Swiggy's tech head on how the foodtech startup is using AI to go beyond food deliveries
In an interview with YourStory, Dale Vaz, Swiggy’s engineering and tech head, explains how the startup unicorn is using an AI-first approach to think beyond food delivery and become a platform that serves as a convenience provider.
Swiggy still wants to serve you great food but wishes to go beyond and help get other products and services at your doorstep as well. Its technology platform processes over 40 billion messages every day and this is just the start for this on-demand services startup.
The foodtech startup has unveiled a bold vision of transforming itself into an artificial intelligence-first product as it has started to leverage this tech to add more services that go beyond food delivery.
Dale Vaz, Head of Engineering & Data Science, Swiggy, in a conversation with YourStory, spoke at length on the unicorn’s technology roadmap.
Edited excerpts:
YourStory: How is Swiggy scaling up its technology infrastructure to keep pace with the startup’s expanding operations?
Dale Vaz: In the last one year, we have expanded from 17 cities to 245 locations. Our monthly active driver fleet, which was at 40,000 a year back, has now grown to more than 200,000. Order volumes have gone up by 300 percent. There has been a lot of hard work that has gone into this from a business perspective. What we do is to support this entire piece.
If you look at the Swiggy engine, it is like a three-way marketplace where we have got consumers, driver as an on-demand worker, and the restaurant partner or a grocery outlet. At Swiggy, we are trying to balance this three-way marketplace. We have the consumer demand but there are challenges from the driver and restaurant perspective.
I need to figure out how to balance demand and supply equation across these three entities. This is something that is unique about Swiggy where it is a constant dynamic engineering challenge that is three-fold.
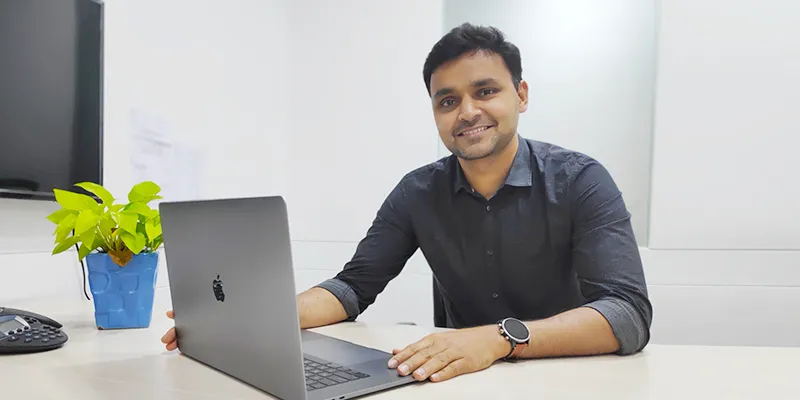
Dale Vaz, Head of Engineering & Data Science, Swiggy
When Swiggy started out, it was all about a food tech stack, and everything was built in-house. However, as we scaled it with an exponential number of customers, there is now a larger vision of being a convenience provider where the platform serves needs beyond food to any kind of product or service.
The first big tech challenge was moving from a food tech stack to a platform in the hyperlocal category. Today, even though we support food, we should able to do the same for other things like buying medicines, grocery, pet food, etc.
We have done that with the launch of Swiggy Stores in Gurugram where consumers can access 3,000-plus stores and order anything. We promise to deliver them in 30 minutes. From an underlying technology perspective, we did not go and rebuild our technology as we only architected to create a multi-category platform.
It is a similar thing with our Swiggy Daily subscription programme, which is a totally different use case. From a business perspective, as we extend to more use cases, the focus is to enable all this by leveraging and building core tech capability. The vision today is that we are supporting Swiggy but tomorrow we should be able to support any other product and business.
The second area of focus for tech is transforming ourselves into an AI-first product. Any company, when it starts, has great founders, a tech backbone and certain good intuition. Once you start to scale, one has to start rethinking some human intuitions and replace them with system-based intelligence.
With our AI-first approach, we are looking at how can we replace and rethink Swiggy products, and replace some of our hard-coded system assumptions with intelligent AI-based systems. We are doing this across the board to look at how Swiggy can become an AI-first product.
The third focus is on scalability and availability. We need to keep pace with the growth of the business but we have a unique situation. As we double our volumes every few months, the kind of architecture that we have now may not make sense 18 months from now, so we have to keep rethinking architectural principles we have built. We are working with a lot of partners to look at how we can rethink specific innovations out there that we can apply to Swiggy.
These are three core strategic directions set for the team and we are constantly working at it.
YS: So, Swiggy has built the fundamental blocks of its technology platform?
DV: Yes, with our Swiggy Stores programme we have demonstrated that we are able to build non-food categories. As we scale, we will uncover new use cases.
The journey has just started from food tech to local commerce platform, and it is good validation that our platform is capable of supporting multiple types of products. Though I would say it is still Day Zero.
YS: Can you tell us more about Swiggy’s AI strategy?
DV: The first thing that we have done is build a world-class AI team. Last year, we hired multiple PhDs from international universities, and tenured people from places like IBM and GE Research as senior scientists.
We have also built a dedicated applied research team that is separate from the data science team. This team focusses on areas around voice, vision, and natural language processing (NLP). The thinking is that there is a team that will look at immediate problems while the applied research will look at how to solve for the next set of 100 million customers.
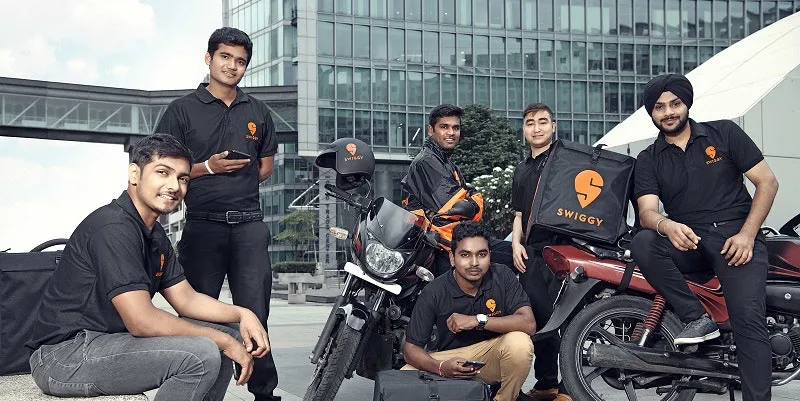
The second step is building data infrastructure. Over the course of last year, we have been working on the Swiggy data platform, which houses all the data that flows through our network. It is a pretty massive system processing terabyte of data: 40 billion messages per day from customers, drivers, and restaurants.
We are crunching all this data and building all the machine learning (ML) models. We have also partnered to get third-party data. For example, we have partnered with a startup for information on recipes.
The third step is having the Swiggy AI stack and building intelligence models on top of that. This will be split into foundational AI capabilities and applications. The foundational AI capabilities could be an area like computer vision, where there are lots of use cases, like automatically taking a picture of a restaurant menu and converting it into a file.
The fourth piece is looking at how we can also build long-term capability by partnering with external research institutes and industries. This will help build a differentiated experience for the long term. We have partnered with universities to enable this kind of research under a grant programme where Swiggy can fund that research.
The university academics and Swiggy scientists can work together to build innovative models. Similarly, we have partnered with startups and industry leaders like Amazon Web Services.
The fifth piece is democratising AI across the organisation so that everybody can build models around it. We launched this programme called ‘AI for All’ where we are training our teams, analysts, and even business leaders. Eventually, our vision is to make AI a part of our culture where we have built the capability and knowledge in the organisation.
YS: How does Swiggy’s tech ensure that the delivery gets done on time?
DV: The entire challenge is real-time decisions or optimisation across the product. Even as the consumers are deciding, the drivers are moving around and restaurants may be also busy with their own set of patrons. At every single moment, there are different variables.
The first thing we do is promise the customer that we will deliver within a stipulated time. There are intelligence models built around the customers, such as what items they would prefer, at what time, etc. There is intelligence built around the catalogue, like the menu items, ability to tag the dishes, etc.
All these are done through our AI model. For example, people refer to chicken in different ways in India, like murgh or Koli (in Kannada). The challenge is how do we make the platform understand that all this is same.
From a delivery partner availability point of view, when there is an order to fulfil, their location pings every few seconds on a real-time basis. All the data we get is on a real-time basis so that we know which driver is available to deliver so that we can tell the customer that we can actually service the order.
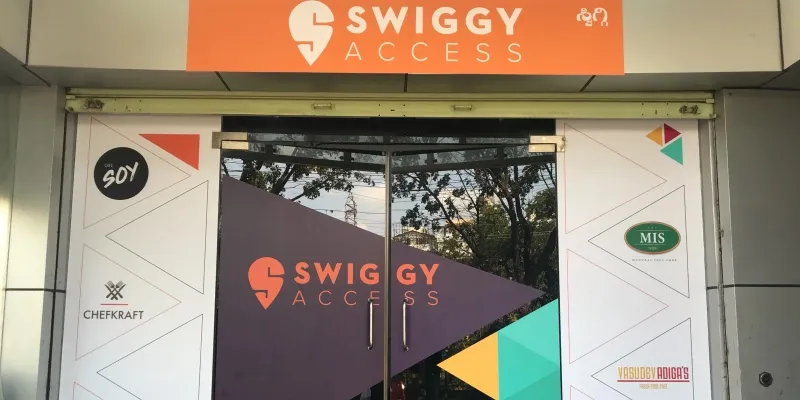
Swiggy Access, Kalyan Nagar Centre
The other input is time and space. The unique thing about on-demand is that your own needs will change according to the time and location. Intuitively, the platform understands during mornings that it is breakfast time so we also need to change our restaurants. We have built the intelligence on our side to understand these requirements. If it is a Sunday afternoon, we show more experiential restaurants.
We have built these models to process all this data and come up with the best possible outcome. The biggest question is not about which delivery partner can be assigned for the delivery; the harder challenge is to assign it to the most optimal partner in a cost-effective way with good consumer experience. That is Swiggy’s secret sauce to balance competing needs.
From the delivery partner side, we need to understand whether the individual is a new person or has a better chance of delivering on time. Similarly, restaurants too have their own challenges, especially surrounding preparation time.
A pizza may take 20 minutes to prepare but lasagna will need 30 minutes. We have built systems that take into account how much time these restaurants take to prepare these dishes. Taking all this account, we run this matchmaking algorithm in real-time every few seconds across India. And all this is automated.
YS: What are the kind of insights you receive from data?
DV: This is a very spiky business, depending on the time of the day. We visualise a lot on data. For example, once the delivery partner reaches the restaurant, they have to mark that they have arrived but when we started visualising, we realised it was different.
This is because they mark ‘arrived’ before reaching the restaurant so that they would get their orders on time. So, we have built more accurate GPS-based location system so that we know that they are physically present at a particular place.
Another data-driven insight is on the food quality, especially when sometimes a customer orders a dish but gets something else. To solve this, we have built a rig-like box that has got a camera on it. This takes the picture of the dish to identify the correct one. This is a deep learning computer vision model that we have deployed.
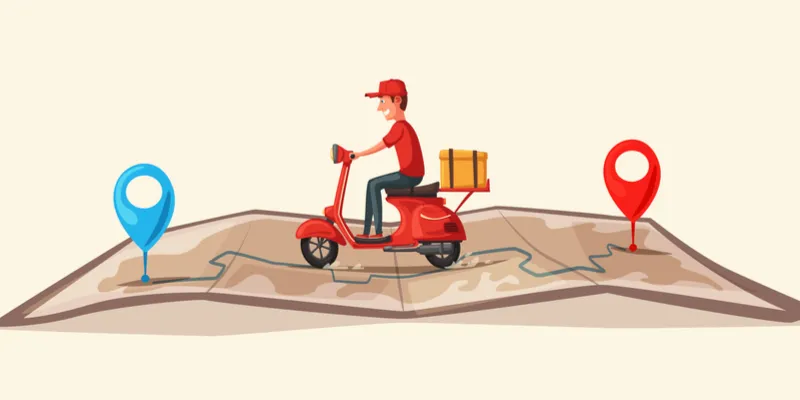
YS: As Swiggy expands its operations, how do you attract high-quality tech talent?
DV: This is one of the hardest things for a startup like Swiggy. We have doubled our tech team in the last 12 months and are now a 450-plus team. We do industry hires and campus recruitment and conduct various kinds of training for fresh engineering talent.
Internally, we also groom our own talent.
From an industry perspective, we have started to jointly work with universities, where the best students work on our hardest problems and create a funnel of solutions. We also spread the word about how Swiggy is a mix of entrepreneurial DNA with solid leadership solving some unique problems.
This makes it a unique proposition as we are talking about scale and also give much larger ownership to our engineers. This is very exciting and attractive to senior tech talent. We have hired multiple people from companies like Amazon and Google.
(Edited by Evelyn Ratnakumar)