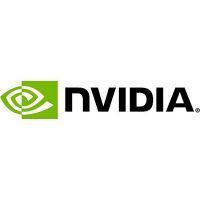
NVIDIA
View Brand Publisher3 strategies for enterprise AI success
Artificial Intelligence is rapidly revolutionising business. As AI enables industries such as healthcare, manufacturing and retail to become more innovative and efficient, enterprises are looking to Chief Information Officers (CIOs) to provide leadership and chart a path towards success. Well-managed companies find ways to thrive, especially during turbulent times, by drawing customers closer, streamlining to save costs, and seeking out a more agile position than their competitors. AI is incredibly valuable on all three fronts.
However, many enterprise-sized companies are used to doing things in a certain way, and the idea of a full-fledged, AI-driven operational transformation can be daunting. But, as Manuvir Das, Head of Enterprise Computing for NVIDIA points out, “AI is a powerful new technology that can make companies better, and companies know it, but not all know how to do it.” So how do you get your enterprise ready to move into an AI-enabled future?
NVIDIA has created technology to drive AI transformations for enterprises. They’ve also drawn upon their deep experience of using AI to run their own business — and helping other companies to transform their operations — to map out three key strategies for enterprise AI success.
1. Start AI in the cloud, but plan to take it hybrid as you scale for success
Cloud computing is popular among enterprises for good reason. Developing new solutions in the cloud makes it easy for development teams to get started, and cost effective for your business when new ideas fail on the path towards eventual success.
AI at scale, however, can quickly become expensive to run in the cloud. To that end, a hybrid approach actually makes the most sense for getting started. Plan your AI development to run in parallel so you can build quickly and be ready to scale by:
- Giving your developers and data scientists the freedom to start building in the cloud. It’s the fastest way to dive into new ideas and iterate quickly.
- At the same time, start building a hybrid (or co-lo) AI environment. Start with a single AI appliance. “You’ll learn a lot from working with a single box, from what tools to use with it, to how to connect it to another box and start building your network out,” Das says.
- When you’re ready to scale, bring your science and innovations from the cloud to your in-house environment.
Also remember that “on-premises” AI doesn’t necessarily mean feeding data from thousands of nodes into one or two giant data centers for processing. “The enterprise data center of the future won’t have 10,000 servers in one location, but one or more servers across 10,000 different locations,” says Justin Boitano, Vice President and General Manager for Enterprise and Edge Computing at NVIDIA. More and more enterprise AI use cases, from catching manufacturing defects on automated production lines to helping customers find what they’re looking for in smart stores, rely on real-time processing.
The latency incurred in sending huge troves of data back and forth between a centralised data center and sensors in a retail store aisle, traffic light camera, or robotic assembly line is a performance killer. Placing a network of distributed servers where the data is being streamed lets enterprises drive immediate action. That’s AI at the edge.
Look for an accelerated platform that offers a range of servers and devices with varying power and compute options, an easy-to-deploy cloud native software stack, and an ecosystem of partners supporting the platform through their own products and services. The platform should make edge AI easy on your IT department, as well, with the ability to securely and remotely manage your fleets. “The infrastructure has to be easy,” Boitano explains. “Just plug it in and connect it to the network. Everything is configured and distributed from a centralised location.” If done the right way, enterprise AI at the edge is almost as easy as plugging in a new piece of consumer tech.
2. Give data scientists the tools they need for success — but hold them accountable to enterprise goals and objectives
Gone are the days of “AI as science project” experiments that drain resources without delivering value. Meaningful use cases and measurable impacts for AI in the enterprise abound, and you need to map AI to solving business pain points.
That doesn’t mean trying to fit square pegs into round holes. Data scientists are data scientists, and CIOs should empower them to do what they do best. They need AI-ready infrastructure to build, test, deploy, and refine models. But data scientists also need to align their work with business goals. “Keep your experts focused,” Tony Paikeday, Senior Director of AI Systems for NVIDIA, advises. “AI is a team sport where IT supports infrastructure management, while data scientists concentrate on data analysis.”
Start by identifying problems your business needs to solve. Then look at how AI can enable those solutions. As Paikeday points out, this doesn’t mean reinventing the wheel. “The good thing is we have a lot of pre-built models for popular use cases,” he notes.
Once you know what problems you’re looking to solve, you’ll need a multidisciplinary team. Think about these roles and responsibilities as you build around your data scientists, including:
- Business analysts who understand their business problems
- Data engineers who understand data infrastructure
- App developers who can take the model and put it into production form
- An executive sponsor with vision to make it all work who can start championing resources
As with any new initiative in an enterprise setting, Paikeday recommends a pragmatic approach to getting the business end of things going. “Start with a quick win to get the momentum going,” he says. “Then the flywheel will kick in and things will really move.”
3. Let AI fit into the platforms you already know
AI may be new, but the way it’s deployed shouldn’t be foreign to enterprise IT. “Don’t think of AI as this weird unicorn tech,” Das says. “Treat it as any other IT appliance.”
Your IT teams are used to using certain tools and methodologies to manage and orchestrate your workloads. Have the same expectation for AI — your IT department shouldn’t have to learn a whole new way of doing things to support AI workloads. “Let the tooling that you use today for all of your workloads carry over — expect that from AI vendors,” Das explains.
AI appliances are, in many respects, just like the storage appliances IT teams are used to working with in data centres. Some of the software applications you’ll use with AI may be new, but others are architectures you’re already familiar with, like VMware, Red Hat, and Nutanix. That’s a big focus of NVIDIA’s work in the enterprise, to take the complexity out of fitting AI into your existing infrastructure.
That complexity is one of the biggest challenges inherent to transforming a large business with AI, as Paresh Kharya, Senior Director of Data Center Product Management and Marketing for NVIDIA explains. “The infrastructure is fragmented. You have a cluster of servers designed to do analytics and storage, and then a separate cluster of servers with GPU acceleration for AI training,” he says. A unified acceleration platform can remove the complexity of managing AI infrastructure. “The platform accelerates all of your workloads — data analytics, AI training, AI inference, and so on,” Kharya says. “You can manage your workloads as demand changes, even as it changes over the course of a single day.”
“Enterprise clients just want AI to work,” Paikeday adds. “They want to stick with the data storage providers, and the other providers they already use, and get a turnkey AI infrastructure that works with their stuff.” Look for an AI vendor offering purpose-built solutions for massive data needs, backed by a software stack with pre-optimised apps for most development frameworks. Turnkey AI solutions are out there, and they make enterprise transformation a lot easier, faster, and better for your bottom line.
AI Transformation should suit your business needs
AI is one of those truly revolutionary technologies that’s impacting virtually every line of work and walk of life. Businesses across all industries are recognising this, and forward-thinking CIOs are leading the way when it comes to AI-powered transformation in the enterprise. Planning for enterprise AI success isn’t fundamentally different than mapping out any other IT initiative: Build your technical roadmap (start in the cloud, then bring it home), empower your data scientists but make sure they’re aligned to the broader business objectives, and work with vendors who understand that AI should fit into the platforms you already know. AI is a game changer, and it should accelerate your company’s journey towards success, not upend the way you do business.
To learn about NVIDIA’s enterprise AI solutions, visit here. Also, this fall, GTC 2020 will take place from October 5-9. The event will feature the latest innovations in AI, data science, graphics, high-performance and edge computing, networking, autonomous machines and VR for a broad range of industries and government services. It is free for individuals from Government, Academia and Non Profit organisations.