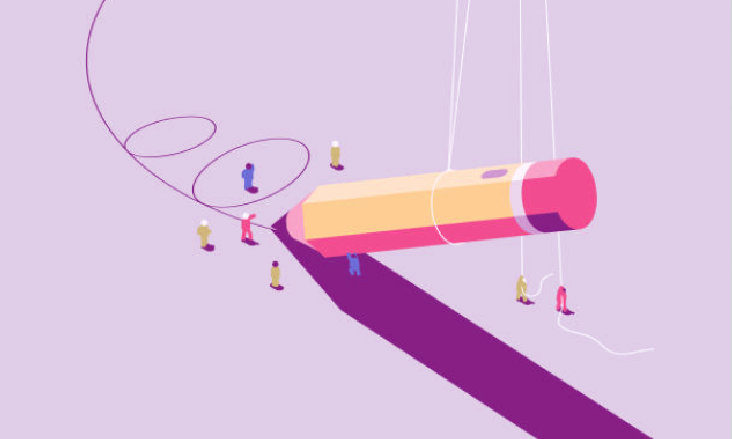
6 Myths about Machine Learning That You Thought Were True
Many believe in many myths about Machine learning so let's have a look at some them.
My first encounter with machine learning was at a workshop in the 1990's. This being the time when the computers were not so common, I was anxious to know how will something like machine learning work. I soon found myself engrossed in reading and researching more on what it is, how it works, and all that needed to be known. My quest got me to a point where I knew almost everything that existed about machine learning.
During my learning phase, I was told a few things about machine learning that I believed were true and I am sure there are many who like me believe these myths. I have tried to compile a list of these things and explain why these are not correct. Nowadays, many providers offering big data services, claim that machine is learning is immune to failure, unbiased, and can work in almost any setting. But, these are anything but true. Let’s evaluate each of these individually.
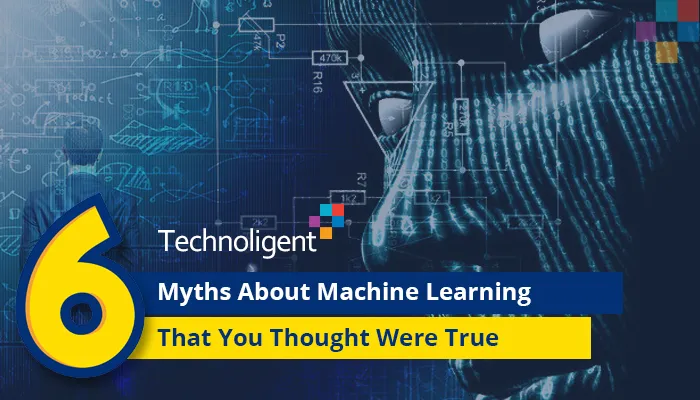
6 Myths about Machine Learning
1. Immune to Failure
This point applies to two sides of machine learning and the one that I am talking about is the development phase. Different situations demand different machine learning solutions. Therefore, if a situation is not well thought about, the solution that is being proposed or applied can result in absolute failure. It is a well-known fact that machine learning works on algorithms. This means that the success of a solution largely depends on the right algorithm and to figure that out, you need to have a clear plan in terms of what data you have and what you really wish the solution to do for you.
2. Works Anywhere
This is a blatant lie. Machine learning is used only in cases where you have big data sets. You would not want to spend hundreds and thousands of dollars to create a customized solution to analyze a data set that a human can do effortlessly and in less than half the money that you would spend in getting a machine learning solution. Therefore, a machine learning solution is suitable only for people who are using big data services. This limits the scope of “anywhere.”
3. Is Unbiased
The fact is that machine learning operates on data sets and analyzes data that is fed into the system. If the source data is one-sided or prejudiced, then the result or the analysis will be biased. Now, this is not entirely the machine learning solution’s fault but as a whole, it is a factor worth considering. This cautions the users of machine learning solution to not blindly rely on the analysis but also look at the data pool to be sure that the results are impartial.
4. Works Without Human Intervention
The phrase “machine learning” can be pretty confusing initially. The actual concept is how machines learn without actual programming codes. But, that is not a possibility. The algorithms that a machine learning solution is based upon are created by human. So, practically, human involvement is a mandate. There is no doubt that employing machine learning limits or eliminates the involvement of human from some critical steps. But, then human intervention cannot be ruled out completely.
5. Is the Future
This can be partially true but in my opinion, there are far more promising technological advances that can take the place of machine learning in a more enhanced way. Some years back, self-driven car or automatic predictors might have been a dream, but now these are a reality. Similarly, there are more advances that can be the future of analysis.
6. Is Same as Data Mining
A lot has been written and said about the difference between machine learning and data mining but these are often confused to be the same. In simple terms, data mining is used to identify properties or patterns that are unknown and machine learning is employed to use the existing patterns and properties to reach a meaningful conclusion. There is a thin line separating the two and both data mining and machine learning work on similar principles but the fact is that these are different.
Machine learning has opened a new door for human imagination and things that were unimaginable a few decades ago are now a reality. But, as it looks everything is not positive about machine learning. Many believe that machines can never learn and human involvement is inevitable. This belief is factually correct at present; but, you never know what the future holds for machine learning. Human involvement is not the only criticism for machine learning, the fact that machines are taking up work that humans were previously involved in has a lot of substance. In this case, the former criticism answers the concerns of the latter. Only time can test the real worth of machine learning and we are all watching the clock tick.