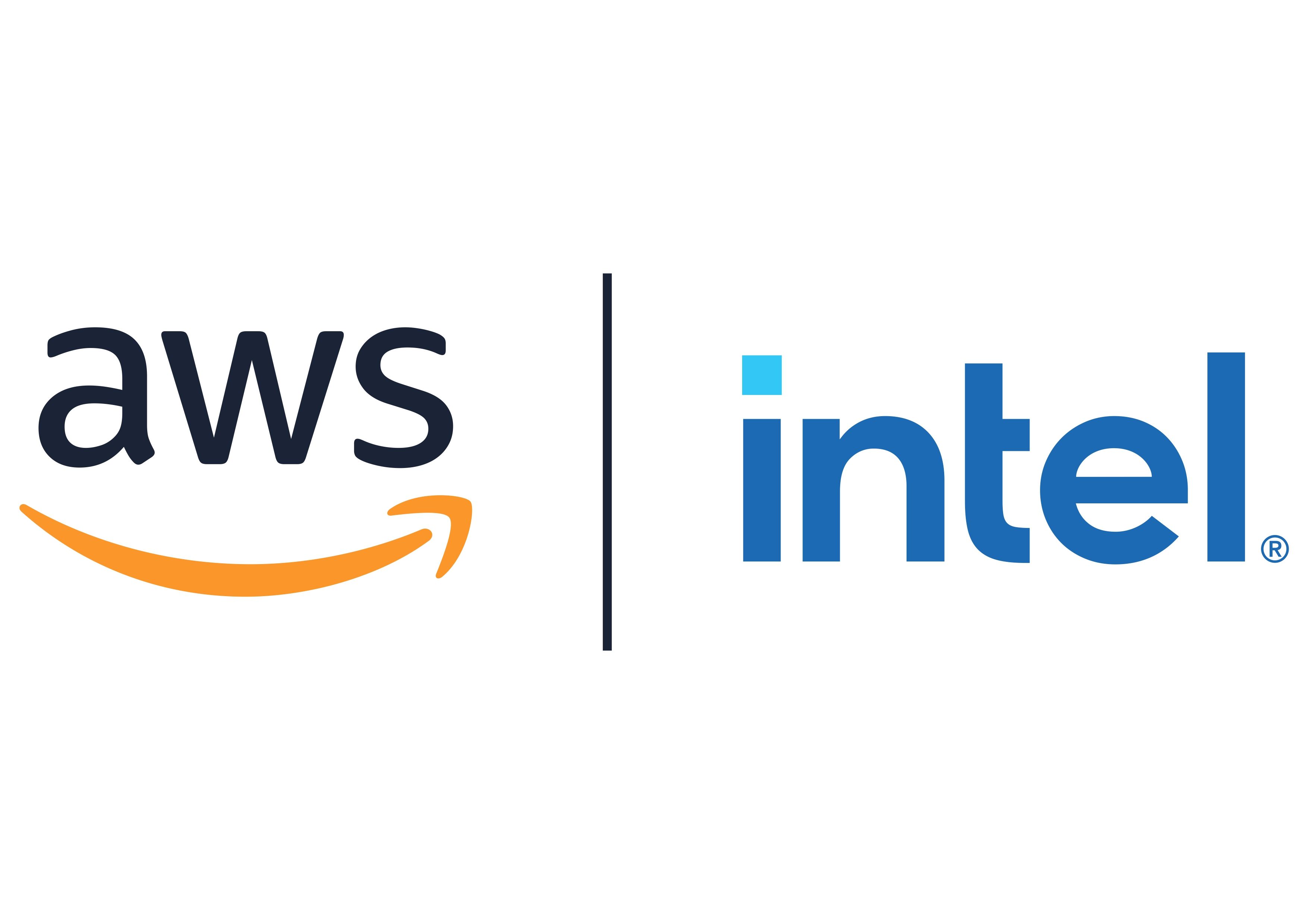
AWS | Intel
View Brand PublisherMaking games immersive and engaging through AWS AI/ML
Artificial Intelligence-driven solutions and Machine Learning (AI/ML) tools can potentially have a huge impact on various aspects of the gaming sector. From improving the overall gaming experience, enhancing the games intelligence and visuals, detecting fraud, predicting player behaviour and more, AI/ML offers significant solutions to game developers and companies.
To discuss this revolution in gaming, a session on Building Smarter Games with AI/ML was held on August 26, 2021, where Yogish Pai, Technical Account Manager, Amazon Internet Services Private Limited; and Arun Kumar Lokanatha, AI/ML specialist Solution Architect, Amazon Web Services; covered the key ways in which AI/ML can be utilised to solve problems in gaming.
The session covered the following topics:
- How AWS can help you with AI/ML in games
- Common use cases which AWS customers are solving in gaming with AI/ML
- Common architectures and demos
How AWS can help game developers
Yogish opened the session by stating “AI/ML on AWS provides one of the broadest and deepest set of features for various use cases.” He spoke about three key areas where AWS can help game developers:
- Building games faster, thanks to 10x better-performing algorithms and 2x the performance compared to other cloud providers
- Creating new and more immersive experiences
- Building ML models to help in player prediction and engagement
ML use cases in gaming
Yogish categorised ML use cases in gaming into different areas, such as personalisation, monetisation, voice, anomaly detection, ML agents, video and more. He then expanded on how games customers used AI/ML in each of these categories.
Personalisation
Yogish explained how predictive algorithms (which come under the AI domain) use the data gathered about a player to predict what a user’s likes will be, and create models and frameworks around player behaviour. He said, “Such a model then can be used to recommend what offers or services can be proposed to these players and these levels of engagement and personalisation truly involves the player in the gaming ecosystem.” He spoke about Amazon Personalize, and explained its impact on both in-game and marketing outcomes. For instance, Personalize can be used to create real-time recommendations in game, offer customised suggestions and periodic updates to store catalogues, and more. With regards to marketing, Amazon Personalize could be used to retain customers through tailored content and to use player history to recommend the best contests and identify spending habits. He also cited the case study of how Sony Interactive Entertainment modernised the PlayStation Playstore, using Amazon SageMaker and Amazon Personalize.
Monetisation
In terms of monetisation strategies, Yogish stressed that the focus must be on retaining high value customers. Specifically, how games should encourage players to spend in the app sustainably, instead of a “churn and burn '' model. In this regard, tracking LTV (Lifetime Value), examining the past spending habits of players and game data can help companies predict the likelihood of a player making a purchase. He spoke about using Amazon Sagemaker to predict those who would abandon the game, so that developers could remedy the situation and retain the player through promotional offers. Additionally, he also spoke of using ML models to recommend relevant items that the player can purchase to help them while they are playing. He then encouraged attendees to look up the Rovio and the Angry Bird case study and how the company used ML to understand the playability of the game.
Video and anomaly detection
Yogish stated, “Fraud is a very problematic scenario for many studios, because it actually devalues your in-game currency that is bought with real money, which could erode the player’s trust in the game.” To combat this, one of the solutions Yogish spoke about was creating custom models on Amazon SageMaker to help companies and game developers detect whether a human is playing the game with inhuman skill and proficiency. The case study he referred to in this section was Krafton, a South Korean-based game studio that uses custom SageMaker models to detect fraud and abuse.
Under the voice category, he recommended the use of Lex or Alexa to add voice commands to control the characters in the game.
AI/ML architectures demos
Yogish then handed the session over to Arun Kumar Lokanatha, an AI/ML specialist Solution Architect, who works with different startups across different industries and sectors. In this section, attendees were taken through live demos on how game developers can quickly build these architectures using AWS’ AI/ML stack.
Before he started the demos, Arun took the attendees through the three-layered AWS AI/ML stack.
- Top layer: (AI Services) In this layer, developers don’t need to worry about infrastructure management or ML code. This layer offers API-driven services.
- Mid layer: (ML Services) is Amazon SageMaker, which gives developers 20+ services across the ML lifecycle.
- Bottom layer: (Framework and Infrastructures) This layer offers framework support and infrastructures, both in terms of CPUs and GPUs.
For the demo sessions, Arun discussed various AI/ML services and demonstrated how to build solutions using these services. He also discussed the mid-layer of the AWS AI/ML stack - Amazon SageMaker - in terms of building, training and deploying models.
Proactive player retention: Arun spoke about Player Churn Prediction and Retention, and using Amazon SageMaker to build, train and deploy a churn prediction model for batch or real-time interference. He then demonstrated how to build this model on the SageMaker dashboard.
Personalisation: The second user case for player retention involves recommending personalised content to the user. Developers can use Amazon Personalize to build the model. Arun then took attendees through the architecture of the model.
Anomaly detection: Arun demonstrated how developers could leverage services in the AI/ML stack to counter fraud, through solution architectures on Amazon SageMaker. He also demonstrated a new feature - Amazon Lookout for Metrics, which uses ML to detect anomalies and identify their root causes in any business or operational metrics.
The session wrapped with a few ways that Amazon could help game developers. These include immersion days, workshops and training, certifications, funding support, guidance from ML experts and more.