How prescriptive analytics can help ecommerce brands improve efficiency, reduce costs
Prescriptive analytics provides real-time recommendations to brands. It can help brands make informed decisions and achieve better outcomes.
With marketplaces congesting the ecommerce field, brands often rely on data to see what works, what didn’t work, or how consumer behaviour is going to change in the future.
Descriptive and predictive analytics have become quite mainstream now. With access to huge volumes of data, statistical models, and machine learning (ML) algorithms, brands have a fairly good sense of how their business is doing. But the big question that remains to be answered is—what action should we take next?
A brand, on average, is expected to make hundreds of decisions every day. Using intuition or gut feeling to make decisions can be unreliable and even lead to costly mistakes. This is where prescriptive analytics comes into play. Building on the foundations of descriptive and predictive analytics, prescriptive analytics provides real-time recommendations to brands. It can help brands make informed decisions and achieve better outcomes.
Let’s take inventory forecasting for example. Say you are a footwear brand. While planning your inventory, you would need to individually look at each type of footwear (shoes, for example), style (formal, casual, sports), colour, size etc. For each SKU, you would need to look at historical sales data. You would also need to account for seasonal trends. Add to that regional/geographical demand. You need sophisticated machine learning models that can ingest data daily, analyse the trends and patterns over a period of time, and then provide recommendations. If you can optimise your inventory to avoid both overstocking as well as stock-outs, you can make significant savings for your brand.
What we have seen above is just using data. With new advancements, we are already seeing ML models that integrate data pulled via APIs from across marketplaces and other platforms in real-time. So not only are the recommendations given based on store data, but they also factor in the market and competitor data. Now, not only can brands improve efficiencies but also stay competitive.
The other area where prescriptive analytics can play a big role is advertisement. This is perhaps the biggest cost component for any brand.
For each product, whether at a campaign, ad set, or ad level, there are so many variables and unknowns. It becomes even more complex considering the festive season and other peak sale days when one needs to step up advertising. Brands often need to make a lot of decisions in a very short period of time. How brands finetune audiences, budget and bids can massively impact the acquisition costs and ultimately the bottom line. Insights and recommendations from highly trained algorithms can significantly reduce this risk.
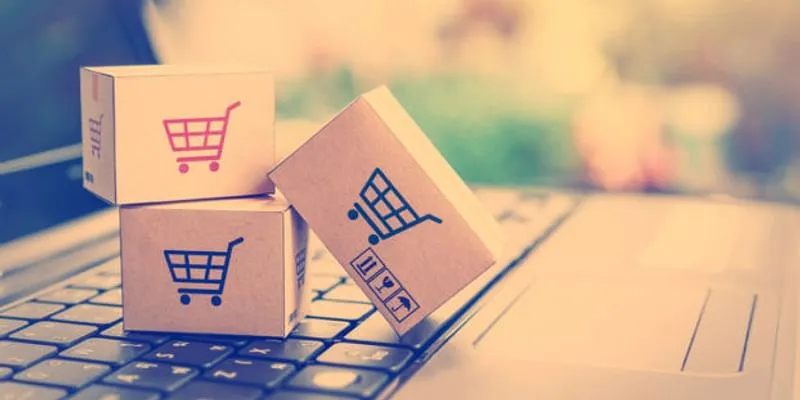
Image Source: Shutterstock
The storefront is yet another area where brands can derive a lot of value. Understanding what content performs well, the quality of listings, product descriptions and images—optimising and improving these can result in better conversions and higher sales. Dynamic pricing, on the storefront, presents another huge opportunity and potential for brands. Based on various signals including date and time of day, product demand, product, comments and reviews etc, ML algorithms can suggest the right price for an SKU. It can also make recommendations based on the brand’s goals—to maximise revenue, order volume, profits or any other metric.
These examples are just the tip of the iceberg. More and more brands have started adopting prescriptive analytics. Finally, when considering how to effectively leverage prescriptive analytics, there are four factors that brands need to keep in mind.
First, is the data itself. The quality of data, its breadth, depth and of course, how timely it is. Data exists in multiple places. The ability to combine data from multiple sources into a single evolving and scalable data model will form the cornerstone for success.
Next is data science. When your business is relatively nascent and data is limited, heuristic models, with rule-based learning, are sufficient. However, as your business grows and you have to deal with large and complex datasets, you will need to lean on more advanced ML models.
Third is automation. The recommendations delivered by the ML models are only useful if they can be executed easily. So, the ability to automate execution across platforms in real-time will be a game changer.
Last, but not least, is strategy. If you want to derive long-term value, then your ML model must work in conjunction with your business goals and strategy. As you start to implement outputs from the models, feedback on success and failure must be fed back into the models. A model is only as good as the input it receives and the more it gets feedback; the more it improves its predictions and recommendations, the better your outcomes will be.
(Prem Bhatia is the CEO and Co-founder of Graas, a company offering Growth-as-a-Service to ecommerce businesses worldwide.)
Edited by Kanishk Singh
(Disclaimer: The views and opinions expressed in this article are those of the author and do not necessarily reflect the views of YourStory.)