It is English that makes Amazon stay up on top
Amazon.com has thousands of classes in product taxonomy and they have to understand the semantics of search.
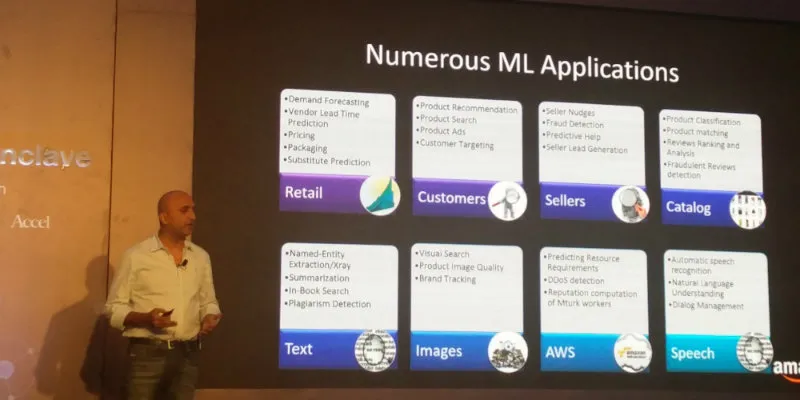
Some engineers may wonder what makes Artificial Intelligence (AI) and Machine Learning (ML) so effective – the answer is the data in the form of numbers.
Think about it - modern devices and search engines are actually understanding the way you interact in search of information. Voice and Text are being converted to data, and from thereon, the software understands the patterns of conversation in numbers.
So, it is in the phrasing of the language, and it is in that context that engineers must figure out how to build machine learning services. This is the consumerisation of IT, where consumers and customer interactions are translated back to optimising processes in retail stores and manufacturing plants.
“This is what Amazon does with millions of its sellers worldwide and in India,” says Rajeev Rastogi, Director of Machine Learning at Amazon.com.
If some of you wondered how Amazon is able to stay on top of the retailing business worldwide, is because it is able to demand forecast better. It is able to use its ML capabilities to understand consumers behaviour, reviews and expectations. This data is used across its fullfiment centres to optimise inventory and preparing its sellers.
So, if sellers wonder what works in Amazon, then it is the algorithm that knows its English well. The ML algorithm decides what should be stocked based on consumer demand and reviews. It decides this based on data of 90th percentile demand, only then does Amazon stock this in its warehouses and fullfiment centres.
Amazon uses Use ML to address
- Business priorities
- To increase product selection
- Lowering prices
- Reducing delivery times
- Eliminating friction and maintaining customer trust.
“We can deliver in select cities in less than two hours thanks to machine learning. We are piloting that with our drones we can do it in less than 30 minutes by dropping of in designated delivery areas,” says Rastogi.
The main theme is to allow consumers to discover products with minimal friction.
The machine learning platform can also track fake reviews and counterfeit products. Amazon can today use ML to figure out a retail strategy by region, and understand customer traits, it can currently train and prepare sellers and integrate their products with its extensice catalogue. The algorithms understand Text, Images and Speech to prepare the entire retail chain.
“We can forecast future demand and that puts our operations on top. Demand forecasting needs to be extremely accurate. Otherwise, we will be stuck with excess inventory. The challenges will be to scale to hundreds of millions of products, new products, identify sparsity, seasonal preferences, demand spikes and distribution of product. Machine Learning keeps your operations on top,” says Rajeev.
Adam Berns, head of the Alexa Voice service, speaking at the Amazon AI Conclave says the key challenge is to understand query semantics and identity phrases. “We have to show relevant results and we have partnered with the likes of Ford and HTC to show how algorithm understand humans very efficiently,” says Berns.
Amazon.com has thousands of classes in product taxonomy, and has to understand the semantics of search. For example, if a customer is using an edge device and activates the voice service and says to it, “I need laptop accessories for a particular brand and chargers for that series,” The algorithm should understand the context and make the right product available.
“Reviewing orders is the key. People make decisions based on this. Linguistic style varies between users, gauging sentiment may require deep parsing of sentences. This is how product recommendations work,” says Rastogi.
So next time you start data lifting with AWS, make sure you understand your customer interactions in language to programme ML and AI algorithms that can make your business user friendly.