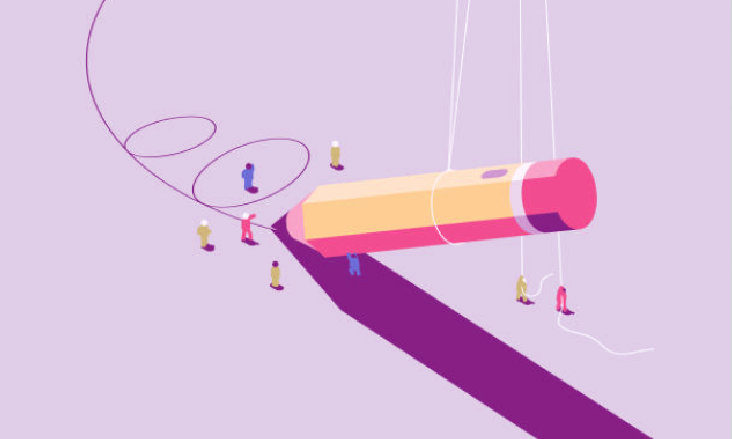
Data mining pumps up organisational ROI; If done right
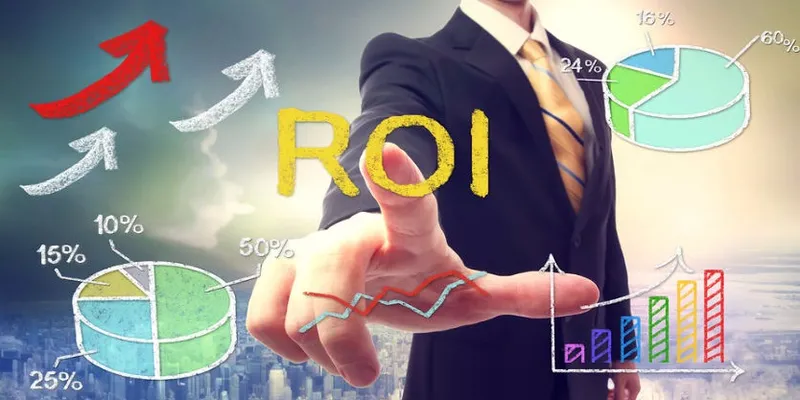
Data is now everywhere and is still growing at a really rapid pace. It is amplifying every alternate years and it is successfully altering our existence. If IBM is to be believed, today approximately 2.5 billion gigabytes of data was generated till 2012, followed with comments from Forbes that data is doubling up at a pace more than it got generated. They also suggest that by 2020, nearly 1.7 billion of data points or information will be generated per second from human inhabitants of this planet. With this increase in data at lightning speed, new terminologies and requirements associated with data processing and data management are also emerging.
Data mining is no more a plain vanilla flavor practice of searching large data sets for data patterns. Instead now it has evolved into something as various other fields including AI, machine learning, data room virtual base management, pattern recognition, data visualization, statistical studies and so on are getting clubbed to it. With this evolved avatar of data mining it is no more about extracting information from various data sets to transform it in appropriate and comprehendible structures for eventual use. One more element that spices up the data mining aspect is data warehousing, the game changer is what it is.
Organizations are running to adopt new technologies; but there are none, which are not powered by data. This makes data mining, data processing and data management; more important than ever.
With so much of aggression in advent in data generation, data mining and data processing; it makes case enough to revisit the basics at least once. This not only would give us a better understanding of the “how and what of data mining”; but also would help in gaining insights about evolved data mining science.
Data mining is not a stand alone process
Data mining is known to be a simple process to discover information & unseen patterns from existing data, however; it is not that simple a process. It depends on the source from the data is supposed to be mined from. If it is the databases the mining is to be done from data in structured form, but if it is to be done from data warehouse, the data is not in a structured form. The structure of data is of so much importance, as it defines if the data is compatible for processing. Data mining clubbed with data cleansing primarily concentrates on cleansing, aka data scrubbing or noise elimination, of data to make it processing ready.
Next important thing that is supposed to be looked at is whether data mining to be done is for static data or for dynamic data. As you all know, handling static data is much easier a task, as compared to managing dynamically varying data. See what happens with static data sets is that the entire data is readily available for analysis, well before processing; and is usually never a time varying data. The case with dynamic data is entirely different. It is like high voluminous consistently differing information which is not a stagnant data. It is also not readily available for processing and to be analyzed. May it be static or data store items like Photos, Files, Database Record etc., or dynamic or data stream such as Live video/audio feed, Stock Market feed; both the sources are being used for data mining or are data mined for various purposes in varied proportions.
Data processing for database vs data streams
Existing data mining algorithms used for clustering, classification and finding frequent patterns are designed and suitable for static data sets only. They become obsolete when it comes to mining stream data. Data streams comprise of temporal, time series or spatio temporal.
Furthermore, currently used data mining algorithms used for clustering, classification and finding frequent patterns fulfill needs of static data sets only. They are not equipped to handle data streams or to mine stream data. Universally utilized concept of clustering and classification is the most preferred ones for current data mining researchers.
Data mining algorithms, conventional vs evolved
Irrespective of the data type that is to be handled, including audio/video signals, sequence/ sequential data, temporal /spatio- temporal data, time series etc.; algorithms or various methods are used to analyze targeted data. Infinite sequence of data points in form of time stamps or indexes, known as data streams; is more than popular among data miners.
It also can be looked upon as data equal to multidimensional vector with integer, categorical and graphics with data in either structured or unstructured format. The fact that we cannot run away from is that more and more data is becoming dynamic as more and more data is generated from various applications and devices. This poses humongous challenges when it comes to analyzing data streams, making conventional data mining algorithms obsolete. Conventional algorithms were designed to conduct multiple data scans over the data which is not at all possible while handling data streams.
Research issues in data mining & problems in handling data streams
1. Memory constraint
The amount of memory which is required by the stream mining algorithm that is being used or designed, for handling streaming data; is one of the top priority factors that needs to be considered. This is due to the reason that with data streams, the data that is generates is not regular and mostly generated at inconsistent time intervals. This compels the algorithm to optimize the memory utilized for data processing. Since the data that we are discussing is dynamic, is not available completely at the time of processing and also is not in appropriate format. The amount of data generated is humongous and is growing while you read this. This has increased the challenges of handling streaming data.
2. Data pre-processing
This is also one of the criteria to be taken into consideration while data mining. This challenge generally arises when data mining is conducted using existing data mining tools or algorithms. Data mining tools available have diverse formats for inputs making this a time consuming, laborious a process. It also increases the chances of data loss.
3. Dimensionality reduction
The next that comes in is dimensionality. Mathematical and statistical approaches are put at task to study the problem of reduced dimensionality. However; when it comes to handling static data and data with streams; this challenge raises head – inevitably.
For example a text data, contains several unnecessary and unwanted features which certainly do not contribute at all, towards the decision making or the analytics. It is more than important to take care of reducing dimensionality of the data which may result in prolonged algorithm’s running time. This also improves the memory requirement, thus attaining space efficiency of the data mining algorithm.
4. Choice of data structure
If you have a suitable and effective data structure, it is like reaching half-way to success; while designing algorithms for handling data streams.
Conclusion
It has become quite typical to handle unlimited, enormous and humongous data volume of dynamic, irregular and variant nature, which is generated from infinite number of applications. Data mining solution providers are experts at handling streams for clustering, classification and topic detection. One of the latest observations is that several companies and organizations are still struggling to incorporate data into their strategies, not because they don’t have experts to do it or they don’t know how to do it. But because, they did not handle the data mining task, in that correct manner. Companies did gather data, but because it was not in the correct format; now is becoming a challenge to define what it can be used for.