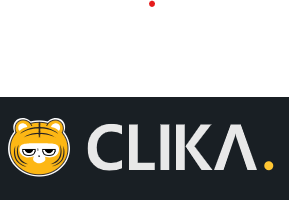
CLIKA’s
View Brand PublisherHow CLIKA’s automated hardware-aware AI compression toolkit efficiently enables scalable deployment of AI on any target hardware
Organisations are making a shift from experimental spending on AI to long-term investments in this new technology but there are challenges involved. Here’s how CLIKA can help.
With democratising AI and greater access to open-source AI models, enterprises today have made AI adoption a mission-critical imperative. According to Menlo Venture’s report, “2024: The State of Generative AI in the Enterprise,” 40% of generative AI spending now comes from more permanent budgets, signalling a shift toward long-term AI investments over temporary or experimental funding. Particularly, there has been a notable increase in the adoption of GenAI, for code pilots, support chatbots, enterprise search and retrieval, as well as data extraction and transformation.
However, as capable as they are, GenAI models tend to come in sizes and with architectural complexity that make their deployment on resource-constrained environments like edge devices technically challenging. But it is also the unpredictability of performance outcomes post-model-compression that makes model compression difficult and time-consuming, often requiring days and weeks of trial and error. This is because each target device comes with different memory capacity, operator support, processing capabilities, and computational power. These factors contribute to model performance variability.
As a result, compressing models requires tailored strategies to account for the diversity of complexities that are inherent in various model architectures, target hardware, and deployment environments. Successful model compression should be done in a hardware-aware fashion while retaining and ensuring consistent performance throughout all stages of the machine learning production pipeline. This is because as models progress through the optimisation pipeline and are converted into stage-appropriate formats, their performance can often degrade in the process.
We noticed that for mission-critical enterprises requiring on-device AI or multi-deployment solutions, ensuring the highest level of model performance consistency at inference—the final stage in the production pipeline after all optimisation processes and conversions—is crucial for building a reliable, production-grade AI-based solution. We also observed that the complexity of this pipeline could pose a barrier to productionising AI for developers without comprehensive hardware and software knowledge. While there are existing, third-party open-source tools, they often come with limitations (e.g. significant performance drops post-compression, difficulty of use, and more).
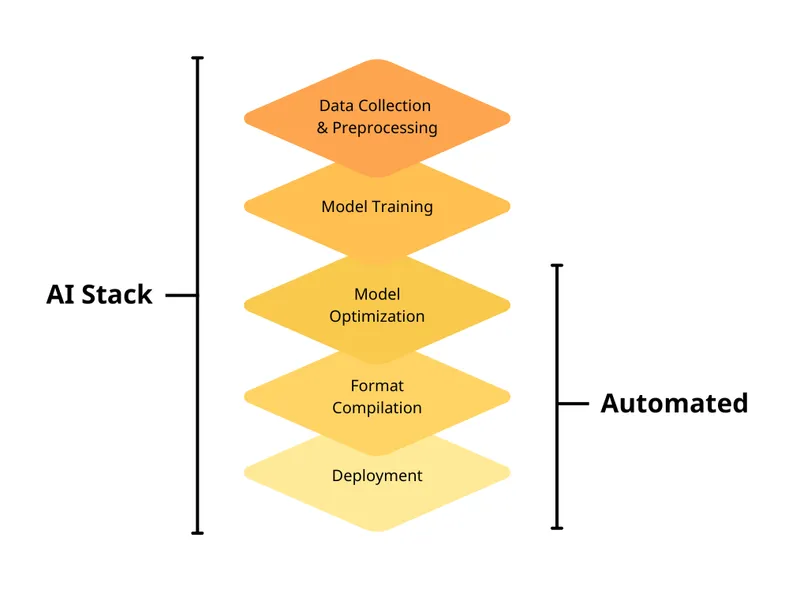
Figure1. CLIKA-automated Inference Optimisation Stack
CLIKA solves these pain points by providing an on-premises toolkit that “just works”. The company has an entire research team dedicated to building software that can automatically compress AI models efficiently on any target hardware. Its toolkit automatically compresses and downsizes models to a fraction of their original size and converts them into inference-ready formats for the target hardware, all without compromising performance.
This toolkit is powered by CLIKA’s proprietary compression engine, which is based on its internally developed quantisation algorithm. It sits on a user’s server, guaranteeing data and model privacy for security-sensitive enterprises. From vision and audio models to language and multi-modal models, the toolkit supports a variety of models for all major popular hardware devices, including those from NVIDIA, Intel, Qualcomm, ARM, and more.
With CLIKA, teams will be able to achieve faster time-to-market through productivity boosts, increase their ROI from AI, and cut down on costs by either packing more models onto the same device or purchasing cheaper hardware. Its toolkit’s automated compression feature empowers enterprises to efficiently scale the deployment of AI-powered solutions across a diverse range of devices.
"The past few months with the NetApp Excellerator have been incredibly valuable in helping us expand our global presence while also refining our solution for market readiness. The extended open innovation period provided us with the opportunity to engage with multiple teams and assess interest across the organization. The support from NetApp, especially from the NetApp Excellerator team members, has been instrumental in connecting us with the right people across the right teams. Their guidance has been crucial in advancing our progress and ensuring the success of our efforts".