How predicting manufacturing downtime using machine learning can ensure business success
As manufacturing becomes more machine and AI-reliant, moving from trying to prevent machine downtime to predicting it before it happens will become ever more important in the future.
The Make-in-India initiative aims at increasing the contribution of the manufacturing sector to India’s Gross Domestic Product (GDP) from the current levels of about 16 percent to 25 percent by 2022. As manufacturing looks to play a larger role in the future of our economy, technology advancement and intervention in this sector will continue to be a great opportunity for the entire IT industry.
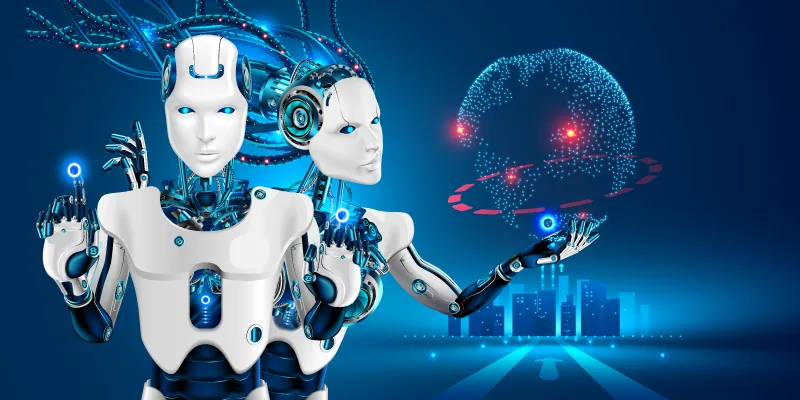
Output by the machine
One of the major concerns and challenges of having a seamless manufacturing output is to prevent and avoid unfavourable machine performance. With the assumption that machines will degrade over time, manufacturing companies, prior to advanced technology intervention, aimed at focusing on preventive and reactive maintenance of the health of their machines, but the use of deep learning technology is leading towards a new-age term method to safeguard the health of machines, coined in the industry as ‘predictive maintenance’.
Benefits of predictive maintenance technology
Predictive maintenance technology approaches can help the manufacturing sector find the optimal inflection point between costs and machine failures. But predictive maintenance is not as simple as a plug-n-play solution, as the requirement of machine learning requires layers of historic data to be collected over time. The true impact of this strategy will only be seen in the mid-term, and like good wine, this technology intervention will only get better and better with time. The data collected over time will help proactively perform machine maintenance to reach optimum efficiency levels of production.
Consider the life-cycle of a (Computer Numerical Control) CNC machine. Today, most CNC manufacturers define the maintenance cycles based on the type of work the CNC machine does for their customer. It is based on their individual experience and judgement. However, if we were to get not just real-time data on the display but also store and analyze the historical data and use of the CNC machine, deep learning algorithms could find out the pattern of use and predict the maintenance and life of the CNC machine.
False positives would occur, i.e. a situation where the algorithm may predict the maintenance incorrectly based on the parameters it has to play with. With some human intervention, this pattern is corrected, learnt, and applied on the following data set to improve the result. So, the algorithm can learn from its mistake and give more relevant and accurate results over time.
Efficient usage and maintenance
Using cloud-based scalable technologies, we could reduce the infrastructure requirements at each premise and even customize the maintenance cycle for each CNC machine based on the customers’ usage patterns. This will not only reduce the cost of maintenance but also improve the efficiency – a win-win for both the CNC machine manufacturer and their customer!
Deep Neural Networks are used in this approach to learn from sequences from data. Unscheduled machine downtime can be damaging for any business. Preemptive identification of these issues can help enhance quality of production and significantly improve supply chain processes. The advantages of using predictive maintenance strategies can enhance overall operational efficiency.
Eco-system of machine learning
Predictive Maintenance strategy is built on the fundamental methodology of Internet of Things (IoT). IoT will not be functional without data and machine learning. This approach is not only about gathering data, but also creating an eco-system to predict and make decisions as a response to the sequences of data collected. Predictive maintenance will be a larger opportunity as global economies progress, and IT solutions providers need to look at this opportunity to further innovate to help manufacturing companies disrupt their industries.
(Disclaimer: The views and opinions expressed in this article are those of the author and do not necessarily reflect the views of YourStory.)