinions expressed in this article are those of the author and do not necessarily reflect the views of YourStory.)