[Techie Tuesday] From genetics to data science: Shantanu Bhattacharyya's many pit stops before arriving at AI logistics startup Locus
Our techie for today, Shantanu Bhattacharyya, went from majoring in biology at school to finding his calling as a data scientist at an AI logistics startup. Read his unlikely journey to heading an ambitious geocoding project at Locus.sh.
“People should be limited only by their imagination and not tools. The best data scientists are the ones who start with a problem to solve and use data as a tool. At present, almost everyone is doing the reverse - learn data science and then look for a problem to apply those techniques.” - Shantanu Bhattacharya, Data Scientist, Locus.sh.
The dynamics of data science are changing by the minute, opening up possibilities one could not possibly imagine, or rather did not imagine. The true north of this ‘science’ lies in its nature of extreme flexibility. Or so Shantanu Bhattacharyya, now Data Scientist at AI-enabled logistics startup Locus.sh, learnt quickly.
At Locus, 36-year-old Shantanu heads the Tiger Global-backed startup’s ambitious geocoding project, using a combination of natural language processing (NLP) and machine learning (ML) algorithms like recurrent neural networks (RNN) and different statistical methods. Using these methods, he interprets human written addresses and converts them into accessible coordinates understandable by a computer.
Before joining Locus.sh, Shantanu made several personal pit stops with his career, from Biology, Math, Physics, to Chemistry. The intersection of these basic sciences eventually led him to work as a Data Scientist with this logistics startup.
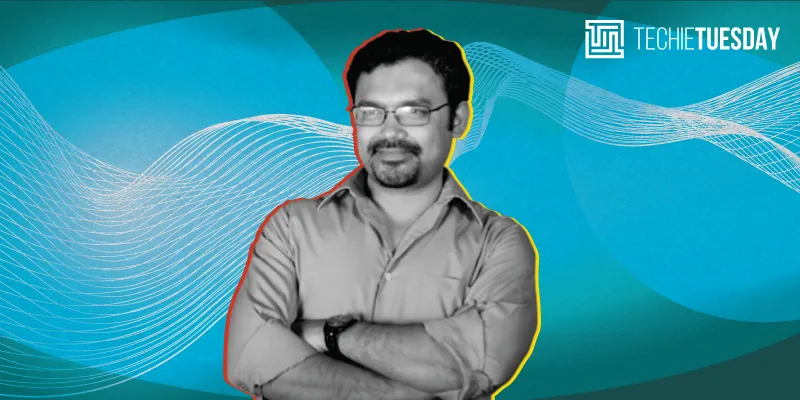
Shantanu Bhattacharyya, Data Scientist, Locus.sh
Creative mindset sans restrictions
Shantanu was born into a family where education was of utmost importance. When his father, a salaried employee at the Ministry of Information and Broadcasting, asked him to not score marks just because he knew how to, Shantanu took that piece of wisdom very seriously.
So seriously that it has translated into every single life choice he has made since then. While his father inculcated a certain fascination for Mathematics in him, Shantanu went a step ahead to develop a habit of original and lateral thinking, but not using linearly standard formulae and shortcuts, to solve problems.
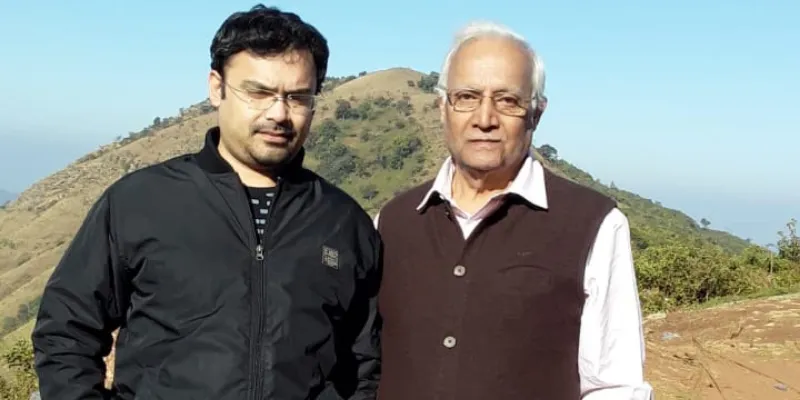
Shantanu with his father
This made him approach problem-solving in its true nature from a very young age. He identified that the education system in India was slightly degenerate, for his peers would run after solving scientific equations using tips and tricks. “These would only get them into a top technology institute or medical school. I wanted to solve them for myself - to better understand what I was actually doing,” recalls Shantanu.
In his 11th and 12th grade, Shantanu had an acute fear of missing out on greater opportunities because he was not an exceptional academic performer like his peers. Although he loved Biology and its empirical nature, he felt like the subject lacked higher reasoning as to ‘why things work’.
This was unsettling for Shantanu as he felt like the subject did not have much to explore further into, unlike Math, Physics, and Chemistry. Having had to learn Biology by heart, he was subconsciously attempting to arrive at the unifying theories of it - essentially through a physical model and approach to the subject.
Biology + Chemistry = Genetics
But, this conflict was soon resolved. Going a layer below Biology, Shantanu found the intersection of it and Chemistry to be in Genetics, and there was no looking back. Here, he could find all the reasoning he was desperately looking for. He recalls how structural proteins and studying DNA provided a steady learning mechanism, and gave more food to his thoughts.
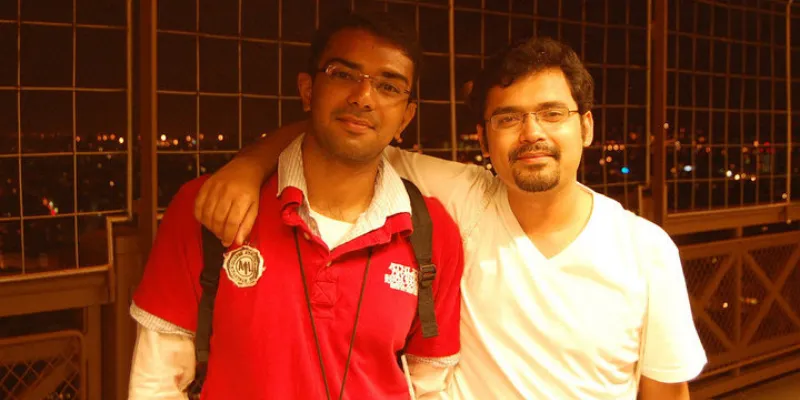
With a friend at the University of Mysore, during his M.Sc
Pursuing his B.Sc in biomedical sciences from the Delhi University from 2001 to 2004 and a Master’s in Biotechnology from the University of Mysore from 2004 to 2006, Shantanu eventually got selected for the KVPY fellowship. The fellowship funded his education from graduation to post-graduation, also allowing him to intern at any premier institution he wanted.
And Shantanu chose to intern at the molecular biophysics unit at IISc, stepping into the real world for the first time.
“I needed an incredible amount of discipline to put myself ahead of the competition. I learnt a great deal about collaboration and teamwork, and that your team members count on you to accomplish something. It’s a part of a bigger objective.”
Shifting focus to computers
With plenty of time to spare during his M.Sc, Shantanu shifted his attention towards computers for the ease with which these machines produced answers. In the process, he decided to change gears to computation and its science and landed in the Ivy League Carnegie Mellon University (CMU), USA in 2006 to pursue a PhD in Nuclear Magnetic Resonance (NMR) and Molecular Dynamics (MD) Simulation.
“I wanted a programme and a school to support me heavily in computation, offer a good biology programme, and some flexibility to do the course work comprehensively. It became really clear to me that I really enjoyed computers,” says Shantanu.
During his PhD with Dr Gordon Rule at CMU, he was learning to solve a great deal of problems with his specialisation in structural biology. While taking his time to adjust to the culture, Shantanu learnt that it was serious business to work on a student’s individual assignments, which involves a deep sense of accountability and individually original thinking.
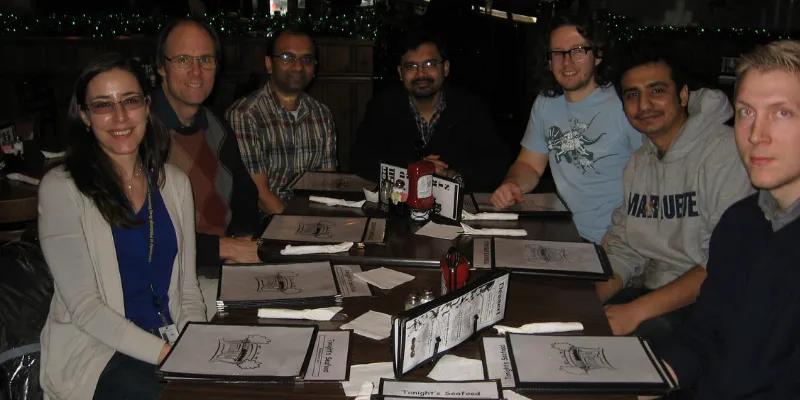
Dr. Gordon Rule (second from left), with Shantanu on the day he defended his thesis.
Obsessed with punctuality and discipline, he was able to align himself rightly with the culture at CMU - one of the best universities for Computer Sciences in the world. But probably because of Pittsburgh’s gloominess or otherwise, Shantanu felt that he was out of the race and might not be able to catch up with the cut-throat competition.
A professor at the University of Pittsburgh changed his mind, pulling him out of this trap. He told Shantanu, “If you think about who you are going to be and what you are going to do, you will never get anywhere. You are only wasting time. Instead, identify the problem, figure out how to solve it, and solve it.”
Combining computation with structural biology
Finally, Shantanu settled into his computer science classes. Gradually, it became clearer that he wanted to do a lot of computational work and loved the speed at which he was able to find answers. At CMU, students had the liberty to experiment with anything they wanted to. With this flexibility, Shantanu wanted to do his PhD research on molecular simulations, which he would run on a computer. Little did he know that this would create the groundwork for a potential career that would last for years.
As part of his post-doctoral research at The Scripps Research Institute (TSRI), Shantanu worked on a computational vaccine design for HIV. Understanding that it might take years for the work to produce substantial outcomes, he arrived at a common ground between the molecular research of DNA and computers to achieve immediate metrics.
While he was working on the HIV vaccine - putting together hundreds of data models and abstractions from the previous research-based observations - he was actually doing Data Science. But, he was yet to realise it.
The subtle art of Data Science
Initially being ambivalent about whether to pursue a job or keep studying, Shantanu was looking for ways to come back to India. Though the lifestyle was comfortable enough, there weren’t enough reasons to keep him in the US. Eventually, having heard about Locus from a common friend of his wife’s, Shantanu spoke with Niraj Dudani of Locus in 2016.
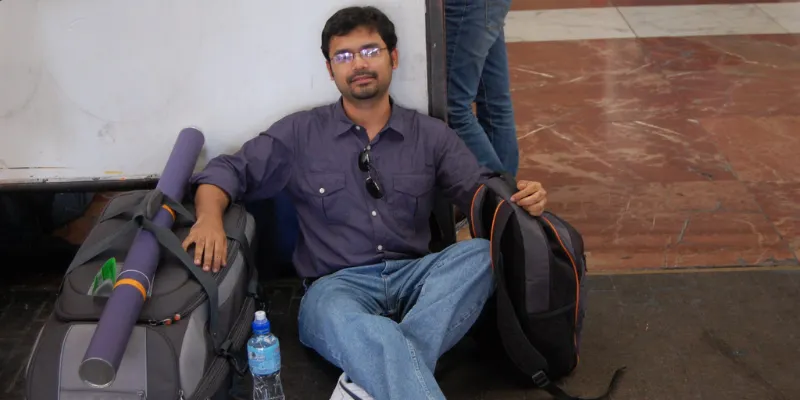
En-route a backpacking trip to Europe, during the Ph.D days
Following preliminary discussions, a puzzle arrived in his mailbox, which took him around a week to solve. Incidentally, Locus still follows the culture of scrutinising potential candidates by giving them puzzles to solve.
It was while solving this puzzle that Shantanu realised that he had been studying data science all along!
From doing data science with protein molecules, he learnt that he could implement it in pretty much everything. In July 2016, soon after he solved his puzzle, he was interviewed by Nishith while being driven around in a car all day, and by the end of it, Shantanu had a job.
Focal point at Locus
Shantanu’s core beliefs have led him to influence numerous things at Locus. In the geocoding project he is leading, he has had to train a machine to decipher physically written addresses and convert them into convenient mapping coordinates.
For a country like India, addresses are error-prone and descriptive rather than formatted. Geocoding is a primary hurdle if one has to automate decision-making in logistics. He uses the location data to provide intelligence on rider reliability and the changing business demands for several clients.
Shantanu says that the ultimate solution arrived when he was able to better define the problem in itself.
“It’s wrong to look at ML as a tool or a career path. It is only a convenient means to develop training models to solve problems in general. Whether I was working on the HIV vaccine or on the geocoding project for Locus 10 days later, I was only essentially executing data science as simply as I can implement it.”
Since data science is fluid in nature, it allows people from multiple fields of expertise to come and crack it. Shantanu believes if JRR Tolkien, being the brilliant linguist that he was, pursued data science to develop NLP models, he would have been the greatest NLP expert ever. And that is the kind of liberty and scope data science offers.
He says, “Predefined notions and prior experiences are very poor indicators to define success in data science. It teaches you the ability to define a problem statement in the most explicit way possible. So it’s equally important to adopt the same mechanism for your mind.”
If an insight goes wrong in the research of molecular biology, it doesn’t take any immediate effects since there are always several vetting processes in place. When you combine data science with producing better solutions for a business like Locus, one wrong turn in the computer will make thousands of riders take thousands of wrong turns.
Decoding the distortion between theory and reality
In theory, Shantanu could solve the complexity of geocoding and produce relevant answers. But in reality, ‘Pick up the keys from downstairs’ is also a part of the address - something you want the machine to understand and convey to the rider. The shift from theoretical mechanism to real-world business solutions, with technology and math, is where he had now arrived at.
“The collaboration that happens between teams here isn’t just about solving a problem, but that it should reach the end user. So it is about defining better problems and arriving at the best possible solutions,” he says.
Digging deeper into a specific problem has only given him more problems to solve, and in the process, Shantanu has been subconsciously nullifying all these possible problem statements. By extending his problem-solving approach to the real world, he realised that his work has real consequences, which only compelled him to identify better problems.
Identify better problems, not solve them better
Though there are multiple definitions to data science and analytics, Shantanu likes to simply call it a Feedback loop - a large-scale parallel experiment where one takes a bird’s-eye view of numerous unique events, separated by time and space. That’s where emotional intelligence of a human coincides with existing data sets, to create potential business intelligence.
“From being deep-rooted with its technological definition, data science is starting to become more scientific. Big data and visualisation as concepts are now being considered as data science. If you are able to figure out actionable solutions out of it, it’s data science. Otherwise, it’s only statistics.”
Shantanu stresses that the goal should be not to learn Machine Learning (ML), but to have the ownership to identify bigger problems in the existing reality. He says that a data scientist or analyst should be more on the lookout to figure out incoming problem statements, without standardised rules.
He, however, did not let go of his academic proficiency from his PhD days. Now, he takes some time to teach some courses within the organisation. Having gone through the journey of data science all his life, he now wants to implement the same with his future, and how his learnings could transcend across different dimensions to solve multi-disciplinary problems.
“In future, data collection would no longer be an important step. Every aspect of our life is getting documented and somewhere, some software is always generating data on us. A major challenge in the future would be to protect this data. Our personal lives should not end up getting commoditised,” he says.