David vs. Goliath: Uber, Urban Company and Tinder
In an app-driven world where booking a service or making reservation is not determined simply by matching one’s choices based on the availability of the service, but is dependent on a host of other factors not the least of which is whether the desired service wants to be paired with us.
Akash Chaturvedi
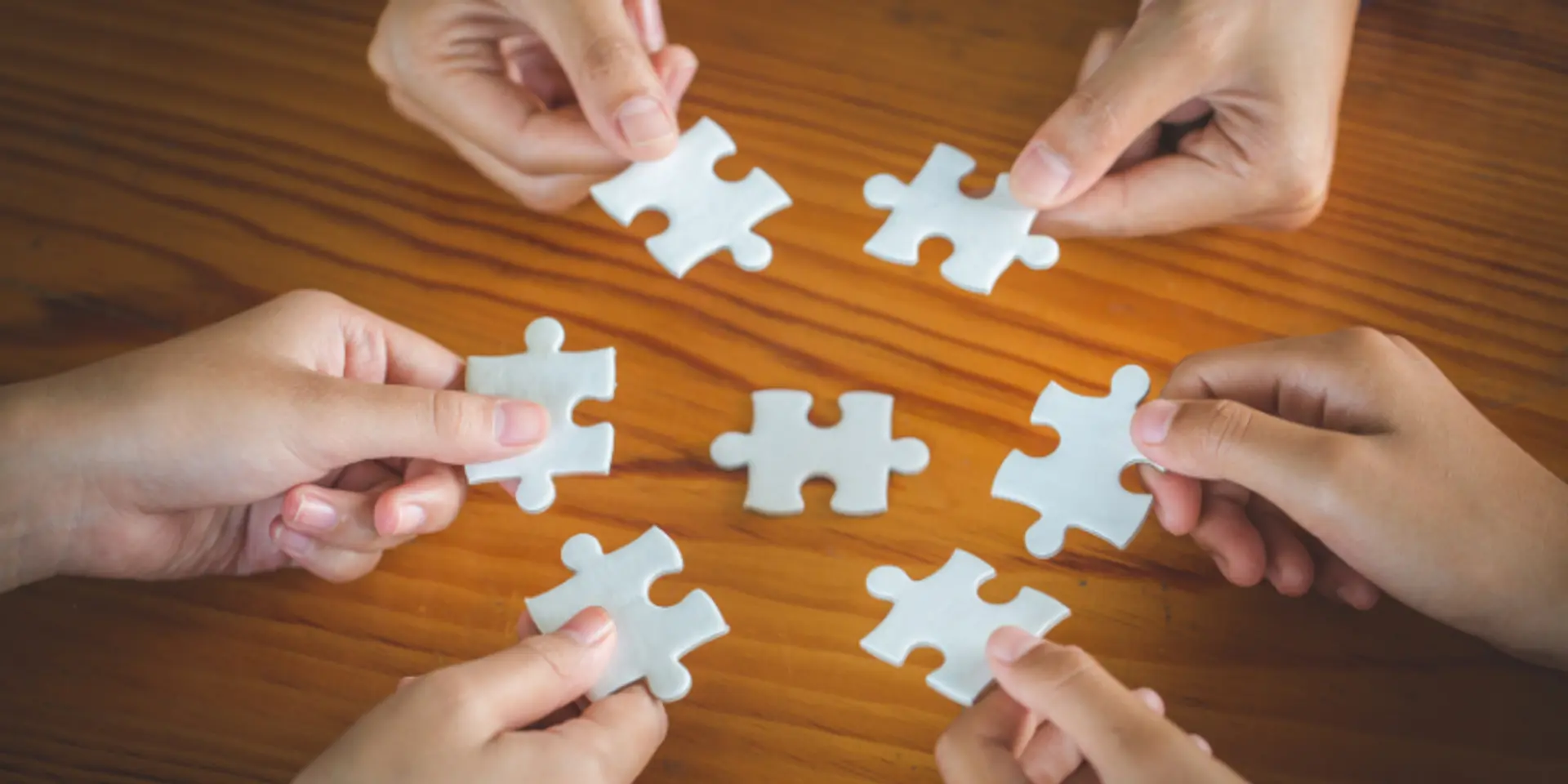
Wednesday September 16, 2020 , 8 min Read
Economics is the efficient allocation of scarce resources, and also about making those resources widely available and therefore, less scarce. According to the Nobel Prize-winning economist, Al Roth, matching is about how we get the many things we choose in life that also must choose us, i.e. not only by what we make out of the choices, but by the availability of choices.
uber
For example: a candidate cannot just email the admissions committee of IIM-Ahmedabad that I am enrolling for your MBA program. The candidate must go through the admission process, i.e. she has to be admitted. Parallelly, IIM-Ahmedabad cannot solely dictate who will consider the Institute for graduate studies, i.e. IIM-Ahmedabad must be chosen by the candidate. Formally, as suggested by Roth, a market involves matching whenever price isn’t the only determinant of who gets what.
If you have ever been involved in labour market, i.e. have tried to look for job or otherwise, have tried to hire someone; asked for a date with someone or have been asked out for a date; have tried staying at an Airbnb lodging or have hosted someone at your place; have asked for a travel ride or have driven someone around: you have participated in a matching market.
These matching markets are everywhere around us (
, Rooms, , , , Urban Company) and COVID-19 has impacted these platforms differently -- where demand for Airbnb, OYO rooms, Uber and Ola has decreased; whereas activity on Tinder, has gone north.To understand the working of matching markets in a COVID world, we rely on the three-pronged framework defined by Roth in his seminal work on the art of designing markets. According to Roth, to function efficiently, markets need to get at least three things in order:
1. Thickness
Matching markets must provide thickness — i.e. there should be enough people on both sides of the market (buyers and sellers) to generate satisfactory outcomes for both the parties.
Platforms like Uber and Ola need to have large proportion of riders and corresponding drivers to give them a ride; Tinder needs people who can swipe right and corresponding people who want to be matched; Airbnb requires guests who are looking for rooms to stay and therefore, corresponding hosts who want to open their houses for these guests; Urban Company needs enough customers who are looking for its services and corresponding service providers to cater to these customers.
2. Safety
The matching markets need to make it safe for parties at both the ends of the market, to reveal or act on any confidential information that the participants are privy to.
Uber wants the riders to feel safe while riding on their platform and Airbnb wants the hosts to trust the guests and open their personal space for them to stay.
3. Congestion
As all good things come with a price, congestion follows thickness and the markets need to override the congestion. This can be achieved by providing the market participants enough time—or the means to conduct transactions fast enough—i.e. participants should stay on the platform long enough to make satisfactory choices among various alternatives but also leave the platform to make way for the other participants to function smoothly.
Airbnb does not want the guests to take forever to go through the multiple host options but also would not like the guest to leave the platform without making any choice.
All things considered, it sounds like only the top players or the Goliaths (the giants) are at an advantage here. We discuss in detail why that is not the case and how smaller players or David (the underdog) can overcome the odds and be successful.
First:
We need to see if thicker markets always lead to higher matching rates? It sounds like it should, i.e. more Uber drivers, more the riders and more satisfied customers? Digital platforms have expended significant effort acquiring customers and fueling growth.
In fact, platforms like Uber, Ola, Airbnb spend billions of dollars a year on marketing campaigns and incentivising drivers and passengers. One key difference between the matching markets and traditional platforms is diversity of elements -- both from the side of participants (demand and supply) and among the goods or services that they offer or desire.
In such diverse and large markets, search is an important factor in finding the right match. However, research suggests that with large and diverse markets comes transactional friction between buyers and sellers, which in turn prevents them from finding an instantaneous match. More the choices, more the expectations, more in turn is the chance that a match exists which also implies that more is the expected time to match!
One thing to understand here is that matching markets have deadlines: a guest needs to book an Airbnb place before the intended travel date, an Urban Company user looking for Diwali home cleaning services needs to get the cleaning done before Diwali, a Tinder date looking for a match has a set need and desire in mind as she plays between swiping right and left.
As time constraints become an important factor, time taken to find a match becomes more prominent. Researchers focused on an Airbnb like rental marketplace operating in Australia and found that when the number of participants on the platform doubles, traveler confirmation rate goes down by 15.4% and the host occupancy rate goes down by 15.9%. In total, the platform lost 5.6% of potential matches daily, simply due to the increased market size. These problems are unlikely to prevail for young players for whom the market size is small and there are not many members on the platform.
Second:
Matching markets facilitate value-creating transactions. Being transparent, i.e. providing detailed and complete information to the market participants about the goods is expected to be a way to ensure trust and safety.
Therefore, matching platforms spend billions of dollars on gathering granular data on each user. In many cases, the relevant information is only partially revealed to the users. For example, Uber currently does not convey to the drivers the passenger’s destination until after the driver has picked up the passenger.
Similarly, Airbnb hosts who turn on the Instant Book feature commit to accepting all requests without knowing some relevant details of prospective guests, such as age or gender. Since evaluation by buyers and sellers on the digital platform involves buyers and sellers reviewing and scrutinising the attributes of each other, conventional wisdom suggests that more the information that the seller and buyer know about each other, more valuable the transaction.
Research on the other hand suggests that revealing too much information about an individual can lower the value of that information as well as of the individual. This means that for trust building and speeding up the rate of transactions, optimal information to disclose is not complete information but incomplete information.
Small players anyways do not have the resources and capabilities to collect granular data at the scale that bigger players collect in the first place and therefore, they anyways are at an advantage as they do not have complete information to share between the participants.
Third:
As discussed before, searching through thousands of listings and user profiles on matching platforms is a time-consuming process. Further, demand for matches typically fluctuates over time.
An Urban Company service provider is expected to be very busy during holiday season but during COVID-19, she might be temporarily looking for extra work. A Tinder user might not respond to a right swipe when she is on a current date or can sometimes be looking eagerly for someone to swipe right. An Airbnb listing may be unavailable for a date because of a visit from the host's own relatives or family. These changes are not immediately reflected on their profile, or even known to the platform.
As a result, a great deal of congestion is likely to be on the platform -- users who are temporarily not available on the platform, but their current status is not available to the platform and this creates congestion. Therefore, features like limiting the usage of a member on the platform works.
For instance, Tinder limits the number of likes/right swipes permitted in a certain period. Airbnb hosts charge a cancellation fee (severity of which varies from host to host). Again, the problem of congestion is less likely to occur for the smaller platforms with limited resources and platform participants.
Overall, we are confident that by following an informed, data-driven approach, small and medium matching platforms are at an advantageous position than their larger counterparts.
(Disclaimer: The views and opinions expressed in this article are those of the author and do not necessarily reflect the views of YourStory.)