Why building the right data science team for your business is crucial
Algorithms don't change the business, only teams that understand the business outcomes with data will be able to scale up, experts say.
According to SAP, only 17 percent of the global businesses understand digital transformation. But going digital is not just about creating apps or websites; it is about business outcomes and using digital avenues to win clients and drive sales. It is all about using data for outcomes. The question then is, what do we need to embark on this journey (combining legacy and digital) to understand everything from employee to client relations and performance of marketing channels?
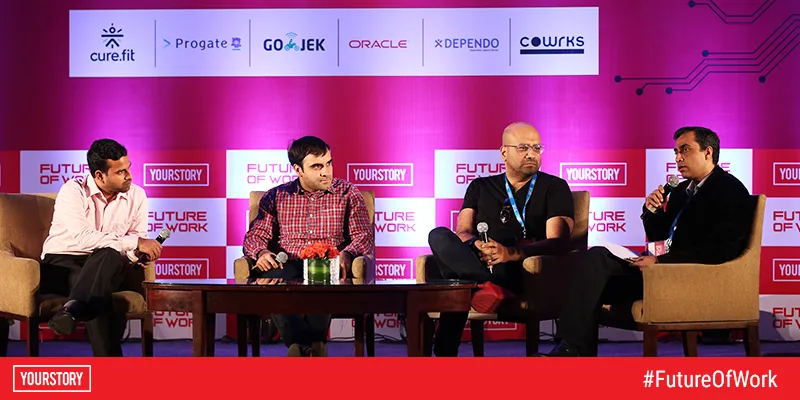
Experts at YourStory’s Future of Work event on Saturday explained why having the right data science team determines the future of a company.
Ashish Gupta, VP Engineering, Rubrik said, “We have to look at the right means to solve the end when we look at artificial intelligence (AI). Engineers have to understand what it means to solve a problem.”
Avi Patchava, VP, Data Sciences, ML and AI, InMobi spoke about the difficulty in deciding what works with a digital team. "On one hand, you have conventional banks that want next-generation credit modelling. Will machine learning (ML) help them? Yes, it will. Then, there are companies like Deep Mind, which are building algorithms that can be generalised for multiple scenarios. The algorithms have known to beat gamers and, in the future, these algorithms can solve several things.”
Startups need to tell the difference between AI and ML. Otherwise, it becomes very difficult to build a business case. One has to figure out the unit economics to make data tech and AI companies work. One needs domain expertise and articulation when they look at data. Avi explained,
I have seen the world of consulting and selling concepts versus building things to solve organisational problems. I shifted because I wanted to see challenging problems. You can't just pick an algorithm and think of changing the business. You need a lot of experimentation with the algorithm and understanding. But that's harder to come by.
Companies need data to be structured when they put together a data team. “Marry data from Excel and combine ML models, compare them and make prudent choices,” said Ashish.
To be an AI engineer, you need good mathematical and statistical skills. Any company with an outsourced champion will not be able to win.
So how should a company look at data science teams? The panellists gave a handy list:
- Formulate roles that translate the higher level business objectives at a technology. The business should be able to predict the right level of inventory or the right level of pricing. It is important to make this translation in the context of AI.
- Data science teams should have multitudes of libraries of algorithms and have the skills to match the algorithm to meet the problem. Understanding the context to a business outcome becomes important.
- Data engineers should iterate fast with volumes of data and figure out what works. For example, Flipkart had thousands of product categories and the data team created different data models for each of them.
- There has to be a hybrid of centralised specialists in statistics and mathematics working with engineers who do AI and ML modelling.
- Train engineers to understand data science problems.
“No one knows what good talent is in the space. People are throwing algorithms in their CVs and a CEO gets confused with these CVs. It is difficult to get good talent. I need people to look at solutions rather than talk their way through them. We test them for technical capabilities and depth in their field,” Avi explained.
The future is in working with people who are willing to learn and build a company's vision. One should not get fooled by claims. Test your data scientists and ML/AI engineers before you hire them.
A big shout out to all our sponsors - CureFit, Oracle, Progate, Gojek, Dependo and Cowrks.