How an investor evaluates the technology of an AI startup
A critical part of investing in artificial intelligence startups is understanding the technology. Here is an angel investor’s take on what investors expect to hear when they listen to pitches from founders of AI startups.
Our coffee intake has jumped as more and more of our day is spent sitting in coffee shops listening to passionate founders pitching AI-based solutions. A critical part of investing in artificial intelligence startups is understanding the technology. But before going deeper, we first try to understand whether the problem the startup is solving requires AI.
The key is not just to use AI, but to use it intelligently for problems that require it. Many tasks that one may feel like applying deep learning to can be carried out effectively using rules and basic automation. For a problem like helping a company close its books faster, automation is enough, because the process involves a fixed, narrow range of inputs and outputs. Applying AI here would not only lead to a drain of resources, but unnecessary complications in the implementation of an otherwise relatively simple program.
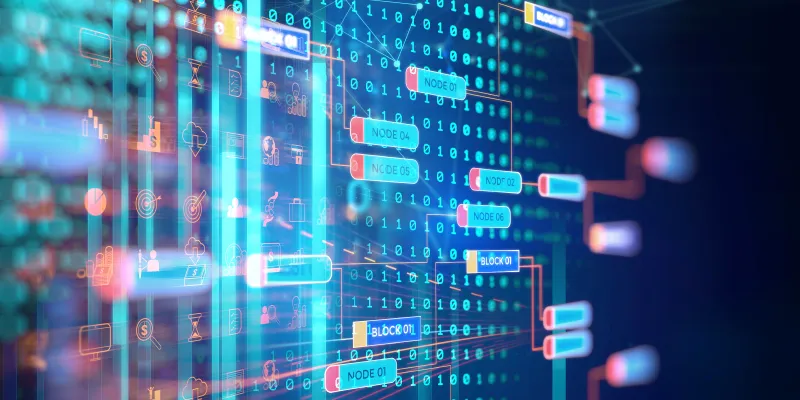
SenseAI, a fund dedicated to startups using AI, likes to invest in spaces like these, where an AI-driven solution is the most optimal approach to solving the problem. Once the need for AI is clear, we do a deep dive into the startup’s technology. To help us structure this assessment, we apply SenseAI’s framework, which uses four core factors required for success as an AI-centric startup:
- Variety in data
- Data acquisition
- Architecture
- Talent acquisition
1. Variety in data: Of the three Vs of big data (Volume, Velocity, and Variety), Variety plays the biggest role in AI.
Variety of data refers to the different kinds of possible inputs and outputs for a given system. When there are a few inputs and outputs and they are easily quantifiable, simple automation suffices. A good example of this is making ledger entries for a firm according to local accounting procedure and rules. The input is limited in its format to numbers, and the output too is determined by simple, static rules.
The game changes when we talk about a dataset like the visual inputs received by a self-driving car. Here, the variety of inputs is virtually infinite. The system must constantly detect and process input that is chaotic and varied. The same applies for situations like an unauthorised intrusion in the network of a submarine or a corporate environment. Voice-driven assistants, especially in the vernacular domain, are another example of effective use of AI.
2. Data acquisition: Effective machine learning or deep learning require vast quantities of data. Hence, a firm’s strategy to acquire data is crucial to its overall strategy. At a high level, the primary source of data is a combination of publicly available data, a firm’s own unique data, and customer/partner provided data. Any successful startup must have a strategy to acquire and integrate data from these sources in a cost-effective manner.
With regulations like General Data Protection Regulation (GDPR), there are regulatory restrictions around the capture, storage, and analysis of data. We assess startups based on their ability to recognise and work around these issues effectively.
If a startup can build large and high-quality datasets it can give them an edge over the competition. We ask questions like: is it possible to create a network effect around the data? Can we build a moat around the firm’s own data?
In a product experiencing the data network effect, as the volume of data provided to a learning system increases, it generates more value to any individual user even though they only interact personally with a small sliver of the data ‘network’. This effect arises due to relationships that emerge between different segments of data.
A data moat, on the other hand, describes a competitive advantage a business holds because of its proprietary data set. It’s the modern version of traditional moats such as branding, trade secrets, and regulatory capture.
We like to invest in startups that strategically exploit the above data-driven advantages and have comprehensive strategy for data acquisition and processing.
3. Architecture: The architecture into which the data is fed needs to be scalable in line with the long-term vision of the startup. We like to see if the architecture can be scaled to enterprise-grade or higher. An organisation can only fully utilise huge amounts of data from different sources if a sound data architecture is in place across the organisation.
An important factor the architecture determines is the analysis time frame. For some kinds of problems—say, obstacle detection in a self-driving vehicle—real-time analysis is essential. The processing power required is then proportionally higher. Other kinds of machine learning applications—say, assigning credit scores to borrowers—can function even with a relatively delayed response. It is important to ensure there is availability of adequate processing power and building of a data architecture fitting to the solution.
Decisions also need to be made about whether the data architecture is built on premise, on public cloud, or a hybrid combination of the two. These decisions must be made in accordance with the requirements of the product, and in a B2B model, in line with the client’s data policies.
We like to invest in companies that proactively understand the importance of these decisions.
4. Talent acquisition: We honestly don’t expect entrepreneurs to have answers and solutions to all the issues raised above. However, we do prefer those that have at least thought through these issues and have a rough plan.
Recognising the importance of these issues at a high level goes hand in hand with being able to hire the people that can explore the answers to these questions in detail. It is only in collaboration with domain experts that AI-based products can be built effectively. An entrepreneur’s ability to understand the talent they need and bring on those that are—in a specific field or technology—more knowledgeable than them, is crucial.
Having a simple framework like the one detailed in this piece, which distils years of understanding, helps us develop our own hypothesis around the team and product capabilities. Like any framework, we do understand that it may not be all-comprehensive, but it has allowed us to make some tangible decisions. We combine it with the frameworks we use for business evaluation to make the call regarding whether to invest or not.
(Edited by Evelyn Ratnakumar)
(Disclaimer: The views and opinions expressed in this article are those of the author and do not necessarily reflect the views of YourStory.)