How Artificial Intelligence is revolutionising respiratory care
During COVID-19 pandemic, deep learning algorithms based on image analysis from chest radiographs and CT scans were used to identify active infections.
Artificial intelligence (AI) in the near future has the capability of transforming the delivery of healthcare services.
With the growth in data and healthcare information from various electronic mediums, which is roughly estimated to be one billion gigabytes in the US alone and growing 48% annually, AI has the capability of integrating data related to genomics, medical imaging, and electronic health records and more.
For the pulmonologists, the COVID 19 pandemic has truly exposed the lacunae in the existing healthcare system and has made them believe that understanding and incorporating AI in clinical research and practice is the way forward. Deep learning, which is a subfield of AI wherein features needed for a particular task are automatically learned from the raw data provided, is gaining prominence.
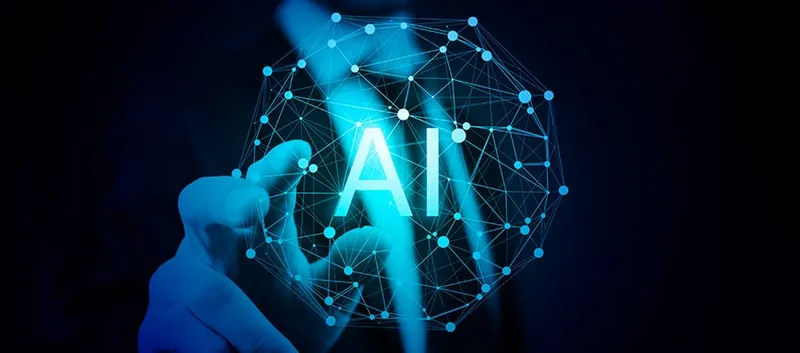

How patient e-consent process can help doctors and evolve healthcare sector
In machine learning, datasets, functions and algorithms are used to search through data and identify output values that best match the data. Machine learning can be supervised learning where an algorithm in which each input of the dataset is matched to a specific output value.
In unsupervised learning, algorithm finds clusters within the input data. Another aspect of machine learning referred to as reinforcement is useful for control tasks such as those needed in robotics for constructing policies where medical decisions require sequenced procedures such as in radiation therapy of lung cancer for formulating treatment protocols for destroying cancer cells while sparing as many normal cells as possible.
In the current scenario, AI in pulmonary medicine is aiding in computer vision for lung nodule detection and prediction risk for malignancy. Detection of early lung cancer by using low-dose screening computed tomography (CT) has been shown to reduce mortality by 20% in clinical studies.
Current evidence suggests that deep-learning algorithms have better or equivalent accuracy compared with readings by expert radiologists for detecting early lung cancers on low dose CT Chest.
Another domain where AI is finding a role in pulmonary medicine is in analysing radiological images in diagnosis of pulmonary fibrosis. Experts observed that the median accuracy of thoracic radiologists was 70.7% compared with 73.3% accuracy of the algorithm in the classification of fibrotic lung disease by HRCT based on the Fleischner Society and other international respiratory society guidelines.
They also observed a good inter-observer agreement between the algorithm and expert readings. Research work is also being carried out to utilise machine learning in developing an algorithm based on genomic data from surgical biopsy samples in order to detect molecular signatures for usual interstitial pneumonia (UIP).
Machine learning is also finding its place in developing predictive models in pulmonary hypertension, asthma, pleural infections and pulmonary function tests.
Currently, in asthmatic patients, machine learning algorithms are used to phenotype asthma patients to help in predicting patients’ responsiveness to steroids. Based on a study using AI-based machine learning, asthmatic patients who are older and those with the latest age at onset, more nasal polyps and high blood eosinophils were identified to be steroid responsive. Asthma patients with obesity, female sex and early onset were observed to be non-responsive.
Another exciting area in pulmonary medicine where AI is already finding its utility is in the interpretation of pulmonary function tests where AI algorithm perfectly matched PFT pattern interpretations (100%), and assigned a correct diagnosis in 82% of all cases facilitating early and effective decision making.
The major utility of AI was explored during the time of COVID-19 pandemic when the technology was used to elaborate early diagnosis and treatment strategies. AI epidemiology algorithm called BlueDot, which skimmed foreign-language news reports and official announcements was used to provide clients with news of potential epidemics.
The same algorithm was also used during the international spread of the Zika virus in South Florida in 2016 to predict spread.
During COVID-19 pandemic, deep learning algorithms based on image analysis from chest radiographs and CT scans were used to identify active infections. Another deep learning platform AlphaFold, developed by Google DeepMind, was used to predict the structural protein shape of COVID-19 which provided vital information for COVID-19 vaccine developers.
However, AI is not without limitations in its current form. Risk of hacking and data breaches and potential of revealing patient identity are serious concerns. Overdependence on automated machine-learning systems or increases in medical errors are also observed in few existing AI platforms.
With rapid advances in technology, algorithms which will meaningfully ingest more data and process massive sets of data in an accurate and rapid way may emerge. Future pulmonologists will inevitably incorporate AI into clinical care to meet the unmet needs in the existing healthcare model.
Edited by Affirunisa Kankudti
(Disclaimer: The views and opinions expressed in this article are those of the author and do not necessarily reflect the views of YourStory.)