Towards inclusive innovation: How startups can champion responsible AI
Startups should leverage AI that’s unbiased and responsible to build trust, promote sustainable and inclusive growth, and ultimately make the organisation more profitable.
AI systems are not neutral. Rather, they are socio-technical artefacts that result from the data, parameters and choices that developers employ to construct them. Data mirrors our society, and the inputs used in algorithms influence the outcomes we achieve.
Consequently, it is not unexpected that AI outputs may reproduce the realities of our world. Nevertheless, this should urge us to contemplate the ramifications of converting the world as it currently exists into software.
For example, if women typically receive lower wages than men, algorithms used to assign credit may inadvertently reinforce this extant inequality. Improving this equality complicates the situation because of the hidden mechanism of AI.
AI needs more women
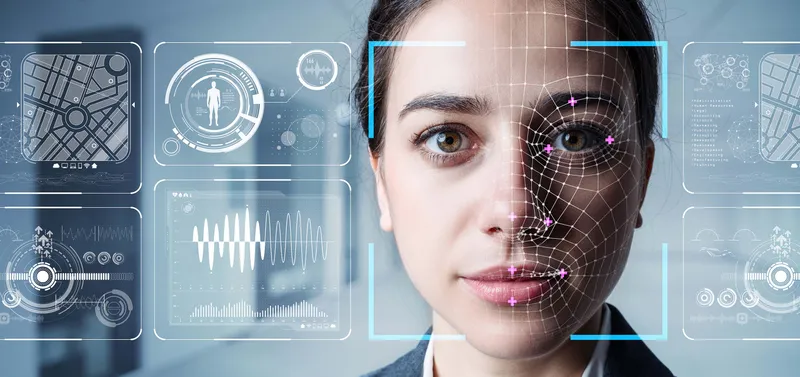
Women are vital in AI to ensure we build AI that benefits everyone and contributes to creating growth.
Since AI artefacts are not merely technical but also social, it implies that they have a profound impact on our society. Therefore, it is essential to ensure that AI reflects the diversity of our society.
This necessitates the participation of more women in AI, not only in coding but also in creating AI products that promote good and responsible use of AI. This is where startups play a role.
The ‘Women in India’s Startup Ecosystem Report’ (WISER) shows that startups led by women have thrived and now account for 18% of the country’s growing startup scene.
Women are a vital link in technology, especially in AI, to ensure that we build AI that benefits everyone and contributes to creating growth.
Why should we worry about inclusion?
AI algorithms are increasingly deployed in our social systems, and some of them may cause harm. And that harm may impact the trust people have in technology. Trust is important if we want people to leverage AI, thus growing our economies.
For example, AI is widely used in human resources (HR) where it can add enormous precision, efficiency and depth of analysis in selecting the right employees for a business. This is something we should embrace.
Nevertheless, HR is also an area where AI can have a detrimental impact on people’s lives as bias may produce discriminatory outputs and leave people out of opportunities, promotions and rewards.
Recently, the US Equal Employment Opportunity Commission settled its first lawsuit concerning AI bias in hiring when one user who was screened out submitted the same application but tweaked the birthdate to make themselves younger. With this change, they landed an interview. However, it would not be prudent to fear AI for these pitfalls; instead they must be used as learning platforms to improve existing algorithms.
Startups, which are known for their agility, must not rush to use or promote undeveloped tools. Instead, they should recognise that leveraging AI that’s unbiased and responsible is the ethical and legally necessary route. This will build trust, promote sustainable and inclusive growth, and ultimately make the organisation more profitable.
How to avoid AI discrimination
The first step to combat bias turning into discrimination is to acknowledge that bias is not just a technical problem but is also a social one. We cannot simply rely on technology to fix bias, because bias is rooted in the values, norms and structures of our societies. Therefore, we need to examine and challenge the underlying biases that shape our worldviews and behaviour.
This is not a pessimistic view but a hopeful one, because it means that AI and Big Data analytics can also help us identify and address these biases, and even inspire us to rethink our legal and ethical frameworks to promote equity and justice.
The second step is to implement effective governance mechanisms at different levels and stages of AI development and deployment. Bias can emerge in various ways throughout the AI lifecycle—from data collection, processing and algorithm design to testing, system deployment, and evaluation.
Here is an overview of the main sources and types of bias in AI:
- Data bias can result from incomplete, inaccurate, outdated or skewed data, which can lead to unfair or inaccurate outcomes for certain groups or individuals.
- Algorithm bias can result from flawed or biased logic, hidden or explicit values, or unintended or malicious manipulation, which can lead to discriminatory/harmful actions and recommendations by the system.
- Interaction bias can result from miscommunication, misunderstanding, or misuse of the system, which can lead to distorted/misleading information or outcomes for the system or its users.
- Societal bias can result from the lack of transparency, accountability, or oversight of the system, which can lead to erosion of trust, privacy, or human rights, or exacerbation of existing inequality and injustice.
Understanding these different kinds of bias is essential to understand how every single step of the AI lifecycle can introduce mitigating measures.
Startups drive innovation, making them ideal champions of responsible AI development. AI tools can be refined in startup environments with diverse perspectives, thus mitigating bias and ensuring inclusivity and ethical use.
The author is Vice President and Global Chief Privacy and AI Governance Officer at Wipro Ltd.
Edited by Swetha Kannan
(Disclaimer: The views and opinions expressed in this article are those of the author and do not necessarily reflect the views of YourStory.)