[Tech 30] Uncanny Vision brings sight and vision to ‘blind’ surveillance cameras
Bengaluru-based Uncanny Vision develops optimised computer vision solutions for the embedded market and uses artificial intelligence to make CCTV and surveillance cameras more intelligent.
Surveillance cameras in important buildings and junctions are a given these days, but can they be used to prevent crime? Uncanny Vision wants to make sure they can.
“We call ourselves AI for surveillance," says Ranjith Parakkal, the Founder and CEO of Uncanny Vision, a Tech30 company.
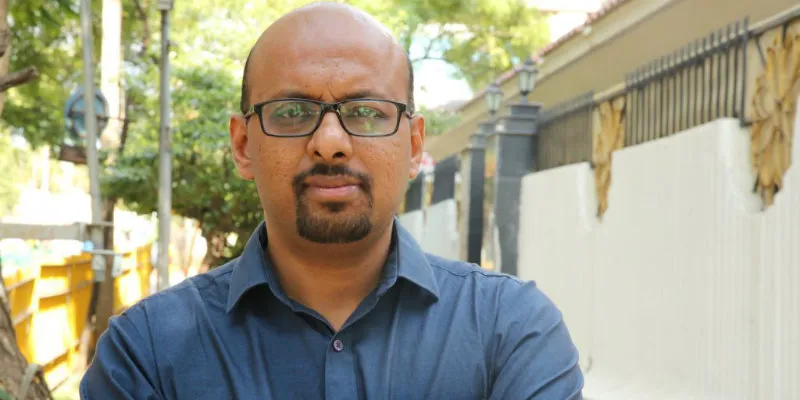
In a conversation at the YourStory office, Ranjith doesn’t come across unlike the regular early stage startup founder. For one, he isn’t a fresh college graduate; he’s a seasoned professional who turned entrepreneur to follow his passion.
Ranjith spent 11 years at Texas Instruments as the global technical architect, and was keen to build something with image processing. He even filed a patent in an image processing product he built.
“I was keen and focused on accelerating computer vision. We had computer vision libraries made faster for employee process. Customers could build their applications on top of it - these could be surveillance or even automotive progress - and make it faster for embedded processes. We realised we weren’t getting anywhere because the scope was too vast,” Ranjith says.
It was in 2013 that he started working on this idea.
Bringing in AI
Ranjith soon realised that developing and deploying computer vision technology was computationally intensive, and required high-end PCs and servers, and that chips were advancing at a rapid pace. He saw that there was an opportunity to leverage new-age hardware and AI to bring real-time “vision” to IoT devices.
In 2013-14, the team realised that AI and deep learning could solve problems much more accurately. Soon after, Ranjith says they decided to apply deep learning and AI to surveillance.
The product now acts as an analytics server that sits behind the camera, picks data, and generates live alerts to the cloud. Starting with ATMs and banks, the team has now moved to automotive and number plate recognition.
“The problem is that there are several million surveillance cameras. But all of them are ‘blind’; they record data and are good for post-mortem analysis. How do you fix something real time and convert your surveillance camera into a vision sensor? That is what we were working on,” Ranjith explains.
What does it do today?
Today, four years later, Uncanny Vision has moved from providing an embedded solution to working on an end-to-end solution, where they even provide the hardware.
When the founders started Uncanny Vision, they were purely into software play, but quickly realised that there were several other missing pieces – such as the right camera and the right processor at the right price point.
“If you just go with the software, making a sale is hard. This is a hardcore intelligent device. It was then that we decided to invest in working on the complete hardware and built our own custom camera, and custom embedded package."
Uncanny Vision’s surveillance system comprises a few key technologies like deep learning-based convoluted neural network (CNN) and recurrent neural network (RNN) models for scene recognition, anomaly detection, face recognition, and human pose detection. UncannyCV and UncannyDL for optimised computer vision, and a network backend connected to Amazon Web Services’ IoT platform for real-time alerts.
The core product
“Our number one product today is the number plate recognition software. The first information you want to know about a vehicle is the number plate. It can be found anywhere, but number plate recognition accuracy has been low in India – about 60-75 percent accurate. This is because Indian number plates aren’t standardised; you have different fonts, materials, and reflectivity. Nobody was solving for this to be used as a real application,” Ranjith explains.
The Uncanny Vision and software component works towards a 90 percent accuracy rate. Initially, the team had banks as their clients and now it is working with other enterprises and traffic controls as well.
With a 51-member team that has a strong understanding of embedded design, computer vision, and machine learning, Uncanny Vision is now in the process of developing a next-generation, intelligent surveillance camera.
Model, market, and future
Uncanny Vision’s business model works like a hybrid SaaS model. For the number plate recognition, the team charges per lane, where the price of the hardware is sold at a one-time flat fee a little north of Rs 50,000.
The revenues, however, are primarily generated by the SaaS model, which includes software licences that Uncanny Vision provides, and a monthly fee for different tools (up to $100).
“For example, we are working to ensure that cars move smoothly and quickly through toll booths at highways. This helps reduce pilferage, vehicles move faster. We are seeing if we can work with wallets on a pay-by-number plates model,” Ranjith says.
Uncanny Vision recently raised an undisclosed round of funding.
The AI-powered surveillance sector has seen a lot of interest globally. Google’s Nest has outdoor and indoor cameras that leverage AI to detect people and security threats. San Francisco-based Umbo CV, which announced a $2.8 million seed round in March 2016, is developing a cloud-first, AI-powered self-learning camera.
Uncanny Vision, on the other hand, is focusing on a niche sector and detecting anomalies.
“We are going to look at different enterprise-use cases and add AI to computer vision. We are looking at city and traffic-related use-cases,” Ranjith says.