A picture worth a thousand recipes: AI serves up feast of recipes for Thanksgiving and beyond
You’re hosting your first Thanksgiving dinner for your extended family. So the pressure’s on. You need recipes that’ll wow the crowd, ones that live up to the gorgeous images in food magazines.
AI may be able to help.
Just upload a photo of whatever tickles your taste buds, and MIT’s Pic2Recipe tells you what’s in it and how to make it. (And even if you botch the dish, the fact that it was chosen by AI will give your guests something to talk about instead of politics.)
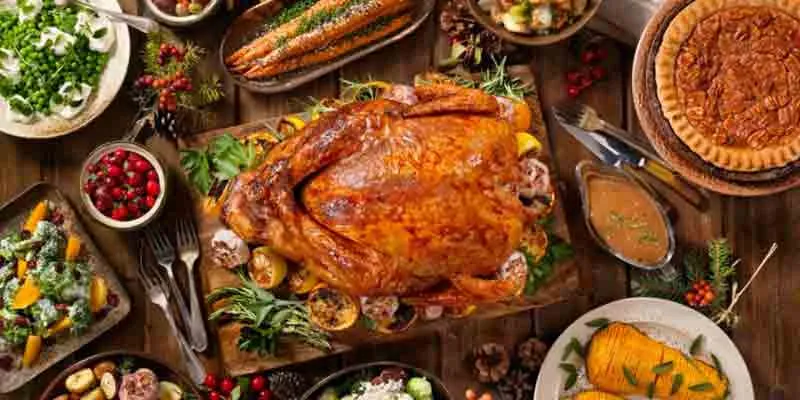
“Whether it’s the best turkey ever or some awesome apple pie, just take a picture and upload it to our demo,” said Nick Hynes, one of the lead authors of a recent paper on Pic2Recipe. Hynes, now pursuing a PhD. at University of California, Berkeley, did the work as part of a team at MIT’s Computer Science and Artificial Intelligence Lab.
The lab’s GPU-accelerated deep learning system isn’t just for turkey day. It contains more than a million recipes — ranging from chocolate chip scones to cheesy oven fries — and more than 800,000 food photos. Researchers say it’s the world’s largest publicly available collection of recipe data, and it’s growing as more people upload images. (The online demo works on a PC or an Android phone, but isn’t yet ready for iPhone.)
AI gets you the recipe
Searching for a dish to spice up my own family feast, I tried out Pic2Recipe on some photos I found online, including the one below of a scrumptious-looking take on sweet potato casserole.
It suggested five recipes, three of which could do the job (Bourbon Sweet Potato Casserole, Pecan Sweet Potato Bake, Sweet Potato Bake) and two puzzlers — Caramel-Coated Spiced Nuts and Streusel Pumpkin.
But Pic2Recipe is about more than recipes. Researchers want to better understand people’s eating habits, with an eye toward fostering healthier diets. That’s possible because the tool detects the ingredients in a dish.
Want to know how many calories another slice of pizza will cost you? Just take a picture. In your own cooking, you might eventually be able to use Pic2Recipe to suggest substitutions that cut calories or boost protein, Hynes said.
Where’s the beef?
Recipes also pose a surprisingly challenging computer vision problem, Hynes said.
While the computer can learn to recognise tomato sauce, it can’t automatically determine if the tomatoes were sliced, diced or chopped. It can’t “see” hidden ingredients like sugar or salt, or whether a lasagna has meat or spinach inside. If the photo shows a cake, Pic2Recipe can infer that it contains sugar. That’s less likely if the cake is sweetened with Stevia instead.
Pic2Recipe performs best with desserts like cookies or muffins because there are so many examples in the dataset. It struggles to determine ingredients in more ambiguous photos.
That probably explains why the photo below of cornbread-sage stuffing yielded two stuffing recipes (but without the corn), plus recipes for creamed vegetables and potato spinach gratin.
The tool also struggles with some language understanding tasks. For example, it doesn’t automatically understand phrases like “combine all ingredients” or “bake until done.”
“You and I know what to do because, as people, we have experience in how the world works,” Hynes said. “But all the deep learning model knows is recipes. It doesn’t know about cooking or flavour.”
Overall Pic2Recipe serves up a correct recipe within the first five results with 55 percent accuracy; within the first 10 results, accuracy climbs to 65 percent. Hynes expects it to improve as more data is added.
How to cook up a recipe finder
To create Pic2Recipe, the researchers built a dataset by scraping more than two dozen popular cooking websites for recipes and photos. They trained their model using NVIDIA GeForce GTX TITAN X GPUs and cuDNN with the PyTorch deep learning framework.
GPUs also helped Hynes put his deep learning model into action, a process known as inference.
“It’s very easy to deploy a model when you don’t have to re-package it to run on the CPU,” he said. “The GPUs makes the demo very responsive.”
Planning dinner with AI
In the future, the team hopes to improve the system so it understands food in more detail. That could include how a dish is prepared (for example, stewed or braised) or several variations of the same food — spaghetti sauce with basil, for example, versus spaghetti sauce with mushrooms and onions.
The researchers may also develop the system into a “dinner aide” that could figure out what to cook given a dietary preference and a list of items in the fridge.
“We want to get new insights into how diet affects people’s health,” Hynes said.
(Disclaimer: The views and opinions expressed in this article are those of the author and do not necessarily reflect the views of YourStory.)