ROAR to help decode Facebook emoticons
A novel computer model called robust label ranking, or ROAR, that helps mine social emotions, could be used to predict various emotional reactions such as love, haha, and angry on Facebook posts, researchers say.
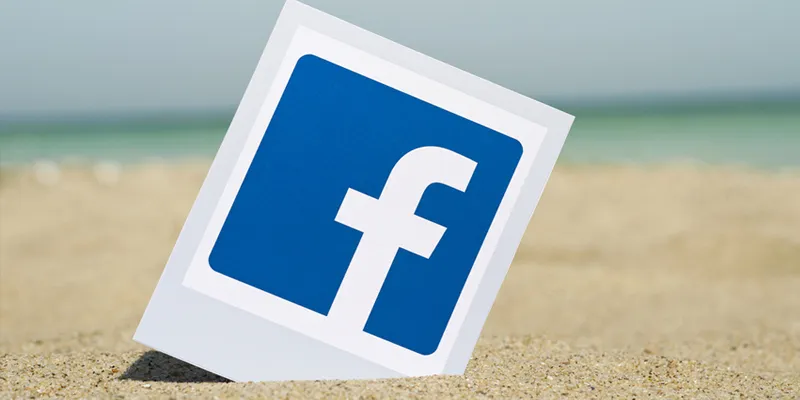
While the trusty "like" button is still the most popular way to signal approval for Facebook posts, ROAR can help navigate the increasingly complicated way people are expressing how they feel on social media.
It could also lead to better analytic packages for social media analysts and researchers.
"We want to understand the user's reactions behind these clicks on the emoticons by modelling the problem as the ranking problem — given a Facebook post, can an algorithm predict the right ordering among six emoticons in terms of votes?" said Jason Zhang, a research assistant at the Pennsylvania State University.
"This is a step in the direction of creating a model that could tell, for instance, that a Facebook posting made in 2015 with a million likes, in fact, consists only 80 percent likes and 20 percent angry," Lee said.
In early 2017, Facebook added five more buttons — love, haha, wow, sad, and angry — in addition to its the like button — the official emoticon reaction.
But, merely counting clicks fail to acknowledge that some emoticons are less likely to be clicked than others. For example, users tend to click the like button the most because it signals a positive interaction and it is also the default emoticon on Facebook.
For social media managers and advertisers, who spend billions buying Facebook advertisements each year, this imbalance may skew their analysis on how their content is actually performing on Facebook, said Dongwon Lee, Associate Professor at the varsity.
The new model was trained using four Facebook post data sets including public posts from ordinary users, The New York Times, The Wall Street Journal, and The Washington Post.
The findings, presented at the 32nd AAAI Conference on Artificial Intelligence today in New Orleans, showed that the new model significantly outperformed existing solutions in precisely understanding social emotions.