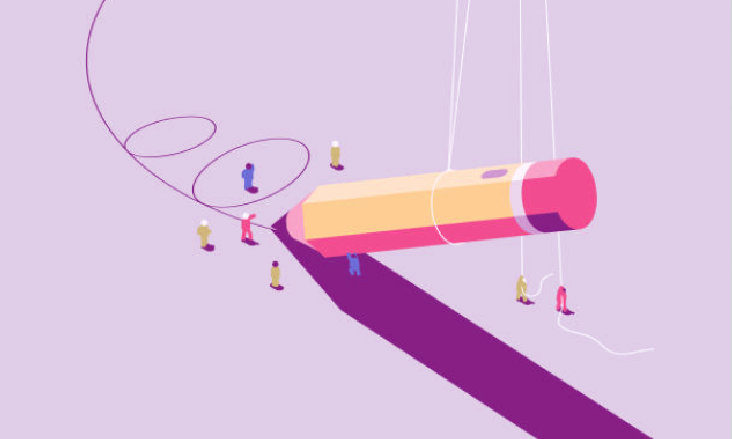
Machine learning is intriguing. It sounds high-tech and many avoid it altogether. What does it actually mean and what good can it do to my business? These are the first obvious questions many of us will instantly think of when reading this peculiar term.
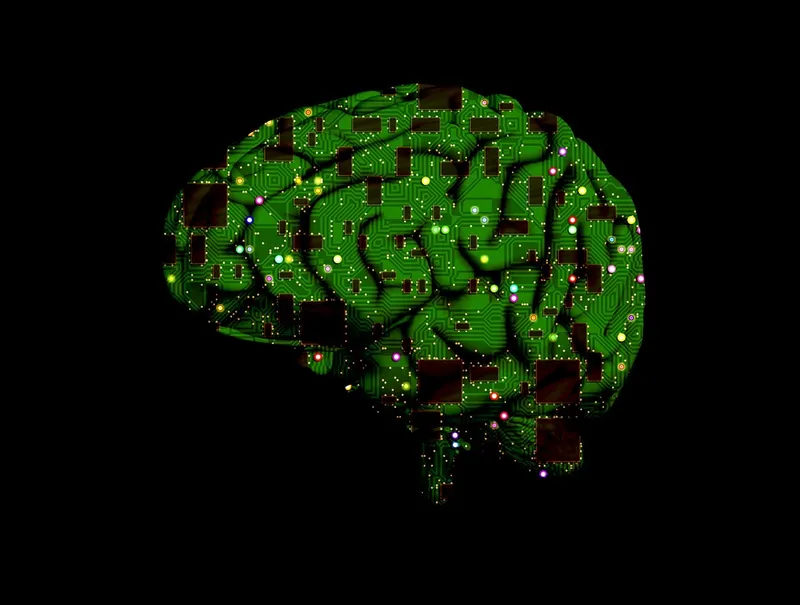
The basics of machine learning
In a nutshell, machine learning is all about detecting patterns. Regularities of data, similar predictions or outcomes, can be considered patterns. The domain is vast, and data scientists talk about machine learning as a new method of understanding and using artificial intelligence. However, what you need to know, for marketing purposes, is that machine learning can be used to identify patterns. Essentially, detecting behavioral patterns for customers, advertising campaigns, spending and so on.
Why would you need machine learning for marketing?
Let's say you own an online toy store. You want more customers for your products, and you create a marketing campaign. You want customers who can afford your toys, let's say customers who spend more than $50, so you direct your campaign towards them. After you launch the campaign, you check out the analytics, but, unfortunately, they don't say much. You can't exactly pinpoint what went wrong and what was successful. You don't understand what will or won't work in the future.
This is where machine learning comes into play. It helps you understand patterns in analytics, what customers do, what they look for, what they buy and what they don't buy. It helps you detect behavioral patterns among your visitors and helps you create your future campaign. Simply put, machine learning sees patterns where we don't.
So how can you implement machine learning in your marketing campaigns? What are the techniques that do work today? Let's take a look:
1. Clustering*
Clustering works by breaking up large groups of items and regrouping them in smaller, compact groups. It's a family of techniques which divide data in K groups, where K is the predetermined number of clusters. The goal is to create subgroups or subsets of closely related items. Basically, you segregate groups with similar traits and assign them to new clusters.
Let's say, for instance, your toy store has 100 customers. Obviously, you can't create a marketing campaign for each individual customer (all customers are unique and want different products). With clustering, you can create subgroups of clients, say 10 customers in each group who have similar needs. For instance, you could create a campaign for customers looking for inflatable toys – you need to direct it at the “inflatable toy cluster”.
2. Decision Trees**
Decision trees marketing is also known as segmentation. It's a method based on demographics, and includes socio-economic, cultural, ethnic, religious or political factors. Segmenting your customer base is a key technique in determining who to target in a future marketing campaign.
For instance, you are a manager for a large magazine and you have a database of 1 million previous subscribers. You want to contact all of them, but you only have budget for 100,000 letters. How do you choose which customers you contact? The answer: through segmentation and decision trees.
Essentially, segmentation creates separate classes of potential customers who may respond more effectively to your offer. Customers earning less than $50,000 will have a response rate of 0.4 percent, while customers earning more than $50,000 will have a response rate of 0.8 percent. Now, you have two different decision trees – a two prong graph. As you add new classifying criteria (in some cases up to hundreds of different variables), the trees “grow”. For instance, the 60 percent of customers earning more than $50,000 own cars, while 40 percent don't. Segmentation is about going through these different levels and growing the decision tree, narrowing your target, and getting the best results for your campaign.
3. Naïve Bayes***
In machine learning for marketing, the Naïve Bayes classifiers are a family of probabilistic groups based on Bayes theorem. Also known as the inverse law of probability, Bayes theorem describes the probability of an event based on historical patterns or similar conditions related to that future event.
For instance, if diabetes is related to obesity, using Bayes' theorem, a person's weight may indicate a risk of diabetes. Essentially, one can conclude that an obese person may develop diabetes just by correlating his or her weight with the statistics.
Let's take a different example. A bank which offers multiple financial products to its customers, including saving accounts, mortgages, insurance policies or credit cards, wants to create a campaign for new customers. Data accumulated from previous customers, for instance mortgage customers may predict similar models for future clients. A person who is 27 and earning $40,000 is an ideal candidate for a new mortgage, as previous data suggests. Consequently, the marketing campaign will be directed at similar potential customers, between the ages of 25 to 30, with a median wage of $35,000 to $45,000.
References:
* https://www.analyticsvidhya.com/blog/2016/11/an-introduction-to-clustering-and-different-methods-of-clustering/
** https://www.linkedin.com/pulse/decision-trees-marketing-ram-n
*** http://bridgei2i.com/blog/naive-bayes-technique-for-machine-learning/