[Startup Bharat] How Bhopal-based Swaayatt Robots is bringing autonomous technology to cars
Founded in 2015, Bhopal startup Swaayatt Robots aims to make connected autonomous driving technology accessible, affordable, and available to everyone.
Sanjeev Sharma always had a fascination for robotics. During his second semester of engineering, he had the opportunity to experiment with robotics, and he jumped in to gain the needed knowledge in the subject.
“It was in January 2009 that I stumbled upon the autonomous driving videos created by Team MIT for the 2007 DARPA Urban Challenge. Watching one particular video changed my life forever, and autonomous driving became a life goal,” says Sanjeev, an alumnus of IIT Roorkee and University of Alberta, Canada.
Working on autonomous driving made him start
in 2015 in Bhopal. The startup aims to enable Level-5 autonomy in highly stochastic and adversarial traffic-dynamics, and in both structured and unstructured environmental conditions.“We are currently testing our technology on Indian roads and Indian environmental conditions. Only when a technology is tested at this level of adversity and stochasticity in the traffic-dynamics and environmental unstructredness, should it be labeled as “Safe” to be deployed in markets abroad, and then in India,” says Sanjeev.
The product and technology
Sanjeev informally started his research in autonomous navigation in 2009, when he was in fourth semester of engineering, to solve the autonomous driving problem, focusing on theoretical CS and mathematical topics (machine learning, mathematical optimisation, reinforcement learning, motion planning, etc).
“Our product is an autonomous driving software, which can be integrated with any vehicle with necessary controllers and computational hardware,” explains Sanjeev.
The way the software works is the cameras and sensors (perhaps LIDARs) provide the visual sensory data. The perception algorithms process this data and build a 3D (or projected 2D) model of the world around a vehicle.
In parallel, localisation algorithms process the visual and other sensory data to compute a vehicle’s position -- against a GPS-only-map or a high-fidelity map. Planning algorithms (motion planner and decision makers) then compute the motion and behaviour of the vehicle to be executed by the controllers.
This is a very simplistic representation of a complex process internally. These algorithmic pipelines are not independent, and there’s a lot of cross communication and temporal-information exchange that happens in the system, including using information from previous compute cycles cross-algorithmic-pipelines.
“Until 2014, I was in academic settings. I was admitted to PhD at University of Massachusetts Amherst Fall 2014 PhD program, but I came back to India from Canada for a year, deferring my admission to 2015. But in India, I had one year to try things out. In 2015, I realised that I could solve the problem sometime soon, and decided to formally register the company. I gave up the idea of PhD, which was my goal since January 2009, as I started my autonomous navigation research,” says Sanjeev.
He adds that towards building the autonomous driving technology, close to 70 percent of the R&D efforts have been spent on developing motion planning and decision making algorithmic frameworks – the algorithms responsible for planning the motion and behaviour of the vehicle on-roads.
“We make use of (inverse-) reinforcement learning and apprenticeship learning for the navigation policies. Around 25 percent of the R&D efforts go in developing perception algorithmic frameworks to allow autonomous vehicles to perceive and contextually understand their environment,” adds Sanjeev.
He explains, the startup ensures the operational cost of the overall system is lower compared to existing competing technologies developed by prominent competitors – Waymo, Aurora, Argo AI, MobilEye (Intel), TuSimple, etc.
“This is where we are developing (i) novel mathematical framework for mapping the environments, consuming 50x less memory in terms of map storage, and 10-70x less computations in terms of inference cost against the maps, and (ii) continuing our existing novelties in planning and perception sections (like ability to generate delimiters on-the-fly) to get rid of the requirement of high-fidelity maps (a norm today). We are one of the 3-4 companies in the world with the ability to enable autonomous driving without high-fidelity maps,” says Sanjeev.
In all of his work, Sanjeev has realised that autonomous driving is a combination of several different branches of engineering, science, and mathematics (pure, and applied alike).
“Even if you decide a field, say computer vision, motion planning, pure machine learning, or pure electronics and embedded systems -- (at least MS with R&D experience to call yourself an expert of these fields, or even to make a significant enough contribution to the field at large that could make a difference) -- there is a long journey, and usually the journey is rigorously mathematical in nature,” says Sanjeev.
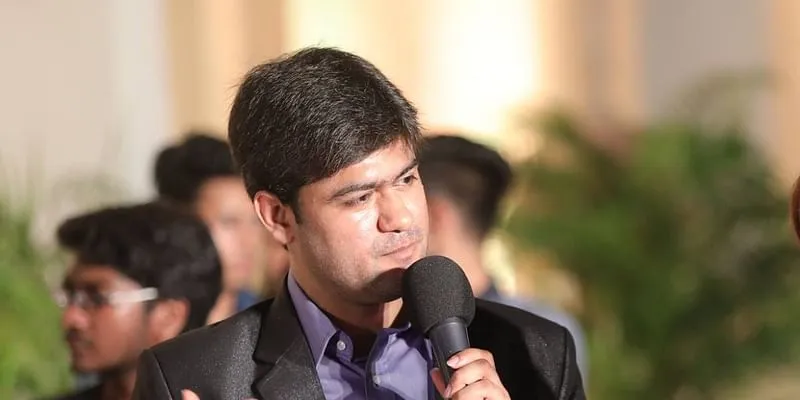
Sanjeev Sharma, Founder of Swaayatt Robots
Challenges
According to Sanjeev, one of the biggest concerns was the cost of setting up.
“While most autonomous driving startups were started with an initial investment of a few million dollars, I started the company with only around Rs 30 lakh in 2015 (less than $60K),” says Sanjeev.
“My primary focus has always been safety, innovating in motion planning and decision, where I have been actively using reinforcement learning to learn navigation policies for tough traffic scenarios,” he adds.
However, when he began the company, LiDAR used to cost $75K (which was more than the money he invested in the entire setup). Thus, in 2016, he started research to develop the technology that enables autonomous driving perception without using LiDARs or RADARs.
“Since I had research and mathematical background in theoretical computer science and applied mathematics (to some degree), I was working for myself from 2015-2018, and thus I was able to keep the cost under check to do the initial setup (computers, GPUs, vehicle, cameras, etc). Until 2018, I single-handedly developed most of the algorithmic frameworks in planning and perception departments. In the meantime, I had support from some of the most awesome interns, who later went ahead with graduate studies abroad,” explains Sanjeev.
The market and future
According to Fortune Business insights, the global autonomous cars market was valued at $1.45 billion in 2020, which touched $1.64 billion in 2021 and is expected to touch $11.03 billion by 2028. Giants like Alphabet, Robert Bosch, Daimler, Baidu, Pony.ai, Ford, Hyundai, Volvo, and others are looking closely at the segment.
Speaking of their technology, Sanjeev says,
“We have better technology compared to our competitors in Europe, North America, and China. In autonomous driving, safety is the primary concern. The way the industry is progressing, it will take them 10 years to ensure safety. Once that is resolved, other factors like the cost (including operational) will be a key factor, which encompasses various parameters, a prominent one is the energy footprint. We have developed algorithmic frameworks that are more capable in terms of dealing with environmental and traffic uncertainties. At the same time, our technology is much less energy consuming, derived from the computational efficiency of our algorithmic frameworks.”
Swaayaat Robots has already raised $3 million from investors in the US, and is looking to raise $9.5 million to scale its existing technology and to scale up R&D efforts significantly, and also to strengthen its collaborations in Europe and the United States, and potential collaborations in the UAE.
One of its key differentiating factors is the ability to learn navigation policies for various challenging traffic scenarios. Sanjeev says, they are one of the handful of companies globally who have been researching in and making use of reinforcement learning to learn such navigation policies.
“Other prominent companies in the space include DeepMind, but their focus is not autonomous driving. Wayve AI and Mobile Eye are two other companies making use of reinforcement learning. Our key clients are automotive and sensor OEMs. We hope that in the next 10 years, many companies would give up solving autonomous driving by themselves, and would want to leverage the technology being developed elsewhere. Thus, we are positioning ourselves as an autonomous driving software company, where our software will enable autonomous driving for a significant percentage of the vehicles worldwide by 2030,” says Sanjeev.
Edited by Megha Reddy