Levelling up the data game: Top trends that will redefine growth
The future will demand maximising value through data. The next level of growth will depend on how companies across industries leverage data to build a more robust business and customer engagement strategy.
Today, data has emerged as the foundation of efficient operations. It is empowering organisations across industries to gain a competitive advantage and enabling them to understand their customers better. With the rise of artificial intelligence (AI) and machine learning (ML) and the cloud migration of data, there has been an exponential increase in the big data and analytics market.
With the rise in mobile apps and the Internet of Things (IoT), the big data and analytics market is expected to witness significant growth in the coming years. The size of the big data market in India was valued at Rs 15,724 crore in 2022 and it is expected to reach Rs 25,322 crore by 2027, expanding at a compound annual growth rate (CAGR) of ~10.60% during 2023-2027, as per ResearchAndMarkets.com's report titled "Big Data Market in India 2023".
It would be right to say that data and analytics will no longer remain merely a tool for decision-making but will become a driving force behind innovation, efficiency, and transformation of industries at large.
As the data-driven world is undergoing a profound transformation, here are the four data trends that are likely to shape the future of big data and analytics in the times to come.
Augmented analytics
AI-powered augmented data analytics will empower fintechs to deliver better products for a variety of reasons. AI in data analytics enables quick detection of inconsistencies in data resulting in quicker fact-checking and validation tasks. It also, in turn, helps to build a more robust system that is aversive to a variety of risks.
AI enables democratised access to data. By combining ML and Deep Learning models, a Natural Language Processing system can extract data from vast amounts of raw, unstructured, and unlabeled text and sound data sets and classify and assign a statistical probability to each possible meaning of those elements, making it easier even for inexperienced users to gain access to complex datasets.
Also, with the help of ML algorithms, it can help make sense of massive amounts of information quickly and accurately. This is key in today’s times when companies have access to a whole bunch of data including behavioural data, referral data, intentions data, location data, as well as data on what customers say about the brand on public forums. This can enable companies to build a more focused strategy for customers that can drive engagement and lead to better conversion.
Interestingly, AI is expected to drive the next level of growth in data analytics. As per a report by Onyxdata, the AI market is expected to reach $190.61 billion by 2025, growing at a CAGR of 36.62% from 2020 to 2025.
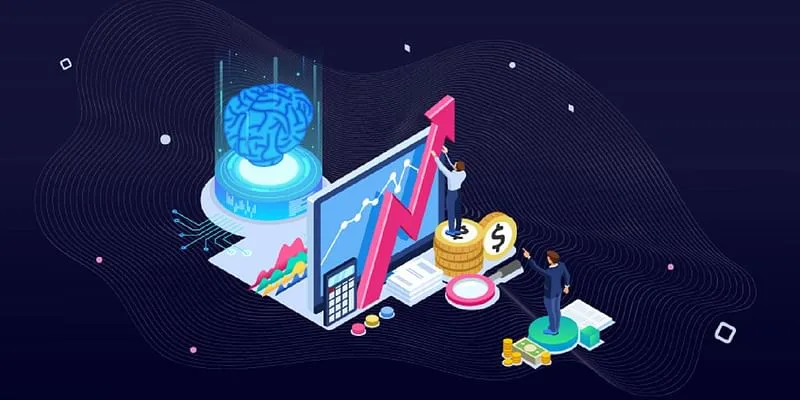
Edge analytics
Edge analytics involves data analysis in real-time at a sensor, a network switch, or at other devices instead of a centralised data store. As the IoT model of connected devices becomes more mainstream, the process of collecting, analysing, and creating actionable insights in real-time and directly from the IoT devices is also becoming popular.
There are numerous benefits of using edge analytics, including saving processing and uploading time. Also, it helps in reduction in operational costs, minimisation of needed bandwidth, as well as reduction in resources spent, leading to significant financial savings. It also helps preserve privacy as sensitive information is pre-processed on-site and not uploaded to the cloud for processing.
Also, edge analytics reduces latency, thereby making it crucial for sectors where data insights are required at the edge, including manufacturing, healthcare, retail, energy, smart cities, transportation, and logistics. The growing need for real-time insights and increased focus on security and privacy are the factors driving the growth of edge analytics.
Data observability
Data observability can be simply defined as the practice of monitoring, managing, and maintaining data in a way that ensures its quality, availability, reliability and performance across various processes, systems, and pipelines within an organisation. When a company has a true understanding of the health and performance of the data within its systems, it can put up the best defence against bad data getting through.
Some of the key elements of data observability include data quality monitoring, data lineage and traceability, data performance monitoring, data security and compliance, alerting and notifications, data governance, metadata management, data documentation, data auditing and logging, ML observability, collaboration and communication, and continuous improvement.
By being data observant, an organisation can derive several benefits such as faster troubleshooting and higher data quality. Organisations can also discover potential revenue-generating opportunities by unlocking new insights and identifying trends, through observability tools and platforms. In highly regulated industries such as finance, healthcare, and telecommunications, data observability helps improve compliance by ensuring that data meets the necessary standards for accuracy, consistency, and security.
In today’s world of ecommerce and digital, one of the biggest consequences of data downtime for an organisation that relies heavily on online sales and services is revenue loss. Hence, such organisations must focus on becoming data-observant, thereby ensuring continuous monitoring of the data pipelines to have complete, accurate, and timely delivery of data at all times, in turn, safeguarding the reputation of a brand.
There has been a huge increase in demand for observability tools and platforms over the last few years. This is only expected to grow in the coming years, as more and more businesses understand the importance of data observability and invest in adding these tools to their kitty.
Data security and blockchain
A lot has been said about the benefits of blockchain and how it can play an important role in driving data and transaction security. The future will see larger usage and acceptance of blockchain technology in safeguarding the reliability and protection of data. Additionally, it can be utilised for purposes of data sharing, while maintaining a record of activities.
Generative AI
Generative AI, or Gen AI, is the buzzword today. An increasing number of professionals are leveraging Gen AI capabilities to generate content, data, graphics, videos etc.
From a data perspective, Gen AI is useful as it can easily pick up patterns and structures from existing data and, in turn, use that knowledge to generate new content. There are challenges with generative AI, especially around privacy infringement and information misuse. As we speak, experts and policymakers are trying to strike a common ground that balances innovation and effective regulation in AI.
(Ritesh Mohan Srivastava is the Chief Data Scientist at BharatPe.)
Edited by Kanishk Singh
(Disclaimer: The views and opinions expressed in this article are those of the author and do not necessarily reflect the views of YourStory.)