With AI taking over, is it time for human intervention with audit?
Universities like Oxford have realised that as the human-machine partnership becomes deeper, machines will make decisions that may not necessarily be ‘human’ after all.
Last year, Facebook ran an experiment with two Artificial Intelligence-based computers – the experiment was simple - computers were taught the English language so they could communicate among themselves on ‘trading’ books, balls, and hats.
No rules were set, and the computers were free to modify and even set the rules of communication to ease and aid understanding. What happened then was beyond anyone’s wildest imagination or calculations. The computers, as expected, were talking and even trading. Only, they were developing an entirely new English language that was alien to the humans.
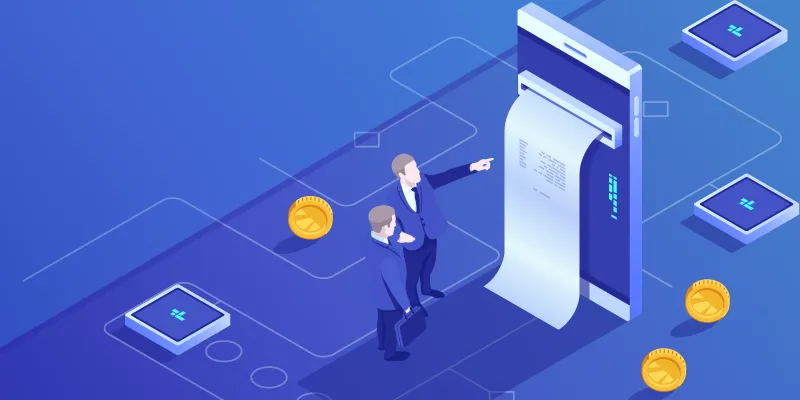
The Facebook team, satisfied that the two computers were communicating, and even formulating their own language to do so, soon pulled the plug on the project. Or so it said.
Then started the conspiracy theories.
One says the project was scrapped as the ability of the computers to communicate among themselves in a language that humans could not understand frightened scientists as, on a large scale, it could pose a potential threat to humanity itself.
While many other theories made the rounds, the scientific community was slowly coming to the realisation that AI was needed, and more importantly, it had to be audited.
“This is an era of human-machine partnerships. Let me tell you this - whenever there is a new technology, people get scared. Take fire or the wheel. There were good things and bad things that came out of those,” Michael Dell, CEO of Dell Technologies, told YourStory at the Dell Technologies conference.
Across technology companies such as Oracle, Dell Technologies, Google and AWS, there is no doubt that AI is here to stay.
These large companies are clear about a ‘narrow’ AI strategy that involves making recommendations, predictions, and figuring out patterns on a real-time basis. There, however, is an entire breed of startups, both in India and abroad, that have been inspired to set up broader AI narratives.
The mad rush towards AI is reminiscent of the music industry after The Beatles’ debut. Record companies commercialised ‘rhythm and blues’ music, and an entire generation of me-too brands – some good, most terrible. Finally, the product lost steam and was pretty much dead by the 1980s.
Unfortunately, AI is unlike music, and any commercialisation will not be without consequences well into the future.
More importantly, since public and enterprise data is what makes the raw material for any AI function, AI auditing is very important to understand how data is inferred, interpreted, and even used and consumed.
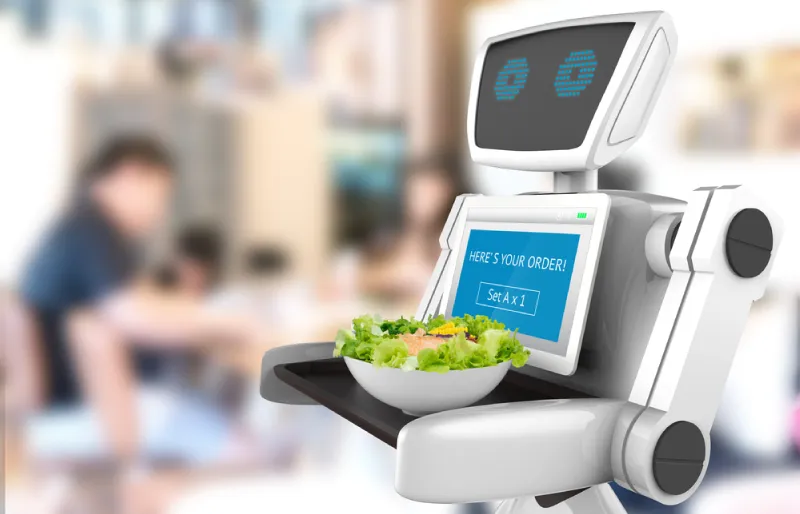
Take an example here – food may be your absolute joy and past orders may prompt AI systems to push particular products for you, be it while ordering food or shopping online. Now what if these recommendations do not go with changing and evolving dietary patterns and choices? On the other hand, what if a company sees that AI introduced a pricing method that did nothing to increase sales of a particular service, nor did it have a positive impact on the bottom-line.
Who is responsible for AI’s decisions?
“This is a very serious subject pursued in academic and corporate research functions. Organisations have to prove to regulators all over the world that their algorithm’s approval or disapproval, of transaction and recommendations, has not resulted in bias or unknowns that can cause any harm to any party involved in the transaction,” says Thomas Kurien, President of Product Development at Oracle.
The Institute of Internal Auditors has outlined why it is important to audit AI.
- The Human Factor
The Human Factor component, which includes ethics and the Black Box elements, addresses the risk of human error compromising the ability of AI to deliver expected results.
- Ethics
Algorithms developed by humans that include human error and biases, both intentional and unintentional, impact the performance of the algorithm. The human factor component considers whether the risk of unintended human biases factored into an AI design is identified and managed, and whether AI has been effectively tested to ensure results reflect the original objective. AI technologies can be transparent given the complexity involved, and AI output is being used legally, ethically, and responsibly.
Algorithm Bias
According to a recent McKinsey & Company report, companies are quick to apply Machine Learning to business decision-making. The programmes set complex algorithms to work on large, frequently-refreshed data sets. However, algorithmic bias is a risky business because it can compromise the very purpose of Machine Learning if overlooked, and left unchecked. (See Controlling machine-learning algorithms and their biases).
Professors at Oxford University argue that robotics firms should follow the examples and regulations set by the aviation industry - bringing in the black box and cockpit voice recorders to investigate plane crashes so that crucial safety lessons are learned after tragic events.
If installed in a robot, an ethical black box can record the robot’s decisions, the basis for making them, its movements, and information from sensors such as cameras, microphones, and rangefinders.
According to information available with Oxford University, it will be a part of a project called the The Realising Accountable Intelligent Systems (RAInS), a multi-disciplinary initiative run by three universities. This project involves a £1.1 million funding from the Engineering and Physical Sciences Research Council (EPSRC), and the project is a direct response to EPSRC's call for research on understanding of Trust, Identity, Privacy and Security (TIPS) issues in the digital economy.
Where do Indian startups stand in this?
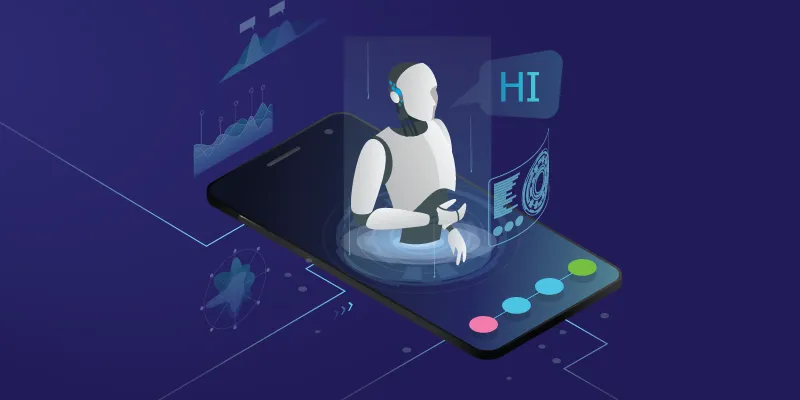
Just like the implementation of the General Data Protection Rights (GDPR) had caught many Indian startups serving Europe unaware, AI auditing too could have startups struggling as they keep busy acquiring customers, but not necessarily following the trajectory the application would take.
Soon, when regulators sit up and ask startups to prove their algorithms are reasonable in making decisions, people will be stuck when it comes to answering those questions, says Sridhar Marri, founder of Senseforth, whose algorithms are used in the healthcare industry in the US.
Regulatory bodies like Securities and Exchange Board of India, Reserve Bank of India and Insurance Regulatory and Development Authority, and several others, will heavily depend on Justice B N Srikrishna’s upcoming white paper on data protection, which may eventually become a law.
Then, most see a scramble among both startups and corporations to figure who is actually liable for the AI.
“We absolutely need auditability and explainability,” says K M Madhusudan, CTO of Mindtree. He adds that there are two aspects to this - one is for serious enterprise-level AI adoption, for which technologists must ensure AI can explain why it made a particular decision. The second is to ensure that it is not biased.
Explainability, thus, is crucial. For example, when working with an airline on a price determination algorithm based on probabilistic and statistical models, every seat on a flight is sold at a different price and, if unsold, accounts for a loss to the company. Every airline needs more than 75 percent occupancy for any flight to be profitable.
Now, if the machine decides the price and the flight still runs at a loss, a business leader should be able to ask the machine for an explanation as to why it determined a price that led to a loss. This ‘accountability’ of machines is not existent today.
Machines take decisions today, but there is no mechanism to question them and that’s what IT companies want to make sure businesses have complete control over.
Today, we are all mostly in the realm of conversational AI, where a machine can infer sales by region, and tell you on a real time basis where losses have been recorded.
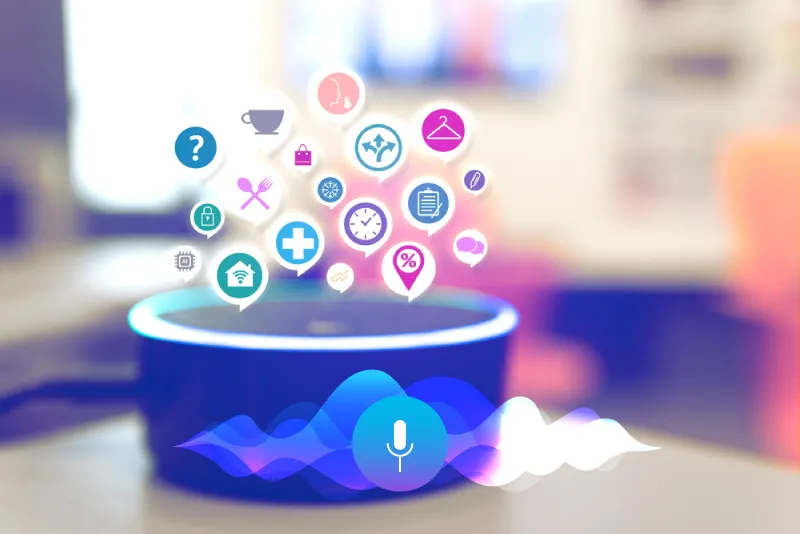
Also, when AI makes recommendations, as seen in cases such as Alexa or a Google Voice Assistant, one needs to know what they are signing up for.
Yes, AI can be helpful in discovering information, but what if it makes decisions, on our behalf that are not in our best interests?
That’s the next best business opportunity - to have AI police itself. Until then, we are all one big data set, and already sold on AI.