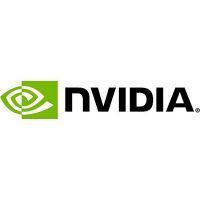
NVIDIA
View Brand PublisherEye on success: AI and business impact through collaborative ecosystems for startups
Corporates and startups are co-innovating through a range of engagement mechanisms such as accelerators, strategic investments, demo days, hackathons, competitions, and meetups. Recent roundtables with a wide range of AI-focused startups in Pune and New Delhi have surfaced a number of commonalities, concerns and convergence of interests between entrepreneurs and NVIDIA.
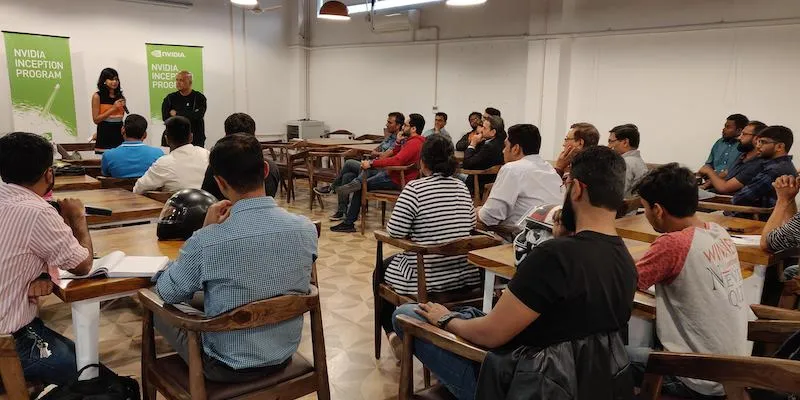
The discussions centred around the different flavours of AI, use case scenarios in India, technology and business challenges, metrics for success, and the role of accelerators for support and scale. The size of the Indian market, rapid penetration of mobile, and the explosion of multi-media content and data have created the ‘perfect storm’ for the application of AI to solve population-sized problems.
However, the path to success requires clarity on AI technologies, integration capabilities, and talent to demonstrate value at scale. Here are five key clusters of takeaways from the wide-ranging discussions from the YourStory-NVIDIA meetups in Pune and New Delhi.
1. Flavours of AI
AI refers to a broad range of tech families, and this can cause some confusion since many different companies claim to be doing AI. Clarity is needed in defining which AI technologies are in play, and in which business context, eg. automation, data insights, learning.
For example, supervised learning can be for fraud prediction, reinforced learning in gaming, deep learning in image recognition, RPA in digital tasks, and chatbots in customer conversations. Some AI applications are visible to customers in B2C settings, others are embedded in core enterprise applications or B2B contexts.
2. Use case scenarios
The Indian B2C and B2B sectors reveal a number of scenarios for application of AI. For example, data uploaded by thousands of retailers need to be harmonised and processed at scale and in real-time, so that analytics can be applied to generate business insights.
While AI technology applications may be straightforward in some cases, the challenge can be in finding the right business model, and offering services in a layered manner so that some can be free while others are fee-based.
3. Metrics
Many startups are grappling with a clear set of metrics for showing AI impact, demonstrating value to clients, and finding a sustainable business model. Metrics need to be differentiated based on outputs and outcomes. These can include accuracy, precision, or performance measures such as F1 score.
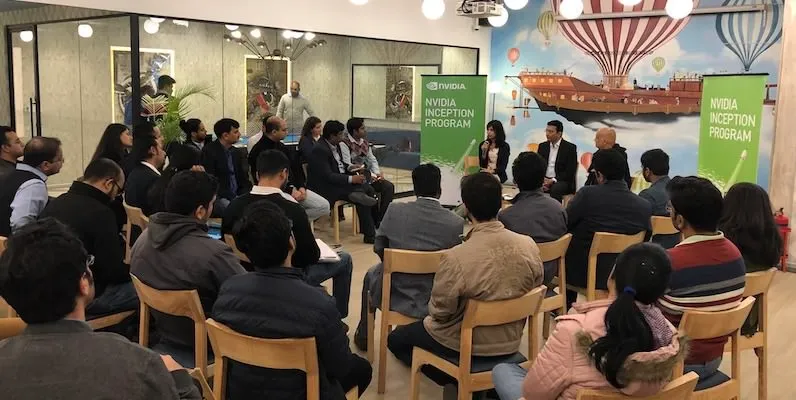
For example, volumes of data processed or speed of extraction are a good start, but these need to be connected to range of insights and their quality. These in turn need to be connected to tangible business metrics like productivity gain, new business acquired, or reduction of risk.
4. Challenges
Data challenges faces by AI startups are of three types: not enough data, or low-quality data, or inability to categories them and find relevant patterns. There is also confusion in the market in terms of where traditional analytics ends and current AI begins.
Availability of talent and continuous training in fast-moving technology frameworks are another issue. On the regulatory front, there are concerns about data storage, extraction and ownership, particularly involving cross-border transactions and interactions.
Other challenges arise in converging technologies, when AI is combined with other emerging technologies in trading, mixed media, and e-learning, for example. While AI applied to Indian language content is a promising application, getting multi-lingual talent can be an issue.
Finally, there are cost challenges faced by startups in terms of affordable access to computing power for AI applications, eg. in sectors like video processing. Many large companies are still unaware of the need for GPU as compared to CPU.
5. NVIDIA Inception
In this regard, NVIDIA’s Inception accelerator programme offers a range of benefits to AI-focused startups, ranging from technology training and event access to sales collaboration and investment opportunities. Specific features of the accelerator include discounted access to GPUs and access to global community of developers.
NVIDIA’s Deep Learning Institute (DLI) offer monthly webinars with industry experts in this regard. There are already over 200 Inception members in India, with more to be onboarded this year. Some members include Julia Computing, SigTuple, Hike Messenger, Niramai, Maxerience, Niki.AI, Tonbo Imaging, Qure.AI, Netradyne, and others.
NVIDIA’s GPU Ventures has invested in 33 companies already. These include Abeja, Acceleware, Drive.AI, DeepInstinct, Graphistry, JingChi, SoundHound, TempoQuest, Tusimple, and Clarif.AI. Successful exits include MotionDSP, Right Hemisphere, Mental Images, and Rocketick.
1550051735940.jpg?fm=png&auto=format)
Other upcoming initiatives that will benefit startups include office hours, hackathons, and curated meetups. Growth-stage startups also stand to gain by GTM support from NVIDIA’s teams, and by insights from NVIDIA research to plan for the next wave of emerging technologies.