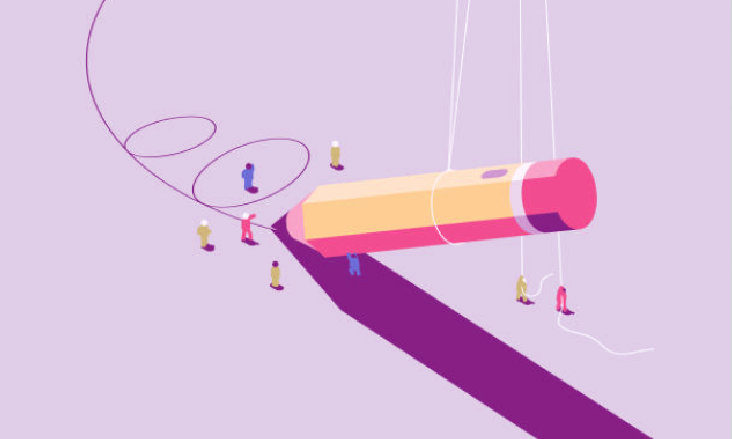
Combining video sensors with deep learning to benefit smart cities
Take a look into the future
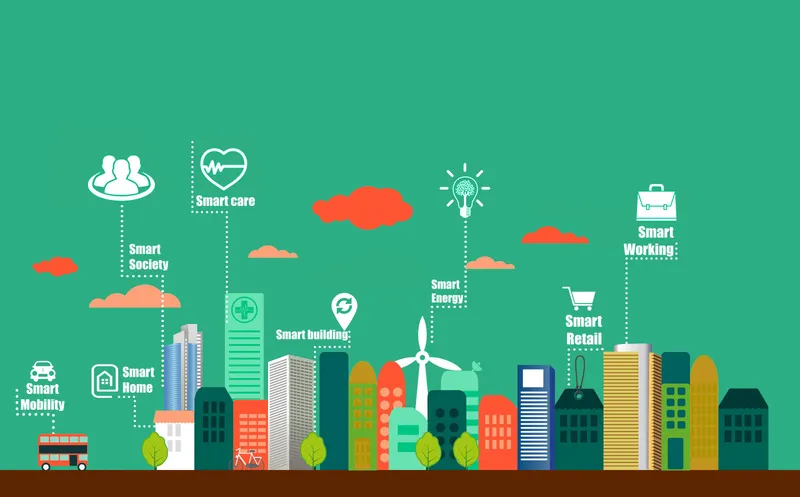
Smart city
Deep learning is being increasingly seen as the go-to-approach for sensors, speech recognition, computer vision, NLP and inevitably, smart cities. One (okay, more than one) step ahead of machine learning, deep learning has garnered enough attention and made enough noises to be noticed by the who’s who of digital technology.
Now, we know that machines are infinitely better than humans at tracking, tracking and unlocking data features. When humans are taken out of the equation, performance improves in a gargantuan manner.
In that parlance, deep learning offers an alternate, advanced approach to the conventional mechanism of Machine Learning, which lifts the weight of defining data-driven features and implementing them off human engineers who can only do so much.
Put simply, the algorithm makes sense of the entire spectrum of advanced computing – from raw sensor-related data to the actual output - by unraveling accurate features and decoding their calculation.
This leads to much more profound levels of computation, which makes them far more efficient than their predecessor. We have seen the efficacy of Deep Learning in Building Automation time and again, thanks to penchant for tracing the exact location and movement of people in a rom.
Smart Cities, Deep Learning and Video Analytics- The Seamless Connection
In the realm of smart cities, deep learning is ushering the advancement of smarter centers that reduces the burden of centralized data. Smart cities are all about leveraging IoT-based infrastructure that aim to improve the quality of life by optimizing resource utilization.
This inevitably needs a conduit of different advanced sensors, communication technology and advanced analytics to collect, process, analyze and implement the goals of these cities. Against that background, the emergence of video analytics can be a game changer.
Let’s discuss some trends.
GPU growth: The transformational growth of GPUs from being a limited niche to improve gaming experience to being the go-to-standard for big things like machine learning and AI is nothing short of phenomenal.
These trends have compelled companies to think architecturally about implementing technologies in futuristic concepts like AI cities. While discussing video sensors & analytics, it is important to know that video streams must adapt dynamically to different use cases, which necessitates a data-driven approach to smart cities.
Growth of security cameras: There are more than 500 million security cameras deployed globally, and that figure is on course to touch 1 billion by 2020. Considering how simple and efficient they are to deploy, this number could be way beyond 1 billion.
Cloud to remain as the go-to computation model: While data that has a physical presence in premises can never be totally redundant, it’s viscerally limited. Migrating data to the cloud will facilitate the sharing of video content with anyone, anytime.
Implementing video analytics a reality
The first step towards video analytics is training the AI to ‘learn’ what things like cars, trucks, builds and people are. With some human interjection, AI can swiftly adopt a self-learning model that learns and assimilate things at an exponential rate. It is important to add here like Cloud players like Microsoft Azure and Amazon Web Services offer GPU in a service-led model to impart deep learning training.
Once that happens, AI can then sift through large amounts of voice data to infer things accurately and quickly. Unlike humans who get fatigued beyond a point and miss out on details like people, number plates, or car colors, AI literally sees and identifies everything.
Unlike a local parking space or a building where only a dozens of cameras need to be installed and analyzed city-wide optimization needs the influx and analysis of tens of thousands of video feeds, for which, cloud is the right platform.
Business use cases
While it can be tempting to dismiss video analytics as a fantastical concept that can potentially be used to build smarter and better cities, their resonance for business use is apparent.
For instance, a retail shop can easily integrate video sensors with deep learning model to decipher its customers’ demographics. Each store can set inventory on the basis of how many men or women enter the shop, any specific patterns on specific days, holidays, etc.
Furthermore, building lobbies can make use of facial recognition to direct the ‘right’ people to appropriate sections and help them reach their destination faster as opposed to being stopped by humans who click pictures and then accesses different systems to ensure they’re sending the right people in.
The possibilities are limitless and the scope is endless. The confluence of video sensors and deep learning are set to foster breakthroughs that could make smart city a real and present reality.