From business improvement to innovation ally: how AI transforms the future of work
This provocative book explains not just how artificial intelligence is transforming business processes, but how the age of the Human + Machine calls for envisioning and creating new business models.
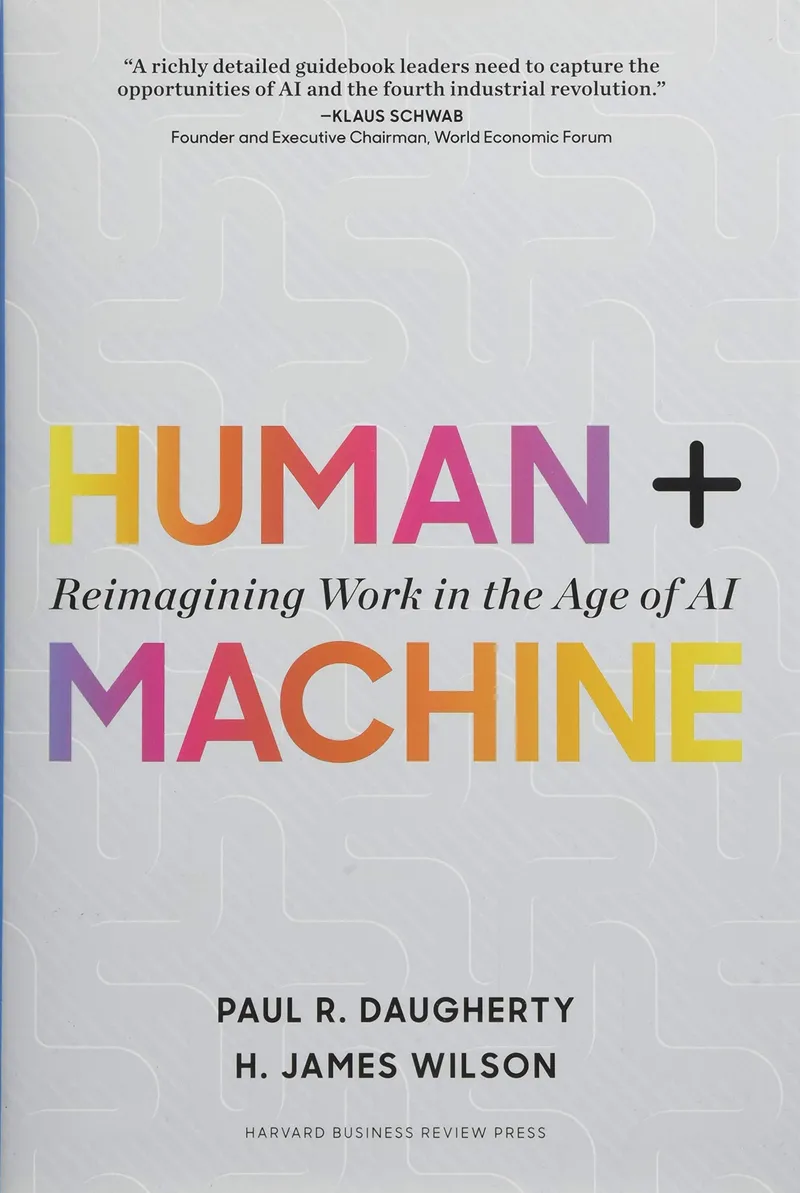
Artificial intelligence (AI) has come a long way since the first conference on AI at Dartmouth College in 1959; the term machine learning (ML) was also coined by IBM engineer Arthur Samuel that year.
Today, AI is transforming factory floors, corporate functions, R&D, and marketing, and offers new ways of envisioning business. AI has now broken out of the research lab and is fast becoming “the cornerstone of disruption”, according to Danny League, former ML head at Uber.
Frameworks and case studies of AI in action are detailed in the new book, Human + Machine: Reimagining Work in the Age of AI, by Paul Daugherty and James Wilson. The authors chart the growth of standardised, automated, and adaptive processes over the past century, and describe eight new fusion skills for enabling human-machine collaboration.
Paul Daugherty is the Chief Technology and Innovation Officer of Accenture, based in New York City. He leads Accenture's AI initiatives globally, as well as its Accenture Labs R&D facilities. James Wilson is Managing Director of Information Technology and Business Research at Accenture Research, based in San Francisco. He is the coauthor of What's the Big Idea: Creating and Capitalising on the Best New Management Thinking.
The eight chapters are spread across 250 pages, and cite dozens of online articles. The material is informative and makes for an easy read for managers, though there is quite a bit of repetition. I have summarised some of the takeaways in the tables below. See also my reviews of the books Life 3.0, Analytics and AI, and Big Data Revolution.
I. Foundations
The authors define AI as systems that extend human capability by sensing, comprehending, acting, and learning. AI will help not just with productivity gains but with designing and developing game-changing innovations.
The book does not dwell on the technical aspects of AI, but presents a three-layer framework connecting machine learning, AI capabilities, and AI applications (see my summary in Table 1 below).
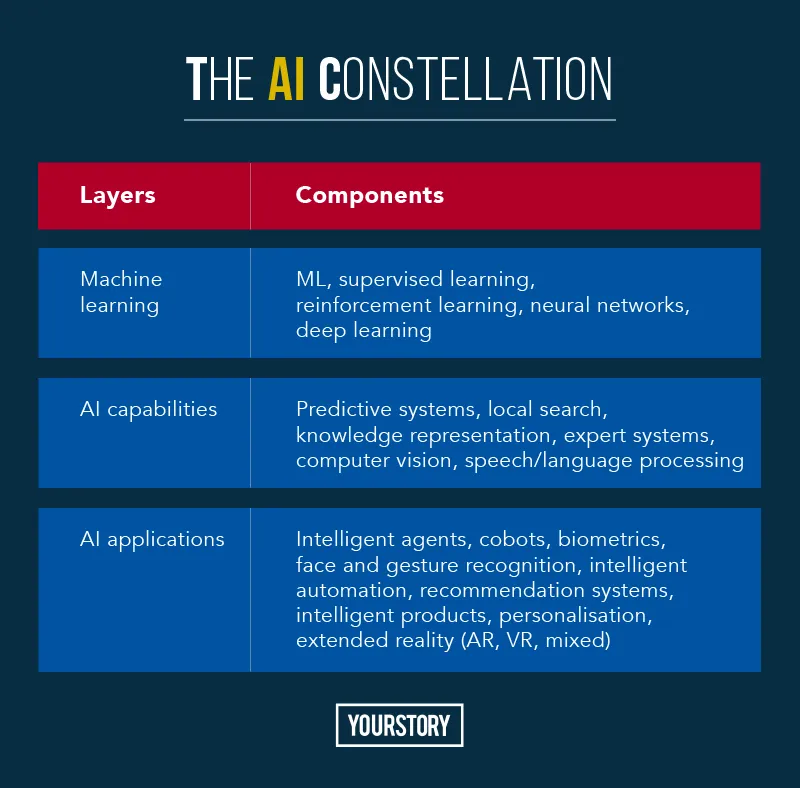
Machines are good at tasks that are repetitive, mundane, routine, and even risky for humans; their strengths are speed, accuracy, scalability and predictive capabilities. Software can process vast amounts of information in real-time, and present it in a visually appealing manner.
Humans are good at resolving ambiguous information, exercising judgement in difficult cases, and dealing with difficult people. Humans excel in activities that involve creativity, improvisation, dexterity, compassion, and empathy.
But rather than depicting AI as human-versus-machine, the authors explain that the real potential lies in the “missing middle” – AI and humans collaborating to achieve new gains through hybrid activities. AI is more than automation of linear, stepwise, sequenced, standardised, repeatable, and measurable processes. It helps envision new kinds of business models, and helps ask more creative questions.
The authors describe business transformation over the past century in three phases: standardised processes (Henry Ford’s assembly lines), automated processes (IT-driven business process re-engineering from the 1970s onwards, eg. Walmart), and augmented processes (flexibility driven by real-time data).
In that regard, smart machines and algorithms can augment human capabilities, and should be seen as symbiotic partners and not adversaries. Impacts of such collaboration are improved productivity, safety, and innovation.
Unlike standard algorithms with pre-determined paths and static code, AI senses, learns, and changes as it goes. Programmers become more like trainers and teachers than rule makers and dictators, the authors explain. To make this new human+machine synergy succeed, the authors recommend organisational changes based on their MELDS framework: mindset, experimentation, leadership, data, and skills.
II. Business impacts of AI
The four chapters in Part II of the book cover the impact of AI in a range of sectors, along with case profiles and examples of startups with AI capabilities (see my summary in Table 2 below).
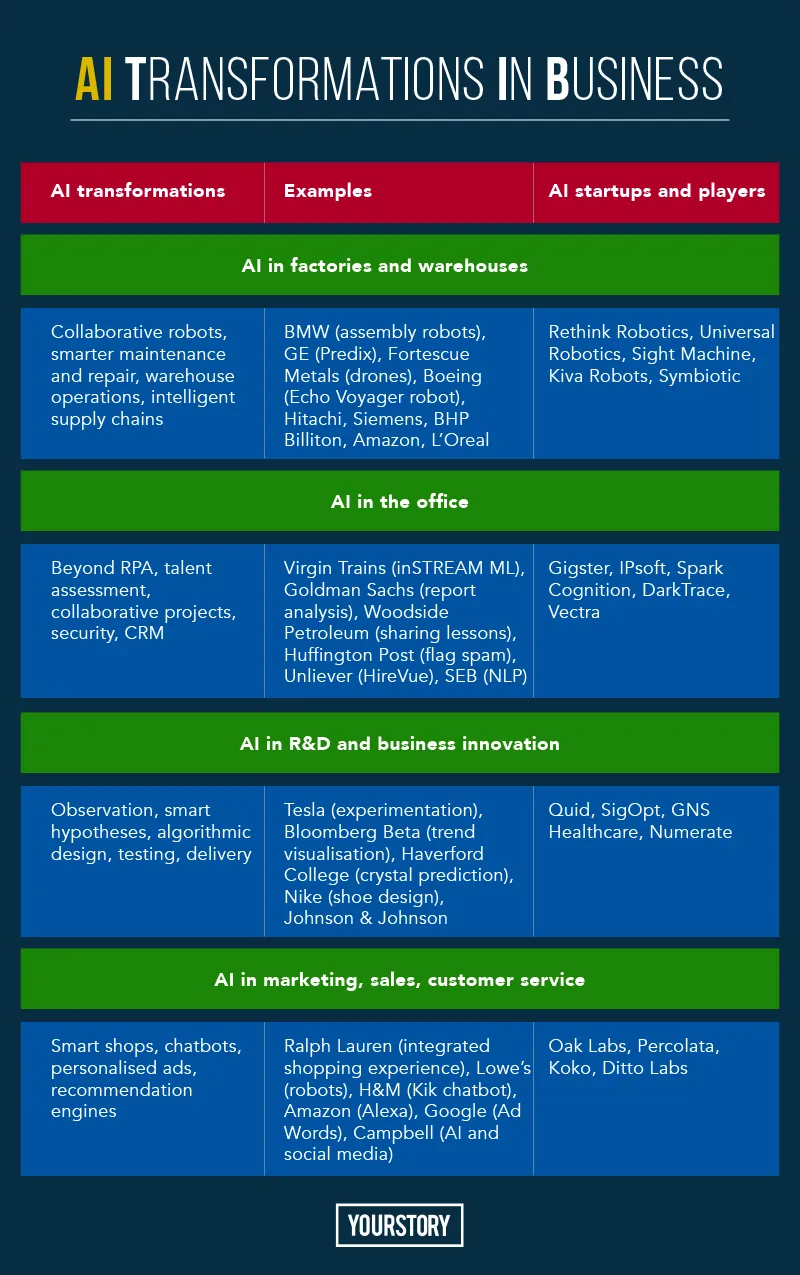
In factories, the early era of bulky, unintelligent and even potentially dangerous robots is being replaced by smaller, smarter and more flexible robots that can work in fluid human+machine teams. Robots can go beyond executing repetitive tasks in heavy lifting or high temperature settings.
Self-aware robotic arms developed by Fanuc and Preferred Networks use deep reinforcement learning to enable better collaboration with factory workers. The robots also learn from one another (“distributed learning”).
Rethink Robotics uses sensors and algorithms for robots to “feel the way” and adjust along the path. Fraunhofer Institute is working on self-adapting assembly lines in car plants that can reconfigure themselves for new customisable models of cars.
Amazon and L’Oreal use robots and ML in their warehouses. Procter & Gamble aims to trim supply chain costs via AI and IoT. Accenture’s Precision Agriculture Service can draw on fine-grain and longitudinal data along with AI for better decision-making in choice of crop and use of fertilisers. In India, Akshaya Patra uses AI and IoT to drive efficiencies and forecast demand in its mid-day meal programmes for needy students.
Based on “digital twins”, GE’s AI-enabled system Predix can forecast when turbine and engine parts may fail, and thus carry out predictive maintenance. New kinds of products can developed, and data can be harnessed across wind turbine farms.
In corporate tasks, AI can help free up time for activities that call for more experience, expertise and judgement. AI can go beyond robotic process automation (RPA) and learn from observation by “watching over the shoulders of employees," according to the authors. AI has helped banks detect suspicious patterns that could imply money-laundering activities. AI can detect patterns in IT security threats, and cumulatively improve defensive mechanisms.
For example, Virgin Trains has an ML platform called inSTREAM that reads, sorts, and routes complaints, thus decreasing manual work by 85 percent. Complaints can now be handled not just from the website but also via email and social media.
Unilever uses AI application HireVue to analyse applicant videos, and has reduced the amount of time to hire employees from four months to four weeks. The time spent by recruiters on reviewing applications reduced by 75 percent.
Gigster puts together teams of developers for software projects, and uses AI to estimate time needs, choose teams, generate quotes, and manage the project. It can also spot potential hiccups along the way.
Swedish bank SEB first tested its virtual assistant Amelia (built by IPsoft, and later renamed Aida) as an internal IT help-desk agent, and then used it for natural language customer communications.
In R&D, AI can help reduce cost, time and tedium by augmenting researchers’ intuition, ability to test theories, and speeding up the product-design cycle. “Where funding a mass-market hit was once a company’s primary driver, ultra-customisation is becoming an increasingly critical - and economically feasible – approach,” the authors explain.
“While human researchers are very good at creative insights, machines are unequivocally better at data organisation and presentation, especially when data volume becomes unwieldy,” the authors observe.
For example, Quint uses AI to analyse large bodies of text data and unearth clusters, similarities, and connections of different strengths. Nike uses AI to design and test better spikes for shoes of sprinters.
GNS healthcare uses AI for precision medicine: finding correlations between drug combinations in seniors that are “hidden in plain sight”. Numerate uses AI to speed up testing of pharmaceutical drug molecules.
IntelligentX Brewing Company uses AI to translate customer feedback for designing better beer. Lenovo uses text mining to gather customer insights for service and product improvement. Johnson & Johnson is training IBM Watson to rapidly analyse scientific literature on drug discovery.
In front-office functions, AI can help create customer-aware shops, eg. Ralph Lauren’s “connected fitting room,” and Percolata’s tools for reducing overstaffing and understaffing of retail stores at different times.
Coke uses Salesforce.com’s Einstein capability to manage stocking of cooler cabinets and predict seasonal variations. The Kraft Phone Assistant suggests different recipes of the day, and refines recommendations by store location and household size. Campbell Soup has worked with Ditto Labs to make sense of customer chatter on social media, for products like its V8 brand.
The startup 6sense helps salespeople target emails more effectively, based on social media data. MIT startup Koko even offers “empathy as a service” to voice and messaging platforms by using ML to gauge user emotional states.
Amazon’s Alexa has now become “the face of the brand”. In earlier times, brands leveraged mascots like Tony the Tiger (cereal) and the Michelin Man (tyres). “These days, brand anthropomorphism extends to conversational AI bots as well,” the authors observe. Bots can also scale better, and learn from interactions with billions of people.
III. Human + machine synergy
The material becomes more interesting in the four chapters of Part III in the book, on synergy between human and machine that builds on the complementary strengths of each actor. I have summarised the four categories of processes in Table 3 below.
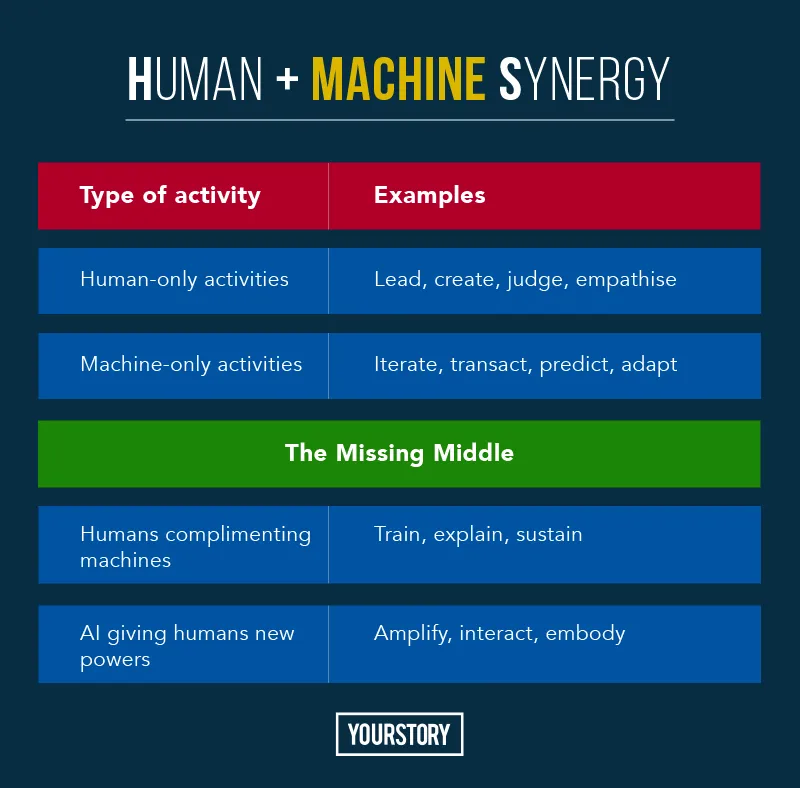
The human-machine dynamic of mutual augmentation positions them as allies, not adversaries or rivals. “Humans can thrive in situations where there is little or no data, whereas machines excel in situations where there is lots of data,” the authors observe.
For example, Rio Tinto remotely manages mines from a command centre with engineers, data analysts, and remote operators. Stitch Fix offers personalised shopping via a combination of ML analysis and human stylists.
Humans need to train physical robots and AI software in mimicking human behaviour, eg., human-cobot combinations for factories, and customer chatbots for sales service. Such “humaneness training” can cover empathy, sarcasm, compassion, humour, conversational skills, and even overall personality and worldview of AI. Data hygienists will play a key role in ensuring quality unbiased data for such scenarios.
Startups in this space include Koko and Init.ai. Mighty AI even uses crowdsourcing to help train systems in visual recognition, eg. mountains in photographs.
Explainers need to make sense of the machine’s workings for stakeholders not familiar with the workings and logic of AI algorithms. Interesting roles in this regard include algorithm forensics analyst, transparency analyst, and explainability strategist.
Sustainers will ensure compliance of AI, fix bad judgment, and promote or demote AI. This calls for new roles like context designers, AI safety engineers, machine relations managers, automation ethicists, and ethics compliance managers. The authors stress the importance of “responsible AI” in this regard – complying with laws and ethical standards, ensuring overall good, and thinking through unintended consequences.
AI augmentation of human activities includes amplification (Autodesk’s Elbo Chair via generative design; Philips Illumeo tool for radiologists), interaction (IPsoft’s helpdesk agent; Microsoft Cortana), and embodiment (Mercedes-Benz factory robot appendages; Zipline drones for blood delivery). This leads to more engaged workers and new business models, not just productivity gains.
The authors also identify eight new fusion skills for the augmented workplace: rehumanising time (leveraging AI to reduce rote tasks and improve well-being of humans), responsible normalising (increasing confidence in AI), judgmental integration (addressing human concerns), intelligent interrogation (asking smart questions for deeper insights), bot-based empowerment (capability extension), holistic melding (mental models of AI), reciprocal apprenticing (between agents and humans), and relentless imagining (creating new business models).
IV. The road ahead
To tap the human+machine advantage, companies need to reimagine and envision new opportunities for co-creation; this calls for curiosity as well as patience. Good data is the fuel for AI, and companies must ensure they have useful, high-quality, and unbiased data. Firms should have a data supply-chain officer in this regard.
A culture of experimentation and willingness to learn from failure is needed for this “controlled chaos,” as seen in Amazon and Walmart’s incubator Store No.8. “After all, in science, an experiment that doesn’t support the hypothesis isn’t called a failure. It’s called data,” the authors rightfully observe.
Leadership should create guardrails and protective cushion for testing AI, use human checkpoints, and address broader legal and cultural issues. “When humans and machines are allowed to do what each does best, the result is a virtuous cycle of enhanced work,” the authors sum up.
It is time now to embrace the exciting new world of human and machine. “Never before in history have our tools been so responsive to us, and we to our tools,” the authors sign off.