Flipkart CTO Ravi Garikapati shares six steps to begin using AI in your startup
Ravi Garikapati, CTO and head of Fintech at Flipkart, talks about how to create an enterprise-wide AI journey.
Ravi Garikapati is famous as the Chief Technology Officer at Flipkart; he is also the Head of Engineering and its Fintech team. So it’s not everyday that you get to hear him talk at length on the tech that rules the world today: artificial intelligence (AI).
At the second edition of YourStory Future of Work last Saturday, Ravi spoke to an audience of techies and entrepreneurs. The master of cloud platforms, big data processing, machine learning, and AI indulging them in the multiple facets of AI for a tech-driven enterprise.
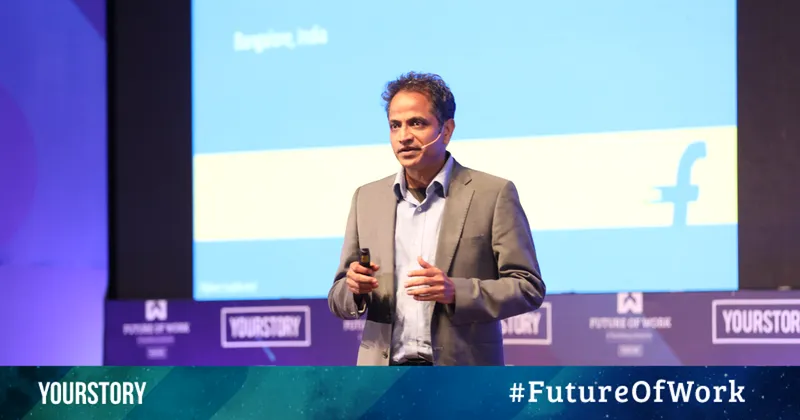
Ravi Garikapati, CTO and Head of Fintech at Flipkart
According to Ravi, an investor's first question to a startup often centres around its AI strategy.
“AI is the new electricity; it will change all industries. It will make a new economy worth tens of billions by 2030 or so," he said.
As such, he advised startups to think about AI strategy holistically. "In my initial days, with any big problem statement I wanted data scientists and scholars. But you need more than one discipline to pull off an AI success story," he shared.
Ravi believes in six steps to make a sustainable AI vision in the company: "It starts with your hardware computing infrastructure, storage infrastructure, and the kind of network you have in place."
AI and machine learning (ML) gives great solutions across functions at any enterprise. But choosing a project to test your AI power has to be careful, Ravi warns: “Make sure that it is not something that gets out of hand quickly. And ask the right questions: Does it give a quick win? It is too trivial or unwieldy in size? Is it specific to your core domain? Is your project creating value?”
AI-first culture
Following Flipkart’s AI-first culture, Ravi strongly endorses adoption of AI/ML for problem-solving among all teams (including business and operations).
"AI/ML skills is not just confined to just one function. Not everyone needs to be thorough; but having some understanding of AI/ML and the kind of advantage it can bring to a business problem across functions is important. You need to device different training modules to suit different audience in the company," he says.
Additionally, Ravi suggests that all data scientists should read research papers and evaluate them. He also encouraged them to collaborate by presenting top papers from global conferences. He also put forward the idea of setting guidelines on the AI-first way of working, and showcasing good AI work at the organisation you work with.
"Use office space branding to inspire people to use AI. Industry leaders giving regular talks at the organisation will also motivate them. Building an AI-first culture should be proactive, not an after-thought,” he advised.
Data governance
Every tech-based organisation has to ensure that large amounts of high-quality data are available to all its teams at any given point of time. Ravi also advocated improving the quantity of data ingested on Big Data platforms. “Come up with data quality metrics and publish them to drive behaviour. Explore other entities that can be ingested in the data platform. Reduce the time it takes for users to discover and access data,” he advised.
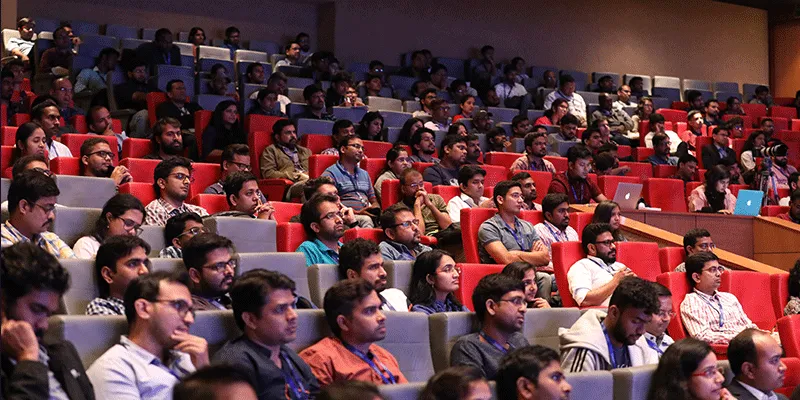
Audience at Future of Work .
Access to research
Ravi also recommends accessing (academic) research from both Indian and international universities for AI. “Building a company is impossible without academic research,” he noted, adding that startups should also seek help from top professors in AI as well as influence top institutions like IITs to focus more on AI.
“Start reading papers applicable to relevant problems. Connect to academia; data we hone will excite them. There is great interest from post doctoral students and professors to collaborate for relevant topics. Find a way to engage them. This will ensure that tech and data science teams in your organisation have access to best-in-class research in AI/ML,” he says.
Ravi also urged startups to evaluate cost and ROI of setting up labs in Indian institutes for AI research.
The hardware question
His experience at Flipkart has given Ravi major insights into the hardware part of the AI question.
“Flipkart had invested in hardware, built our own cloud, invested in computing and storage, etc. But when we wanted to solve some problems, we were handicapped with our hardware infrastructure. Some of our hardware worked well for database applications, but they were underwhelming for solving ML problems. You need to understand your business applications and the kind of computing infrastructure you need - for various amounts of data,” he says.
Ravi believes that the right hardware will enable all current and future AI/ML applications at your organisation. He recommends research on hardware currently available in the market before building your own.
“Arrive at a two-year understanding of your organisation’s hardware needs, and evaluate sensors that can be installed in your operations for more data collection. Then come up with low cost but effective ways to scale data storage in the future,” he notes.
Creating an enterprise with AI essentially requires people with the right skills to apply AI/ML to solve problems for teams like DS, tech, product, business, operations, etc. Ravi proposes regular training programs for various functions in a cost and time efficient manner. He also recommends hiring of all engineers and project managers to include data orientation.
Domain understanding
To ensure that engineers and data scientists have high levels of understanding of their domain, Ravi recommends regular business talks for engineers and others, as well as giving regular visibility to selected business and financial metrics to engineers.
“From my experience, I have learnt that if your data scientist or engineer does not have a deep domain understanding to solve the problem statement, it will disrupt the full usage of AI in the organisation. Outcomes will be very underwhelming and disconnected from what the business needs,” he says.