From learner to leader: How businesses ride the artificial intelligence wave, and how you can formulate your AI strategy
The need of the hour is for productive, effective, innovative and humane artificial intelligence solutions, as this bestselling author explains.
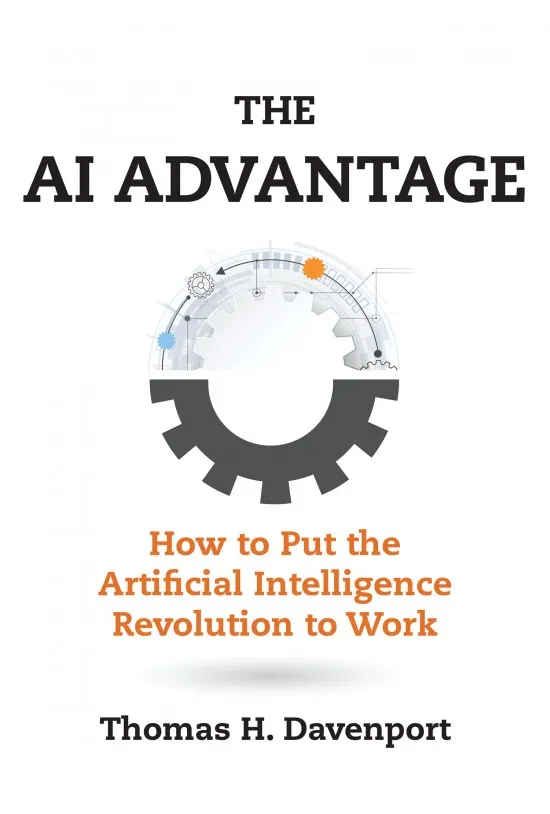
Launched in 2012, YourStory’s Book Review section has insights from over 180 titles on entrepreneurship, creativity, innovation, leadership, design and digital transformation. Also see our list of Top 10 Books of the Year for Entrepreneurs for 2018, 2017, 2016, 2015, 2014, 2013 and 2012.
Artificial intelligence implementations are becoming increasingly visible today in smartphone interfaces, customer relations, sales, operations, corporate IT, product development, supply chains, and manufacturing. While the creation of artificial intelligence algorithms is becoming easier and cheaper, the challenge is in infrastructure integration, individual behaviours, and organisational cultures, as this new book explains.
Babson College professor Thomas Davenport, the author of bestsellers in knowledge management and analytics, provides a range of frameworks and case studies of AI deployments, strategies and startups in his latest book, The AI Advantage: How to put the Artificial Intelligence Revolution to Work. His earlier books include BigData @ Work: Dispelling the Myths, Uncovering the Opportunities (see my book review here).
The book draws extensively on artificial intelligence surveys conducted by Deloitte, McKinsey, Gartner, and Teradata. The eight chapters are spread across 250 pages and make for an informative read. Here are my seven sets of key takeaways from the book. See also my reviews of the related titles Human + Machine, AI and Analytics, Life 3.0, and Big Data Revolution.
I. Artificial intelligence: hope and hype
Over the past decades, artificial intelligence has been through “winters” and “springs,” but is revitalised now. “Startups build their businesses around new technologies. Large enterprises are typically next in line,” Tom explains. Overall, many companies are testing the waters of AI rather than taking a deep dive.
The most aggressive users of AI in products and processes are digital-native companies like Amazon, Google and Facebook, who are entering other sectors as well. Large banks and professional services firms are also coming on board, and disruptive AI startups are a major competitive threat to incumbents.
The book cuts through the hype of the AI craze (eg. hopes that IBM’s Watson can cure cancer), and explains real-world applications of AI. Instead of going for moonshot initiatives, Tom advises organisations to go for a combination of projects that can harvest low-hanging fruit.
Rather than replacement of workers, AI’s contribution is in operational improvements and augmentation. AI is a “straightforward extension” of what companies are doing today with data and analytics, and is slowly coming of age.
Artificial intelligence is well-suited to automate structured and repetitive work, provide extensive analysis of data through machine learning (“analytics on steroids”), and engage with customers and employees via chatbots and intelligent agents.
As prudent observers have explained, it is important to look at what AI has accomplished so far, where it has failed, and where it might soon spectacularly succeed. AI is real but not easy and calls for a systematic assessment of data availability, process maps, competitor moves, AI talent, and vendor partnerships.
Tom cites Amara’s Law in this regard (named after scientist and futurist Roy Amara): “We tend to overestimate the effect of a technology in the short run and under-estimate the effect in the long run.” According to Kevin Kelley, AI will eventually be compared to electricity – ubiquitous, embedded and even boring.
While AI is currently providing evolutionary benefits, it is likely to be revolutionary in the long run, Tom predicts. Companies will succeed in AI if they take the long view, invest steadily, and improve their capabilities.
II. AI technologies and functionalities
The author classifies seven types of AI technologies and functionalities (see summary in Table 1 below). These technologies differ in terms of factors such as model transparency and dependency on labelled data.
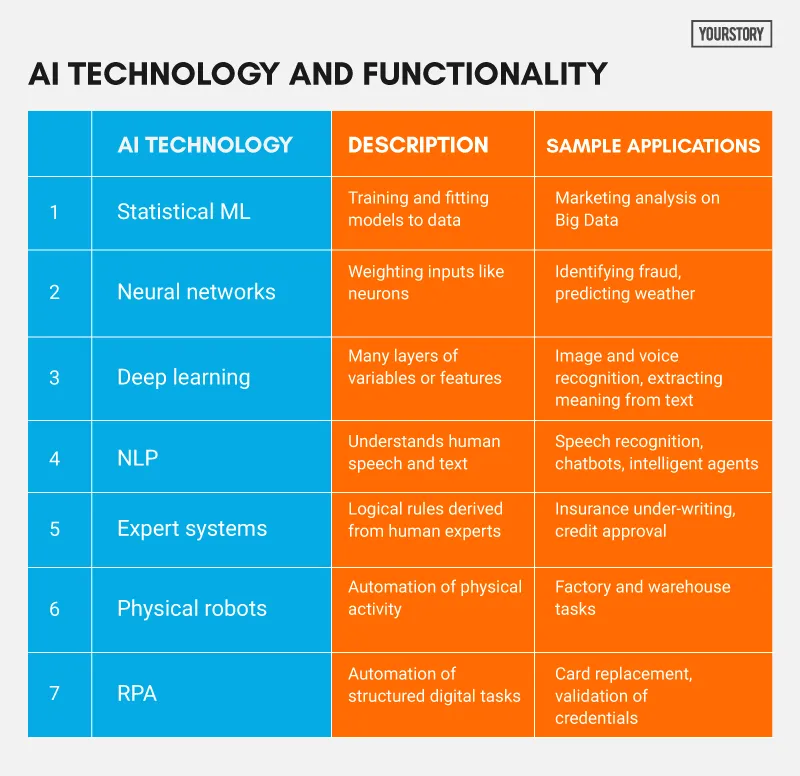
Machine learning (ML), based on statistics, uses techniques like gradient boosting and random forests. Supervised learning is used for fraud prediction. Reinforced learning is used in gaming contexts.
Deep learning models for image and voice recognition can exceed human capabilities in some areas. Deep learning using back propagation has been used by AlphaGo. Geoffrey Hinton is regarded as the father of deep learning.
Statistical NLP is based on ML, as compared to semantic NLP (based on syntax and ontologies). The “brute force” correlational approach of statistical NLP can be quite effective. Making sense of questions is different from answering questions, which is dependent on context and intelligence.
Rule-based experts systems require human experts and knowledge engineers, but challenges arise when the number and interactions between rules increases. Cognitive-aware physical robots are becoming more collaborative than traditional robots, which even had to be cordoned off at times.
Robotic process automation (RPA) for “digital labour” involves software and workflow rules rather than physical robots. It can be combined with chatbots and machine vision.
III. Artificial intelligence capabilities
The author defines three types of artificial intelligence capabilities in organisations: process automation, cognitive insight, and cognitive engagement (see my summary in Table 2 below). These categories can be combined as well, and projects can be prioritised depending on data silos, process costs, data volume, and speed of decision.
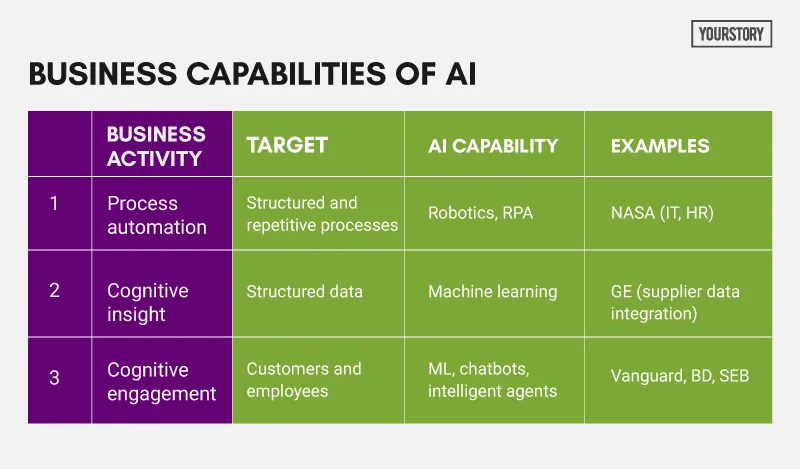
“RPA is the least expensive and easiest to implement of the automation technologies, and typically brings a quick and high return on investment,” Tom explains. NASA uses RPA in accounts payable and receivable, IT, and HR. “So far it’s not rocket science,” says NASA’s Jim Walker.
Cognitive insights using AI can detect patterns and interpret meaning, almost like “analytics on steroids.” This is the oldest category of AI and has been used for prediction, fraud detection, personalised targeting, and insurance modelling. GE uses technology from Tamr to integrate supplier data by eliminating redundancies across contracts; this leads to better contract negotiation.
Cognitive engagement with natural language conversations spans customer service, employee questions, and recommendations for retail and healthcare. Vanguard has an intelligent personal advisor agent for customer queries. Nordic bank SEB tested the internal Aida IT helpdesk application (based on IPsoft’s Amelia), then rolled it out for customers.
Some companies have created “cognitive centres of excellence” (or tech outposts) to develop AI capabilities that increase with time and data availability, such as medical giant BD’s Global Automation function, and health insurer Anthem’s Cognitive Capability Office.
Lowe’s Innovation Labs in the Seattle area have developed the LoweBot store robot to guide customers and even clean stores. Insurance firm Sompo Holdings uses AI at its centres in Tokyo (ML), Silicon Valley (autonomous vehicles), and Tel Aviv (cybersecurity).
Cognitive work redesign can benefit from disciplines such as design thinking to restructure work processes in an experimental, iterative and agile manner. Employees will need to be educated in new ways of working, Tom advises; this calls for early exploration and experimentation by business leaders.
IV. Cognitive strategies and tasks
Business strategies focus on what to make and sell, and how. Accordingly, artificial intelligence opens up new avenues for tackling existing problems or targeting new opportunities; these, in turn, can be classified as internal or external, with respect to decision making, innovation, knowledge retention, and process optimisation.
Companies will need to design and evolve specific strategies for AI with respect to content, talent, startup acquisition, and even country-level approaches. Specific steps identified by Tom include buying or accessing content databases, organising capacity-building workshops, and forming partnerships with companies like Nvidia and Waymo.
Countries ranging from China to Singapore have launched ambitious artificial intelligence strategies. China wants to become the world leader in AI by 2030. Singapore has plans for a national corpus of speech data, AI apprenticeship programmes, business initiative investment, and the Smart Nation initiative. Though the US has strong private sector effort in AI, the government seems to be falling behind, Tom observes.
One chapter focuses on the specific tasks that AI can perform, along with implications for organisational structure and business processes (see my summary in Table 3 below). Some of these tasks are already mature in terms of AI use, but others could take several years more.
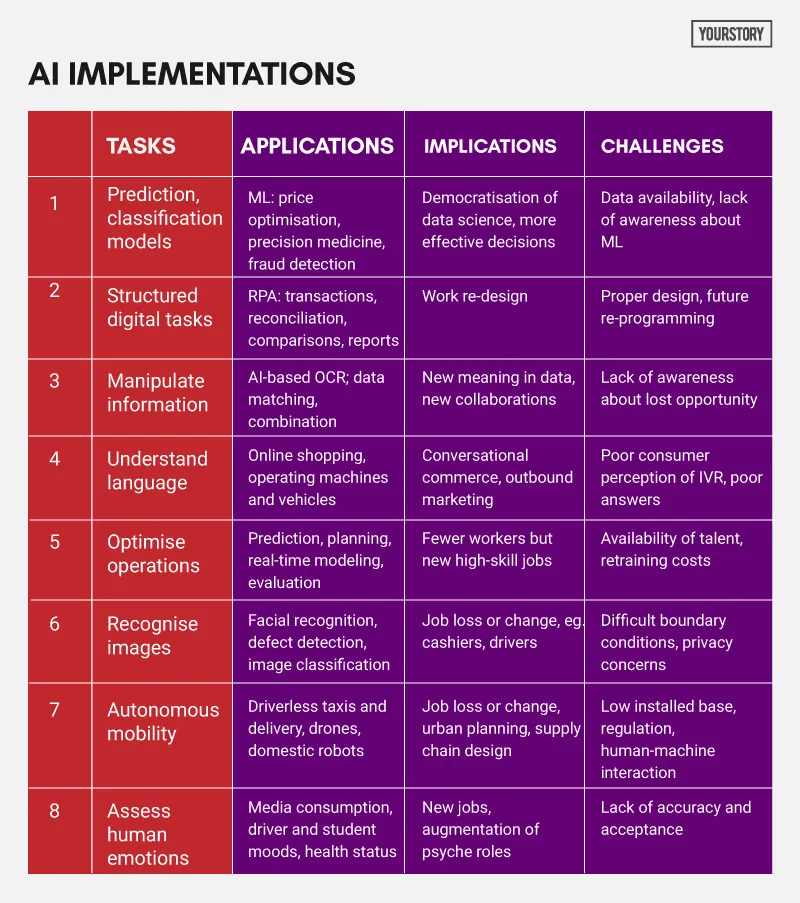
V. Talent and technology
Artificial intelligence has implications for both automation as well as augmentation at scale. Automation can lead to cost reduction and operational improvement, while augmentation favours innovation and flexibility, Tom explains.
Jobs consists of many tasks, and some of these can indeed be automated or augmented by AI. Full automation may not always be feasible due to economic, ethical, social, legal and regulatory reasons. Some companies do view AI as a way to directly cut jobs, while others may not hire additional employees after retirement in case AI can perform their jobs.
The experience of previous generations of technology also shows that massive automation may not take place, eg. ATMs and internet banking did not decimate the bank teller profession. Furthermore, new kinds of job roles will also be created, eg. Man-Machine Team Manager (as identified by Cognizant), or Explainers and Sustainers (as explained by Accenture).
Tom identifies five steps to deal with automation: step in (improve machines and fix mistakes), step up (move to higher-order big-picture roles), step aside (move to intelligences other than cognition), step forward (create next-gen AI tools and code), and step narrowly (specialise).
The author also draws on the autonomous vehicle classification of six levels, including partial, conditional, high and full automation. AI technology with respect to automation has been classified by consultant Anand Rao into three types: assisted intelligence (now widely available; improves what people already do), augmented intelligence (emerging; helps do new things), and autonomous intelligence (future; machines act on their own).
The jobs that will change the most, according to the author, are those that have high structure and repeatability, do not directly involve customer contact, are data-centric (eg. digital ad targeting), and are entry-level. To prepare for the future, Tom advises that people continually learn new domain knowledge and skills in communication, emotional intelligence and analytics, or particularly those that add value to what machines do. Companies should start awareness campaigns about AI, capacity-building workshops, new roles, and even new departments in this regard.
In terms of AI technology capability, companies have a number of options: build their own cognitive applications, implement them via vendors, or use open source capabilities (Tensorflow, Caffe, Torch, Cognitive Toolkit, DSSTNE). Companies should start becoming data-ready, and lay the “data groundwork” to exploit internal as well as external data.
For example, companies like Procter & Gamble and American Express have been working on AI since the 1980s. Kone, Black Diamond, and US Bank are using AI capabilities of SalesForce.com’s Einstein (eg. sourcing technicians, product recommendations, and lead scoring, respectively). SAP offers a range of capabilities collectively called Leonardo ML for cash management, video analysis, and trouble ticket management.
Bank of Montreal started with a Smart Core of data capabilities, moving from regulations and security to customer acquisition and growth, via RPA and ML. GlaxoSmithKline uses AI to integrate its research data across silos, such as health records, clinical trials, and DNA sequencing. The approach was based on Tamr’s ML technology instead of top-down “map and move” master approaches; the company is also tying up with UK BioBank for genetic data.
VI. Case studies and disruptive players
The author documents the “Cambrian explosion” of experiments in AI, in the words of Gill Pratt, head of the Toyota Research Institute. This covers the work of emerging startups as well as established giants, along with partnerships and M&A activity.
GE has internal and external AI initiatives, ranging from digital twins to supplier information integration. Digital twins can be extended to entire fleets as well to detect patterns, anomalies and signatures in machine performance. Industrial robots can even learn from each other, making factories more efficient, reliable and flexible.
Verizon’s Data Science and Cognitive Intelligence group, headed by Asim Tewary, applies AI to marketing and customer care. Capital One uses the text-based customer service chatbot Eno.
Singapore’s DBS Bank has implemented a range of AI projects, such as ML to predict ATM refilling needs and churn of salespeople, fraud detection algorithms, robo-advisory, and customer chatbots. Its goal is to use AI not for reducing headcount, but for moving from transactional to relationship banking.
Insurance startup Lemonade has put AI at the centre of its business. Act 1 of insure-tech focuses on better user experience, particularly for younger consumers; Act 2 will build on the mountains of data and transform risk and insurance in unique ways, according to its co-founder Daniel Schreiber.
IMB Watson helps Sloan Kettering’s cancer centre learn from the 2.5 million new medical research articles published each year. It also helps Novartis, Pfizer and Celgene speed up the processes to research, develop, test and launch a new drug. Watson for Drug Discovery has ingested 27 million abstracts and four million patents.
Working with IBM Watson and AI vendor Cognitive Scale, Macy’s conversational agent handles 25 percent of customer queries on its website. Watson beat human experts in the TV game Jeopardy in 2011, and is now an ecosystem platform for a range of APIs. “The company’s marketing has gotten ahead of its ability to deliver results,” Tom cautions; and advises more judicious marketing instead.
Using ML, Cisco’s Global Customer Insights group autonomously generated 60,000 customer models of their “propensity to buy.” Autodesk uses Everstring’s AI-based business mapping methods to identify potential partners in its Enterprise Business Agreement Propensity Model. Boston Consulting Group uses ML to analyse companies’ strategies and make recommendations on transformations.
Lenovo has made a number of AI innovations in the user interface, such as facial recognition, healthcare wearables, and conversational learning. Autodesk uses generative design powered by algorithms to come up with novel designs and prototypes, such as cabin partitions for Airbus.
Monsanto (acquired by Bayer) offers prescriptive planting services for farmers. Boeing, Cummings, Caterpillar and Bosch use AI for predictive maintenance. Procter & Gamble uses recommendation services for cosmetics and beauty aids. Levi’s works with AI startup ModeAI for its Virtual Stylist, using ML and deep learning image recognition.
ServiceNow’s NLP helps companies handle internal IT and HR questions from its employees. UPS uses ML to fix daily routes, adjusting for weather and traffic in real-time. SalesForce.com has AI capabilities clubbed as Einstein, and has acquired a number of startups.
Google’s AI initiatives are evinced in AlphaGo (based on technology from acquired startup DeepMind), Google Translate, RankBrain, Waymo, and Calico, spanning self-driving cars and medical diagnostics. Facebook’s image recognition algorithm Lumos is used for content filtering and personalised ads. Amazon uses ML for demand forecasting, product search ranking, recommendations, merchandising placements, fraud detection, and translation.
Startups like Canada’s Element AI offer “AI as a service.” The startup OpenMatters uses AI to classify and recommend business models. Lola incorporates human and machine-driven help to leisure and business travellers.
NASDAQ bought Australian AI startup SMARTS to identify problematic trades and partnered with Digital Reasoning for trader NLP. Bank of New Zealand is working with Intel Saffron (an AI tool acquired by Intel in 2015) to accelerate crime detection.
AI is powering new business models for autonomous, shared and electric vehicles. In this regard, Ford acquired Argo AI, and GM acquired Cruise Automation; both auto giants have Silicon Valley outposts. Uber and Lyft use ML for route planning and pricing.
AI is helping amateurs play wider and deeper roles by assisting with a number of tasks, such as website design and app development. Large firms are more likely to implement AI than SMEs are, and this will help big companies get even bigger, Tom observes.
VII. Larger issues: workforce, ethics
The concluding chapter addresses the ethical and social implications of artificial intelligence, ranging from algorithmic bias and explainability to privacy and regulation. After all, technology simultaneously creates problems as well as new solutions. While AI has enormous potential for good, it can also have unintended consequences, and businesses should avoid doing harm to society, the author cautions.
Challenges include algorithmic bias with respect to human identity, as seen in algorithms predicting criminal activity. Hiring approaches using AI should ensure non-discriminatory practices. Other problems have arisen with route navigation; some neighbourhoods are banning through traffic from ride-sharing services.
The author recommends steps like ML model transparency, the disclosure of artificial intelligence usage to consumers, algorithm explainability, external reviewers, algorithm certification, and careful assessment of external or additional data over time. For example, one aspect of GDPR is the “right to an explanation.”
Combined approaches with humans and AI will be needed to tackle fake news on sites like Facebook, such as technical tools for spotting fake news, third-party fact-checkers, and warning systems. Microsoft had to discontinue its Tay automated social media system, which began to spew out hate messages.
Job loss has been another fear surrounding AI. “The offshore business process outsourcing industry is likely to be the hardest hit. If you can outsource a process to people thousands of miles away, you can probably automate it with RPA,” Tom explains. He advocates that companies give adequate training, upskilling and participatory experimentation for employees; a sense of judicious balance between opportunity and responsibility is needed.
A number of artificial intelligence approaches have been used for cybersecurity (though it has also been used for cyberattacks), such as Google’s Bouncer scanner against malicious app code, and Recorded Future’s threat mapping.
More dialogue and collaboration is needed between ecosystem players. AI in healthcare, for example, will need to factor in the broader ecosystem of regulators, insurance firms, hospitals and patient communities.
Trends to watch include the rise of IoT, which will generate even more volumes of data. “Only AI will be able to address data of this volume and frequency,” Tom predicts. Artificial intelligence will work particularly well for companies that already have had success with earlier digital transformation.
“AI holds fantastic promise for extending the reach and range of human capabilities,” Tom sums up. The need of the hour is for productive, effective, innovative and humane AI solutions.