5 key challenges with developing and managing AI-powered products
Legendary American football player Vince Lomabrdi once said, “It takes months to find a customer, and seconds to lose one.” It’s a story we are all too familiar with. You spend what seems like hours on the phone trying to explain a problem to a customer service representative or even weeks over email only to come back completely dissatisfied with their response.
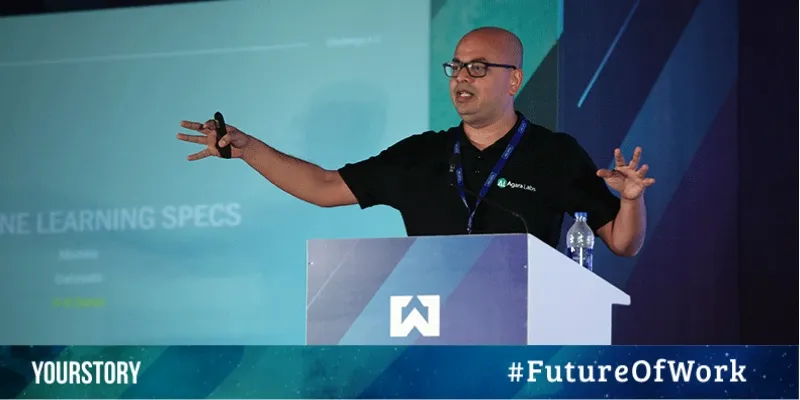
Abhimanyu Singh, Agara Labs
“Our vision is to take this ineffective solution and try and apply AI to it and make it as efficient as possible,” said Abhimanyu Singh, Co-Founder of Agara Labs at the second edition of YourStory’s flagship Future of Work event in Bengaluru on February 9. Addressing the packed hall on the topic of Developing and Managing AI-powered products, he said that when it comes to products and product development, AI changes everything. “These are not just buzzwords but clear, ground-level tools.”
Setting the context for his talk, Abhimanyu said it was it was important to remember that AI tools are non-deterministic and temporal. “Typically, when you build a software for a product, it takes you from A to B and that is what it does day in and day out. But when it comes to the real world, when you give A to a product, depending on the way you are handling it today or the day after, you could get a B or a C or a D. And that changes the way we think about what a product is supposed to do, the way we are supposed to test them, evaluate them etc.,” he said.
“Products can get better or worse over time. The reality is that once we give input A, depending on the time that we are giving the input, things change. Once again, it’s impossible to figure out whether something is getting better or worse over time. The example we are giving here is what we call develop and deploy. We have been running this for over a year and these are some of the insights to come out of it,” he said.
In the current scenario, a customer sends in an email with a support query. The email reaches the company’s collect desk. “An agent will come in on a Monday morning, read through it and understand what the customer wants and will respond.
He or she will take time to write a nicely worded response and that is when you get a resolution to your problem. There’s stuff that goes on in the middle and that is the crux of it. At Agara Labs we come in the middle and we start evaluating that email immediately. What we are trying to do is understand all the things the adviser has to do and resolve it before the adviser logs in again. So, when the adviser logs in, he or she does not see just a question, but the question with all the resolutions. This saves a lot of time.” But no system comes without its challenges:
- For any product, the first thing you have to understand is what it is supposed to do. When you have a machine writing an email, what are we optimising for? The answer should be that this should be the best email that can be written in this situation. But what does that mean? Each individual could have a different idea of what’s best. So, how do you build a pipeline when you don’t know what the optimal output is? We sell to multiple customers and the best solution is to present these features in the best manner possible. But what if we don’t know what those features are? Ten people looking at a screen can have 10 different versions of what the best features are. That’s one the biggest challenges product managers face.
- The second is when you go deep into AI and Deep Learning is writing the specs for Machine Learning (ML). A lot of engineers have an intuitive understanding of what a problem needs, but when it comes to ML, it can become challenging. One solution is to have excellent predictions for a small set of subjects or have average predictions for all of your subjects. Product managers have to decide where along this spectrum their solution lies.
- We tend to think of AI as a monolith – a giant brain sitting somewhere and doing magical things. Typically, even in the largest of systems, Deep Learning components are going to be one central path surrounded by jungles of software and it is this software, written in a traditional manner that determines how good or bad your product is, and not just the central part. And this can produce an infinite set of solutions. At the end of the day, the person sitting at the other end of the computer has to do something with these infinite options and that is called re-ranking. This is paired with other inputs to come up with a final answer.
- So, how do we determine how good that final answer is? If you were writing an email, you would write differently based on whom you are writing to. How do we measure success across cases and time? And how do we improve outcome? In the software world, we have AB testing, where we start with two interfaces, give the same input and get the same output. But what if your outputs are different? In ML, the minute you change one bit of your model, the output is different. That’s when comparing becomes a challenge in AB testing.
- Ultimately, AI is nice to have. But one of the biggest challenges is we need to talk about it objectively and honestly. The truth is that AI is not a silver bullet. There is no AI that can solve everything. Even in the best-case scenario, AI does very few things in very small scenarios, some of the time. It’s important to keep a human in the loop to do the rest of the work. We can build systems that do specific tasks incredibly well. Finally, there is end-user sensitisation. Always keep the end-user, i.e., the person who will be working with your product in mind.