From capability to control: how a bill of rights can help humans harness AI for good
Wharton professor Kartik Hosanagar, a graduate of CMU and BITS Pilani, traces the growth of AI and its impacts in the new book, ‘A Human’s Guide to Machine Intelligence’. He also calls for wider understanding and cooperation across the board to harness AI for the benefit of all.
The complex interplay between the power and unpredictability of AI is addressed in Kartik Hosanagar’s new book, A Human's Guide to Machine Intelligence: How Algorithms Are Shaping Our Lives and How We Can Stay in Control.
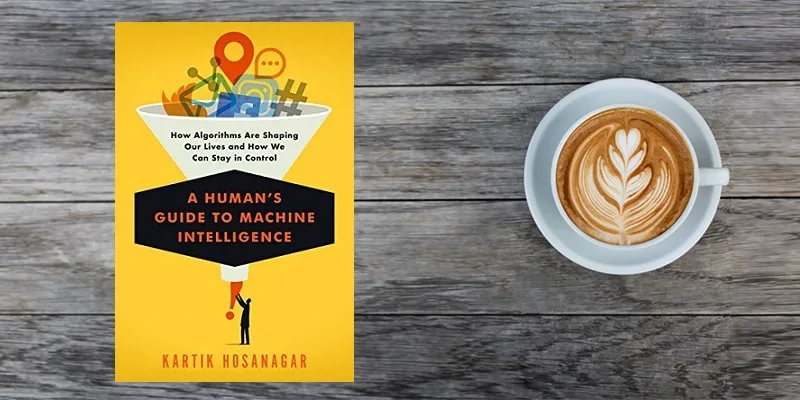
Kartik Hosanagar is a professor of digital business at The Wharton School of the University of Pennsylvania. He also co-founded Yodle and SmartyPal, and is involved with a range of startups as investor or board member. Kartik has consulted for Google, American Express, Citi, and other firms. He graduated from CMU and BITS Pilani.
The 10 chapters are spread across 260 pages, including 14 pages of references and notes. Here are my key takeaways from the book, divided into three parts: Foundations, Impacts, and Control.
See also my reviews of the related books The AI Advantage, Human + Machine, Life 3.0, The Four, The Inevitable, The Industries of the Future, Machine, Platform, Crowd, and What to do When Machines do Everything.
Though AI is an engineering marvel, it also raises important questions of ethics, economics, psychology and even philosophy. Key questions to ask include whether AI will put an end to human biases or accentuate them. AI is seen as the greatest opportunity for human progress – but its unpredictability poses the greatest threat as well, Kartik begins.
Understanding AI today has a sense of urgency due to the scale and speed of large global tech platforms and specialised applications, and varying human perceptions of trust with regard to AI across domains. Automation will pose challenges for job creation, and calls for extensive workforce reskilling. Issues of gender, religion, and caste bias in AI algorithms will need to be framed and addressed.
For example, some philosophers such as Nick Bostrom argue that the unpredictability of AI poses an existential threat to humans. Data scientist and political activist Cathy O’Neil even cautions that modern algorithms can be “weapons of math destruction.”
Kartik proposes that just as humans are shaped by a combination of genes and experience, so also the “nature and nurture” framework can be used to understand and even tame AI. The book concludes with a call for a “bill of rights” to limit AI’s powers and require a degree of transparency, explainability, accountability and control. This can help protect user privacy, safety, and anonymity.
I. Foundations and properties
Four chapters trace the rise of AI and some of its properties. Key figures in the journey of algorithmic practice include Wolfgang von Kempelen (Mechanical Turk, 1783), Charles Babbage (analytical engine), Alan Turing (imitation game), John McCarthy (computer models of thought), Claude Shannon (automata theory), Herb Simon (bounded rationality, Logic Theorist), and Geoffrey Hinton (neural networks).
Though early AI may have been “over-promising and under-delivering,” the tables began to turn with IBM’s Deep Blue defeating Gary Kasparov in chess in 1997. Though this was generally from “brute computing” (200 million moves explored per second in advance), modern AI as in Google’s AlphaZero is seen as more successful: it learnt chess in four hours, and evaluated 80,000 positions per second.
Kartik defines an algorithm as a series of steps one follows to get something done. Early algorithms consisted of predefined steps, whereas modern algorithms can learn completely new sequences of steps. Machine learning is one of AI’s most important aspects; it involves learning from experience and progressively improving performance.
Artificial general intelligence (AGI) or “strong AI” tackles a wide range of tasks, while artificial narrow intelligence (ANI) or “weak AI” addresses narrow, specialised tasks. Early “expert systems” with preset rules were seen as too brittle in novel situations, and attention shifted to ML in the 2000s.
AI and ML are being increasingly used to govern decisions regarding online shopping, movie selection, navigation, music playlists, news, insurance, recruitment, medical diagnostics, security, social media, and even dating.
The rise of the internet and smartphones, availability of big data, and powerful processors are driving AI today, along with techniques like deep learning (layered neural networks for detecting patterns that even human programmers may not notice). Data can also be generated by machines themselves (reinforcement learning), without requiring humans to feed them data (supervised learning).
AI is also being deployed in India by companies such as Myntra (apparel design generation), GreyOrange (robots for warehouse management), HDFC Bank (conversational chatbot), and Belong (resume screening).
1. Structure and automation
Blended approaches (eg Spotify) involve combining the “knowledge and fairness of content-based design” (eg Pandora’s 450 music attributes) and the “simplicity and social appeal of a collaborative filter” (eg Last.fm), Kartik explains, drawing on the music industry as an example.
“Pandora has a deep understanding of music and the vocabulary to articulate it,” he says. On the other hand, automated collaborative filtering as in Last.fm is unable to explain recommendations, and is able to work well only on large and diverse data sets.
Algorithms such as search engines have helped manage the information explosion, and draw on the power of inferences. At the same time, they have spawned gaming phenomena like “Google bombing” to skew results, and also tend to favour popular choices over obscure but important items (“rich get richer”).
Though niche products can be discovered, they lose overall market share and mindshare to blockbuster products under algorithmic recommendations due to popularity bias, Kartik cautions. In many cases, it is also important to have not just accurate recommendations but diverse recommendations.
2. Predictability
While “if-then” statements lead to predictable rules and results, they constitute the strength as well as weakness of deterministic systems in the face of the wider world. According to Polanyi’s Paradox, we know more than we can tell or articulate, eg. how to describe your mother’s face so that others can recognise her.
ML can help uncover knowledge hidden in data, and make cross-industry inter-disciplinary connections, according to Kartik. Systems based on codified and articulated rules are limited, and can be gamed or manipulated. This has led to the field of adversarial ML, or learning from data that may have been modified by adversaries to trip it up.
There is an important tradeoff between predictability and resilience, Kartik explains. Adaptability is important for resilience and longevity, as seen even in political documents like democratic constitutions. Without adaptability, systems will become obsolete. Resilience calls for adaptability, but this may not lead to predictability.
3. Context
Algorithmic results are determined by their logic, data, and human interaction. These three components affect each other in complex ways in system features like personalisation, as seen in the rise of filter bubbles, echo chambers, political polarisation, and social fragmentation, Kartik explains.
Understanding these phenomena require inputs not just from computer science, but social science and psychology as well. Understanding and managing this “complex cocktail” is an increasingly difficult challenge as datasets, interaction patterns, and algorithms continue to evolve.
II. Impacts
One section of the book explains the impact of AI on human perception of free will, and unanticipated consequences of AI. Both these impacts feed into growing demands for greater human control over algorithms.
1. Free will
The notion of free will in human decision-making is challenged by the rise of automated recommendations, which are responsible for an estimated 80 percent of viewing hours streamed on Netflix, 35 percent of Amazon sales, and the vast majority of matches on dating apps like Tinder and OkCupid. Such recommendations also leverage design approaches like notification and gamification, which can unfortunately exploit and amplify human vulnerabilities, Kartik cautions.
“While we might feel as if we are making our own choices, we’re often nudged and even tricked into making them,” Kartik adds. Even multiple-choice options have many possible alternatives excluded; newsfeed algorithms thus shape our worldview and opinions when it comes to reportage.
Studies have shown that emotions can be contagious, as people who see fewer negative posts tend to be more positive in their own posts, and thereby affect moods of hope or despondence. Algorithms thus have a transformative impact on our lives and on social outcomes.
Interestingly, stated preferences and actual user behaviour can vary. Data trails have revealed differences between what users say they want and what they actually view online, eg. when it comes to movies and dating matches. Algorithms based on actual analytics can be more accurate in this regard when it comes to making recommendations for users.
2. Unanticipated consequences
Unfortunately, the rise of AI also opens up the risk of conscious, unconscious, and non-conscious bias creeping into algorithmic decisions. Risks arise from the fact that ML techniques like neural networks learn strategies and behaviours that even their human programmers can’t anticipate, explain or understand.
Lack of clear mental models of how algorithms make autonomous decisions poses challenges for researchers, civil society, and regulators, Kartik cautions. This was seen during the “flash crash” of 2015, when the US Commodity Futures Trading Commission approved a rule giving it access to trading firms’ source algorithms. In the gaming world, even the programmers of AlphaGo did not understand the moves it made to defeat the game Go’s world champion, Lee Sedol.
Racial and gender bias have been reported in the predictive terms appearing in search queries, and in automated tagging of photographs. This is partly due to lack of machine training on diverse and unbiased data pools. Better data therefore may be more important than big data, though this changes with overall size of data pool, Kartik explains.
Unanticipated consequences are generally classified into unforeseen benefits (eg. Viagra), perverse results (deterioration, eg. “cobra effect” in colonial India), and unexpected drawbacks (eg. rise of fake news). Unfortunately, the continued rise of AI can lead to even more side effects; tackling them requires continuous testing, detection, steering, resolution, and refinement.
For example, Microsoft’s conversational chatbot Xiaolce was launched in China in 2014, and attracted over 40 million followers, receiving much warmth and affection. On the other hand, its Tay.ai chatbot launched on Twitter in 2016 for a US audience was shut down within a day after it spewed out hate speech.
III. Taming the code
The last section of the book explains what needs to be done for humans to trust and control an AI-dominated future, and the early steps taken in this regard. This calls for research and activism across the board.
1. Trust
Humans seem to trust algorithms in many situations (eg. movie recommendations, stock trading), but not in some notable ones like driving. Though human error is to blame in an estimated 90 percent of crashes, many people still consider autonomous cars to be less safe than human drivers.
Perhaps the level of expectations for safety and the cost of error are seen as very high, Kartik explains. The level of trust in IT and AI also varies across sectors, and the level of early hype, as seen in fields like medical diagnosis. Some analysts have predicted job loss in fields like radiology (a “medpocalypse”), others see AI aiding and augmenting rather than replacing humans. Either way, AI is set to redefine many fields.
“We cannot take user adoption for granted, especially in light of AI’s public and trust-sapping failures,” Kartik cautions, pointing to accidents in driverless cars as an example. The battle for trust in AI has to be won if doctors are to trust diagnostic systems or if users are to ride in driverless cars.
2. Control
Large tech firms and their users are not always on the same page when it comes to issues of profit, engagement levels, and user satisfaction, Kartik explains. Issues to research include whether giving users some control over the algorithm improves their trust and satisfaction in it.
Level of trust also varies with users and devices; for example, some early users of driverless cars were seen to place too much trust in them, and not enact some safety recommendations. However, “driverless elevators” were hated when first introduced; features like a “stop” button were seen as reassuring by giving users some control.
Product control can be of three types: behavioural (product features), cognitive (product information) and decisional (eg. refunds). In the context of the large tech platforms, users are generally allowed to rate products or report hate speech.
3. Transparency
Transparency has a tangled relationship with trust, Kartik explains. A certain degree of transparency helps trust, but too much transparency may reduce trust by causing suspicion or confusion. For example, studies have shown that “over-explaining” grading systems reduces trust in them.
In the context of ecommerce and retail, transparent explanations of products can vary in terms of features, purpose and tradeoffs. Research is needed to uncover how trust is impacted by competence, motive and track record of the algorithm. This has spawned the field of XAI, or Explainable AI.
Regulators can also play a role here by calling for transparency in algorithms, eg. making source code available to auditors (technical transparency). However, the effectiveness of this measure depends on the competence of the regulator in understanding opaque algorithms.
The algorithmic bill of rights
All the above discussions are woven together in the final chapter calling for an “algorithmic bill of rights” to protect society in the age of AI. The ACM recommends that these rights should be based on guiding principles like awareness (of potential bias and harm), redress (for those negatively affected by algorithms), accountability (of those who use algorithms), explanation (of algorithmic decisions), governance (of data), auditability (of algorithms and data), and validation (regular testing, public availability of results).
Professor Ben Schneiderman has called for an Algorithmic Safety Board. Europe’s General Data Protection Regulation (GDPR) protects sensitive data and calls for transparency of data used by algorithms. The tech industry’s Partnership on AI is meant to address safety, bias, human-machine interaction, and broader policy issues. A role for external regulators and civil society is also needed in this framework, beyond self-regulation.
Kartik urges four pillars of user rights in this regard: description of data collected and used by algorithms, explanation of procedures, feedback/control, and awareness of unanticipated consequences. This framework of rights, responsibilities and regulations calls for involvement by techies, scientists, entrepreneurs, regulators and end users.
Kartik signs off with a compelling question: “How will we conceive, design, manage, use and govern algorithms so that they serve the good of all humankind?”
(Edited by Evelyn Ratnakumar)