The Awesomeness Of Data Interpretation: Manu Sharma, Principal Data Scientist, LinkedIn
When LinkedIn was launched in 2003, it took them 500 days to reach their first million customers, but after that the next million signed on in just 6 days. Today there are two new registrations with the site every second and the data analysis team looks at 200 TB of data each day to understand its users better.
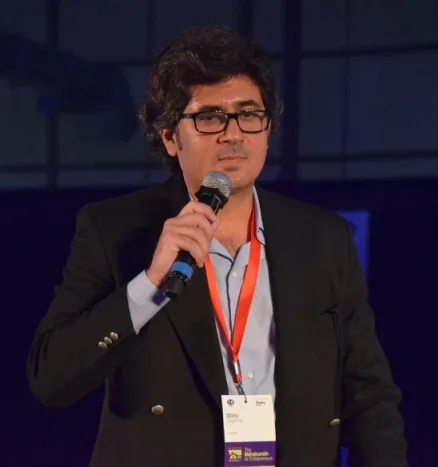
“As a data scientist, my role is to understand data, analyse it, set standards, derive insights and use them effectively to help customers use the platform and improve business,” said Manu Sharma, principal data scientist, LinkedIn, while addressing the 1200-strong crowd assembled to hear his power talk at TiE Mumbai. A lot of existing solutions in the market doesn’t work for LinkedIn because of their need to intelligently use data. As a result they have to come up with their own unique solutions to solve the problem at hand. Some of the recent data led innovations have been the ‘People You May Know’ section which the networking website introduced sometime back and the skill endorsement facility — where you can endorse your contacts for skills they possess.
According to Sharma, there are 8000+ variants of ‘IBM’ on Linkedin and 6000+ different ways of writing ‘software engineer’ on the site. “And these are just what we have come across so far, they could be more different ways of writing IBM or software engineer on the site,” explains Sharma. Given such challenges, Sharma says standardization of data is very important, as that is the key to building compelling products. “Do you know the top name in engineering on LinkedIn is not Peter or Daniel but Rajesh and its data which told us that,” says Sharma.
The power of data analysed by LinkedIn was also used as a reference in US President’s report last year which dealt with employment in various sectors. Internet, online publishing and philanthropy jobs were most popular areas for jobs, while areas like newspapers and restaurants performed very poorly in the report. Besides plain analysis, strategic analysis of data on site is also equally important said Sharma. “You have to understand the value of action that the user takes when on site. Early behavior on the site, can be used to predict the future engagement with that particular user.”
The best practice in terms of data, according to Sharma is to have more data, than less data. Raw data is better than processed data. Data standardization and data quality is key to taking the right decisions. Simple models of looking at data are always better than complex models and it’s better to fail fast, iterate and test...test...test your product, because that according to Sharma is the only way to succeed.