The power of cheap prediction: how AI is transforming tasks, business models, and strategy
This book by University of Toronto professors shows how prediction machines are changing human judgment, jobs, and the broader economy.
Insights and frameworks for harnessing the powers of artificial intelligence (AI) are well charted in Prediction Machines: The Simple Economics of Artificial Intelligence, a book by Ajay Agrawal, Joshua Gans, and Avi Goldfarb. The authors are professors at the University of Toronto's Rotman School of Management.
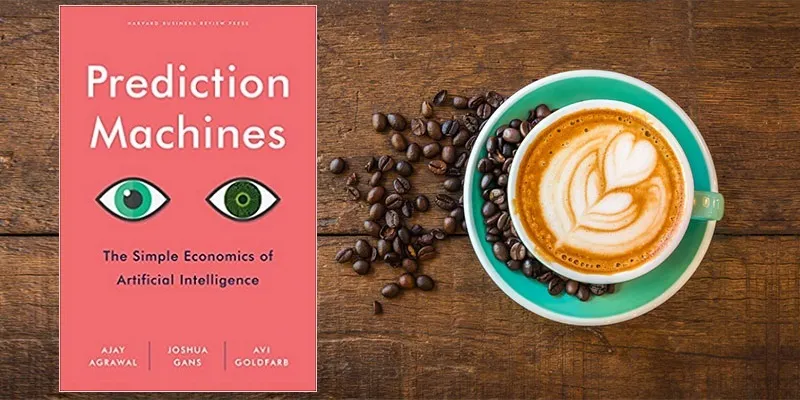
The 19 chapters are spread across 250 pages, and assess AI developments from the viewpoint of economists. Here are my takeaways from the book, summarised in the table below. See also my reviews of the related titles Seeing Digital; A Human's Guide to Machine Intelligence; Machine, Platform, Crowd; The AI Advantage; Human + Machine; Life 3.0; and The Four.
AI contributes a critical component of intelligence: prediction, which reduces uncertainty in decision-making, the authors begin. Deployment of AI involves a range of tradeoffs between speed and accuracy, data and privacy, autonomy, and control.
The authors invite readers to recall when they had their first ‘AI moments’, or when they realised the power of AI for the first time. This could be analogous to the ‘Sputnik’ moment, which triggered the space race.
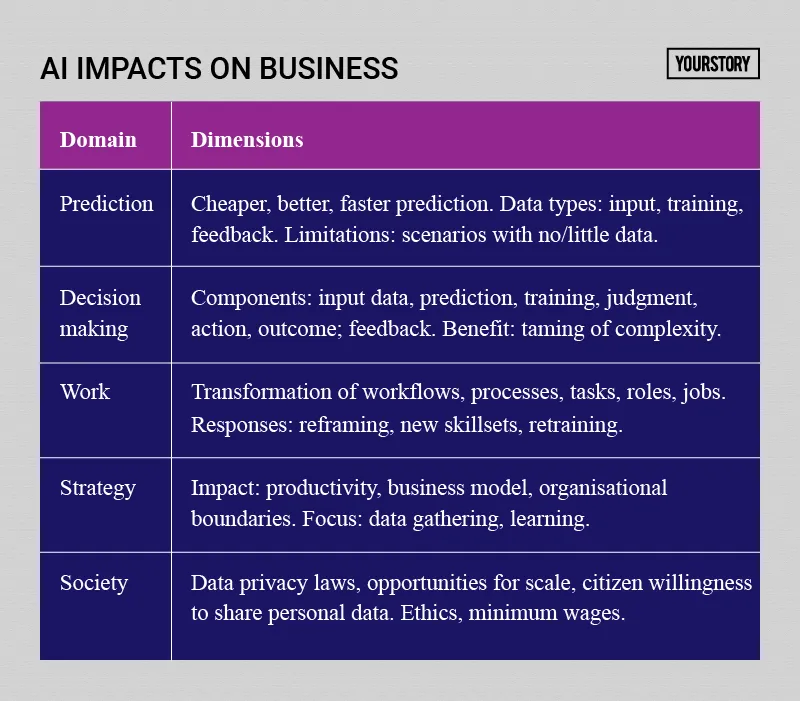
The power of cheap
“Economic history has taught us that the impact of major innovations is often felt in the most unexpected places,” the authors explain. After all, when the iPhone was launched, who would have thought that one day the taxi industry would be disrupted by companies like Uber?
Trends towards cheaper capabilities have impacted business models and entire industries. The Internet is an outstanding example of a recent innovation, which made lots of things cheaper: communication, distribution, research, and so on.
When Google made search cheap, this transformation disrupted industries like Yellow Pages, classifieds, and travel. It reshaped the media industry, and ultimately made Google one of the most valuable companies on Earth.
Earlier, such examples include cheap and abundant electricity. The computer itself made arithmetic cheap, which impacted music composition, digital photography, and language translation. Thanks to tech advances, AI is helping make prediction cheap as well.
“Cheap creates value,” the authors explain. “Cheaper prediction will mean more predictions,” they add. Prediction has improved traditional tasks like inventory management and demand forecasting. It has also reframed other problems as prediction problems, such as navigation and translation.
Cheap predictions will remove human roles for some tasks, create new roles, and also improve decision-making quality for humans in an augmented relationship. For some firms, the impact will be not just on productivity but strategy itself. The authors conjecture that Amazon’s business model could even change from shop-first to ship-first (‘anticipatory shipping’) if prediction becomes sufficiently accurate and the risk of returns reduces.
Cheaper predictions will increase the value of “complements” – data, judgment, and action. “In economists’ parlance, judgment is the skill used to determine a payoff, utility, reward or profit. The most significant implication of prediction machines is that they increase the value of judgment,” the authors emphasise.
Prediction
The power of prediction or filling in missing information helps interpret the past, present, and future, the authors explain. For example, credit card fraud detection involves pattern analysis of spending habits, balanced with an assessment of risks of declining a translation by mistake and causing customer dissatisfaction.
Framing translation as a prediction of words and phrases need not even require prior models of language like grammar. Telecom and financial industries use prediction to reduce customer churn.
The rise of the digital economy is making data collection easier and cheaper than before. For machine learning (ML), data is of three types: input, training, and feedback. Prediction machines require data in three stages – create, operate, and improve.
The risks of making a mistake differ with tasks and industries, eg. predictive typing in emails as compared to not predicting a heart attack. Prediction strengths of tools, and their brand perception, also play a role in user work habits; e.g. which search engine they use for common searches as compared to rare ones.
The authors chart how the rise of AI-driven prediction gives rise to new balancing points with respect to human prediction. Human prediction suffers when the number of variables is too high, or due to biases and prejudices; this is seen in industries like medicine and law.
However, humans may be better able to cope with novel or rare situations when there is no prior data, less data (‘thin data’), or little prior experience (‘unknown unknowns’). The authors also cite ‘Black Swan’ events like the rise of Napster, which upended the music industry; even human responses failed in this situation.
Even with powerful prediction machines, there will be a role for the learning power of experimentation. Examples include dealing with those who game or spam search engines, and finding out the right price point for recruitment services.
Humans and machines can predict better together as well, by using cognitive division of labour to balance out the different kinds of mistakes humans and machines make. Examples include improving skills in assessing the creditworthiness of loan applicants.
Decision making
“Making a decision requires applying judgment to a prediction and then acting,” the authors explain. Decision making requires input data, prediction, training, judgment, action, and outcome; the results yield feedback which, in turn, goes back to refining the decision.
Decision trees are a common decision-making tool in personal and professional life, and help map the flow of choices, actions, and consequences. Judgment will be needed to weigh the risks and costs of outcomes (‘payoffs’), or to value different scenarios and errors. Assessments which call for experimentation (and not just modelling or analogies) will also incur additional costs.
Prediction machines are capable of offering faster, better and cheaper predictions. This has impacted professions as varied as radiology and certified London taxi drivers. In the long run, cheaper prediction will increase the value of those tasks which require more human judgment.
Human desires and actions can add complexity in automated approaches to decision-making; e.g. driving routes may be chosen not just because of distance or time, but proximity to a fueling station or restaurant. Such subjectivity and idiosyncrasies need to be factored in as well, and make hard-coding difficult.
AI can help tame complexity by handling more ‘ifs’ and ‘thens’ in traditional ‘if-then’ models of conditional actions. This helps machines operate even in uncontrolled environments; e.g. autonomous vehicles in Rio Tinto’s mining operations, and future scenarios like mining on the Moon.
Humans often use ‘good enough’ solutions to problems, or shortcuts – rather than coming up with the best solution, which takes time, energy, and resources. Making such compromises has been referred to as ‘satisficing’ by Nobel Laureate Herbert Simon, whose work involved AI as well as economics.
Workflows
One section of the book describes how businesses should map out their workflows, processes, tasks, roles, and jobs. This will help them understand how AI can improve existing tasks, remove some jobs, and even create new processes, business models or strategies. This can call for new skillsets as well, and diminish the value derived from earlier skills.
This transformation is more than automation, and calls for re-engineering and even reframing the company’s mission. Business leaders need to be very clear on what ‘success’ means for the organisation, in terms of internal and external metrics, for the near term and long term.
As early examples, the authors explain how spreadsheets transformed the tasks of bookkeepers. Contemporary cases include the use of ML to transform fulfilment activities in ecommerce.
Challenges here lie in seemingly simple tasks like getting robots to grasp objects of diverse shapes, sizes and textures. Amazon hosts the ‘Amazon Picking Challenge’ to spur innovation in this activity (warehouses can be more complex environments than car assembly plants, in this regard).
Strategy
Devices like smartphones have diffused into and transformed a wide range of tasks, business models, and industries. Reduction of uncertainty via AI can change processes as well as business strategy, and even reshape organisational boundaries.
Tech giants like Google, Microsoft, Salesforce, Amazon, Baidu, and Alibaba have gained the most by investing heavily in data, algorithms and experimentation. “We want to be a connector to help accelerate cooperation, innovation and industry convergence,” says Chen Juhong, VP, Tencent.
Other players will need to partner with these giants, or catch up by building their own in-house capabilities. Examples include optimised demand forecasting, logistics, warehouse locations, and delivery at German ecommerce venture Otto.
The authors advise business leaders that if they want to become AI-first, data gathering and learning will need to become a key priority. This may lead to sub-standard offerings in the beginning, which will improve only as more data and activity improves the customer experience.
Revenue and user numbers may also take a hit in the beginning. There will be some error early on as the AI learns and improves predictions. Use of AI has implications for risk management, liability and brand perception as well, eg. in autonomous vehicles. Costs of error due to releasing an AI-enabled offering too early also vary across sectors.
One chapter classifies the different kinds of risks in AI. They include liability risk, quality risk, security risk, and data risk. Examples include unintentional biases and discrimination in ads and legal judgments, and malicious responses to public AI experiments (e.g. Microsoft’s Twitter chatbot Tay).
“Unique data is important for creating a strategic advantage,” the authors emphasise. This has implications for corporate acquisitions and partnership approaches.
Other trade-offs are between the speed and accuracy of learning in the cloud versus learning on the ground (or in devices). New human roles will emerge in training AI. Humans will be needed to refine judgment capacity, which may be difficult to assess due to the subjectivity involved.
Gathering and using data calls for tradeoffs with privacy and surrendering of consumer data. Such approaches have varied between companies (e.g. Google, Apple) and countries (e.g. China, EU).
Social implications
The book concludes with discussion on topics like responsible AI (ethical approaches), universal basic income (social safety net), approaches to lifelong upskilling, and how long it will take to create artificial general intelligence (AGI).
AI will have impact not just on jobs but the income generated from them, and who is best positioned to benefit the most from learning these skills. “AI will unambiguously enhance productivity,” the authors emphasise.
“The key policy question isn’t about whether AI will bring benefits but about how those benefits will be distributed,” the authors caution. Concerns also revolve around the monopolistic power of digital giants, and whether new challengers can arise.
China has an AI advantage in terms of its population size, AI budget, and control of citizen data. “By contrast, in Europe, privacy regulation makes data access far more stringent than elsewhere, which may shut out European firms from AI leadership altogether,” the authors caution.
“The rise of AI presents society with many choices. Each represents a tradeoff,” the authors sign off.
(Edited by Saheli Sen Gupta)