Future of Work 2020: Haptik co-founder decodes role of product manager in AI
Haptik's Swapan Rajdev says product managers should own acquisition and sanity of data because AI is only as good as the data.
“In the time to come, you will see a lot of job descriptions calling out specifically for Product Managers (PM) - Artificial Intelligence and not just Product Managers because there is a difference between traditional product management with regard to software versus AI,” said Swapan Rajdev, Co-founder & CTO, , while delivering a talk on ‘Product management for AI products’.”
In his 20-minute talk on Day 1 of the Product Track at the third edition of Future of Work, Swapan gave an overview of aspects like what does a Product Manager in AI do, how does it differ from product management for traditional software, the relevant terminologies and metrics, and what AI Product Managers should understand about data.
At Haptik, Swapan oversees the engineering function, which includes product development, scalability, and future vision from a technology and product perspective, and leads technology for the startup across machine learning, integrations, and platform.
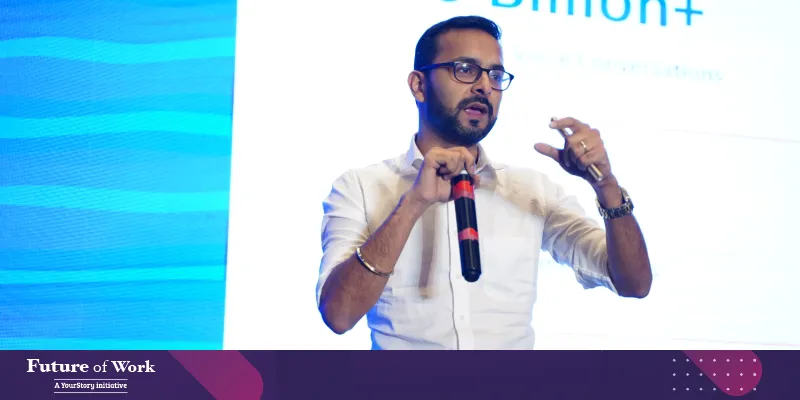
The role of Product Manager in AI
“A PM in AI actually does everything that a PM in software development does and a little bit more,” said Swapan. Explaining what the work for PMs in AI entails, he said, “First and foremost, PMs must define AI. As a PM, you have to define the problem that you have to solve. But with AI, this becomes a little tricky because you are not only looking at how to solve a problem but also how your algorithm can solve it.”
He also said the onus on defining the success criteria also lies with the PMs.
Importance of data
Swapan called for PMs to own the acquisition and sanity of data, “Because AI is only as good as the data.”
He added, “Data becomes as important as the algorithm of the model that you are trying to build.” He highlighted that at the core, the data should reflect user behaviour for the model to be successful.
Swapan also said PMs in AI must be open to creating data either synthetically or manually. Highlighting that the creation of data for Machine Learning is a billion-dollar industry, he pointed out that at Haptik, they partner with companies to create data that reflect user behaviour. He said, “Data is where human intervention in ML is the highest.”
Data bias and how to address it
“It is possible that the person(s) creating data are biased at a certain point, which results in the end result being biased.” Sharing an example of this, he said, “If you are building a speech recognition system and training the data with only people from India, and when you take this global, it will not work because it fails to recognise the difference in accents, which can be problematic for the solution that is being built. Not surprisingly, this is why you see some ML algorithms being racist, sexist, etc.”
Sharing how this problem is commonly addressed, Swapan said, “Let’s take an example where you are building a model with 100 data points. In this scenario, you use 80 percent of the data to build and train your model. You then use the rest 20 percent to validate the model. And, you keep iterating by taking a random 80 percent, building and training the model and then a random 20 percent to validate -- all in a vicious cycle. This will help to weed out a lot of bias. Lastly, you then need a separate set of data which is very different from the set used for developing the ML model. Ideally, a person building the model should not have seen this data set at all. So, it will be for the first time that you are running this data set on the model. To sum up, the test data should be different from development data and within development data, you split it between building and validation.”
Best practices for PMs
Swapan also shared a few best practices for PMs to adhere to. The first was to not just run experiments, but also keep a track of the results. The second was to ensure that the end results stay consistent.
Explaining the reasons, he said, “While the results are probability-based, there should be some consistency. If you get the same image, you need to get the same result/probability. If you get the same image every time but the result is different, it is an indication that something is not right and it becomes harder to explain. So, while the results are probabilistic in nature, they still need to be consistent.” The third best practice is to stay on top of the developments in the space but cautioned PMs from getting caught in building state-of-the-art algorithms for every problem. He said, “While it is very exciting to build state-of-the-art algorithms, every algorithm need not be so. Because, sometimes for real world problems, simple algorithms work best and drive results. The focus should be on solving the actual business problem.”
To sum up, Swapan’s key takeaways for PMs in AI are: To define the problem, own the data, define the success metrics, keep a track of industry developments and most importantly keep it simple.
He said, “If you get all these aspects right, you will be able to say - I can build a successful AI product.”
(Edited by Javed Gaihlot)
A big shout out to our Future of Work 2020 Sponsors: Alibaba Cloud, Larksuite, Vodafone Idea Limited,
, Adobe, , , , , , , Maharashtra State Innovation Society, and GetToWork; and our Knowledge Partner: Ascend Harvard Business Review.