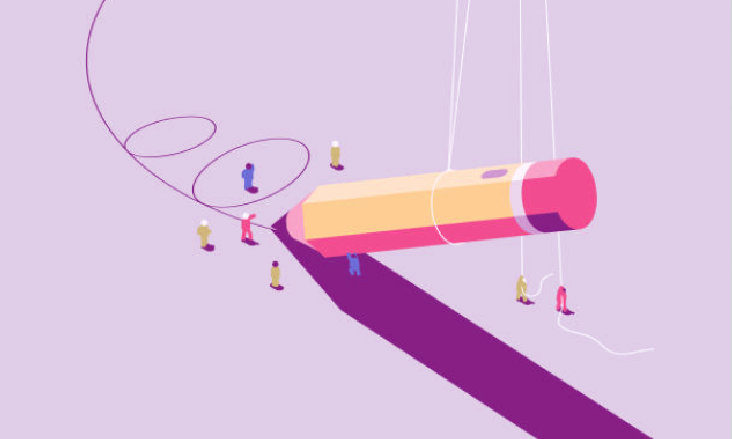
Why Data Readiness is the Key to Self-service Analytics
Amidst the craze of adopting big data and analytics in order to gain competitive edge, many businesses began encountering one persistent challenge: a shortage of data scientists and IT staff to manipulate and derive insights from the tons of data they aggregate from multiple sources. Out of this challenge, a solution was borne; self-service analytics. This is an evolution in advanced analytics that enables business users-who have little or no background in technology and statistics-to identify business opportunities through querying and generating reports from business intelligence (BI) tools. Business users interact with the analytics platform through highly graphical dashboards, which sit on top of simplified data models that enable easy access to data todrive real-time decision-making.
But even the most advanced big data technologies may not be of much use to a business that is not data-ready. Adequate data preparation is pivotal to the success of self-service analytics. According to a recent study by TDWI, business users and analysts in many organizations start experiencing data-related challenges during the data preparation phase. During this process, BI and data managers typically encounter poorly-defined data, and erroneous designing of data preparation and transformation modules. Some of the other major challenges associated with big data, which validate the need for data preparation tools include:
• Poor understanding of data, which calls for additional efforts to derive any significant value from it.
• Insufficient data quality and inadequate metadata, which undermines the trust users have in the data.
• Different varieties of data format, which hamper timely data exploration.
• Data sharing complexities compounded by the potential of having sensitive and personal data hidden deep within the data assets.
The outcome of these problems is that both business users and IT staff become underproductive. Data preparation challenges, when left unchecked, can prove catastrophic especially in today’s business environments, which are characterized by high mobility and rapid evolution. About 67 percent of the respondents interviewed in TDWI’s study cite financial constraints as the main challenge in their data preparation efforts. The other major setback most enterprises encounter is lack of a strong business case to justify data preparedness.
What are some of the ways in which self-service data preparation tools can address these challenges? According to Gartner, in its 2015 “Market Guide for Self-Service Data Preparation for Analytics”, these tools are capable of data discovery, data structuring, data exploration, data transformation, anomaly detection, data cataloging/inventorying, and sensitive attributes’ surfacing. The most important data attributes that organizations should focus on when embarking on data preparation include quality, accuracy, and validity. On a broader context, the data preparation tools offered by different vendors today are capable of:
• Supporting additional sources of data
• Applying advanced technologies such as machine learning, statistical pattern recognition, text analytics, and many more smart capabilities to data.
• Enhancing user collaboration experiences.
• Improving both data discovery and data quality capabilities.
Why is it critical that businesses adopt data preparation tools? The 2015 Gartner study finds that self-service data preparation tools can fuel a business’s towards data discovery and advanced analytics that is business-user-generated. The tools achieve this by mitigating on the complexity and cutting back the time required to prepare data for analytics.
It is worth noting that the same self-service data preparation tools also double-up as data governance platforms. The TDWI study notes that data governance is a key priority for most analytics platforms and the organizations that implement them. The concept primarily concerns itself with ensuring that sensitive data is secure and protected, and its use is guided by the stipulated regulations. Data preparation tools do, in fact, prioritize data governance, even though these capabilities have been abstracted from uses. These tools have also successfully expanded the definition of data governance to include focus on data quality, different data models, and sharable user-generated content, such as visualizations.
For businesses preparing to adopt self-service data preparation tools, Gartner has a few recommendations that can steer them in the right direction.
• Even though there are vendors offering either standalone, or BI-integrated data discovery tools, businesses should consider adopting the latter. When such tools integrate seamlessly with initiatives centered on BI, data management, and analytics, they effectively boost the data preparation process.
• Creating a deployment strategy to power implementation of self-service data preparation tools. A critical component of this strategy is evaluating the most suitable vendor to meet your data preparation needs.
• As noted earlier, data preparation tools seek to boost data governance through offerings such as metadata support, data lineage, and data quality enhancements. That said, organizations should not use these tools as a substitute for formal data governance initiatives.
• It is also critical that organization understand that self-service data preparation is a rich source of knowledge, which can complement enterprise data integration workflow. However, these tools should not come as a substitute to the more powerful and robust data extraction, transformation, and integration solutions.
When it comes to actual data preparation process, some of important factors to take into account include the depth and completeness of data, duplication levels. Data refresh frequency, data formats conformance, access availability, data consistency across different data sets, and data’s ability to service to ad hoc requirements.
Summary
Undeniably, there is a lot of value that data preparation brings to self-service analytics. Technology consulting companies can help organizations evaluate their need for data preparation and accordingly select the right tools. Self-service data preparation tools empower business users to achieve much more on their own with minimal dependence on data scientists and organizations’ IT staff, to service their data discovery and data analytics needs. The automation capability of these tools lessens the burden of manual work so that the process of data discovery, cleansing, and transformation are much easier and more effective. Data preparation also goes a long way in mitigating on the challenges that crop up during the preliminary stage of processing, whose outcome is a major determinant of the value and quality of data and information that fuels business decisions.