Data strategy: How to progress from use cases to business impact
This wide-ranging book describes six uses cases for data insights, and five steps to harness the data advantage. Here are some key takeaways and tips.
Launched in 2012, YourStory's Book Review section features over 320 titles on creativity, innovation, entrepreneurship, and digital transformation. See also our related columns The Turning Point, Techie Tuesdays, and Storybites.
A useful overview of data-driven capabilities and business strategies is covered in the second edition of the book, Data Strategy: How to Profit from a World of Big Data, Analytics and Artificial Intelligence, by Bernard Marr.
The data potential offers a massive advantage for businesses, but many companies are still not harnessing data effectively. This guidebook covers practical insights and dozens of examples on how to gain a competitive edge in a data-driven world.
Bernard Marr is a bestselling author and consultant, and is regarded as an industry influencer. He has written over 20 books; see my review of his earlier book, Big Data.
Here are my key takeaways from the 260-page guidebook, summarised as well in the table below. See also my reviews of the related books Winning in the Digital Age, Future Tech, Innovation Ultimatum, Seeing Digital, Out-Innovate, From Incremental to Exponential, The Next Billion Users, and Machine, Platform, Crowd.
“Those companies that view data as a strategic asset are the ones that will survive and thrive,” Bernard begins. This applies to “big and small” data, and the way data insights are used to generate real-time value.
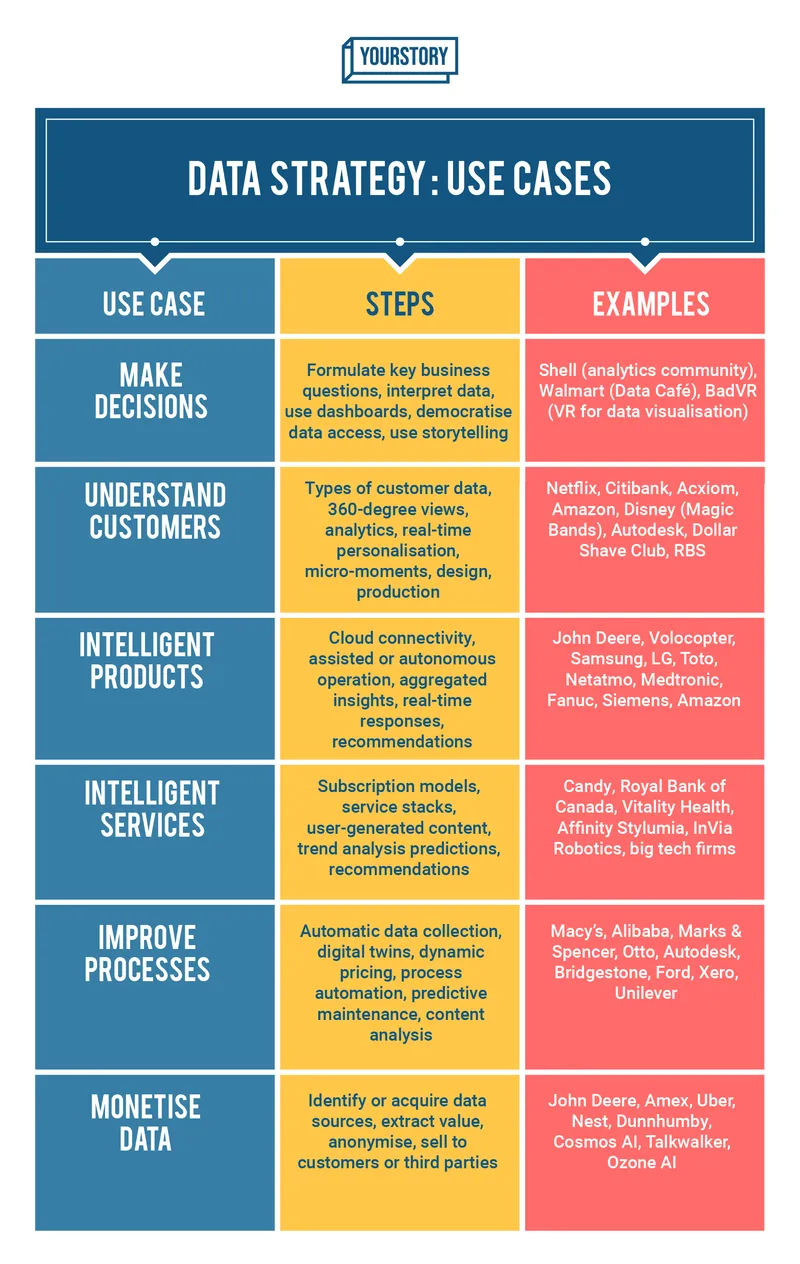
Foundations
The volume and quality of available data are increasing, and powerful tools such as AI/ML are becoming more affordable thanks to cloud computing, IoT, and processing power. This helps computers perceive, understand, learn, and react better.
But it’s easy to get dazzled by the potential of technology. “Data is nothing without strategy,” Bernard cautions. There are also larger ethical, legal, and regulatory issues involved.
Bernard identifies typical misconceptions or barriers around data strategy: We are not a data company, It’s too expensive, We already have enough data, and Our customers aren’t asking for it.
Proactive companies, however, will seize opportunities in the six use cases of data strategy: making decisions, understanding customers, improving processes, making intelligent products and services, and monetizing data.
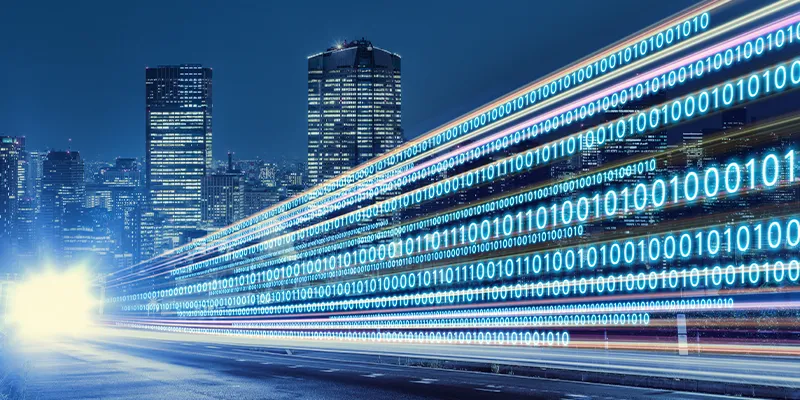
Making decisions
Data insights can augment or automate decisions. Bernard advises companies to first come up with a list of key business questions (KBQs), ten or fewer in number.
“Good questions lead to better answers,” he explains, citing customer traffic, store visits, enquiries, purchases, and repeat visits as simple examples.
Once data is gathered from internal and external sources, it needs to be integrated and analysed to spot trends. While experts create the first sets of digital dashboards, companies should train others as well in how to interpret them and make business recommendations.
Bernard recommends the use of data storytelling here, as well as a role for the “data translator” as a conduit between data experts and decision-makers. Data storytelling helps build compelling cases, and cuts out noise and irrelevant information.
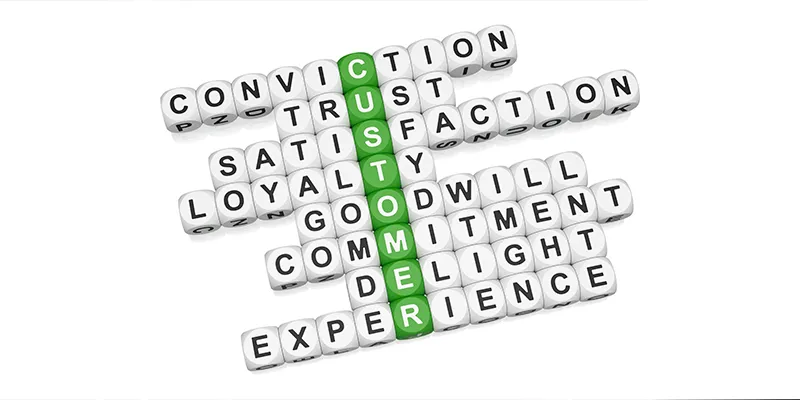
Understanding customers
Data-driven insights help understand what current customers want, use, purchase and assess. This also applies to the broader market.
Bernard defines three types of customer data: personal, behavioural, and attitudinal. Data can be gathered internally (eg. loyalty programmes) or externally (third-party providers like market research firms, government websites, big-tech services).
A broad funnel helps understand customers as well as non-customers. Companies should create a safe and secure environment for customers to share their data, with privacy policies clearly explained.
Such insights can also help generate the right kind of content for targeting campaigns, and designing better offerings as well. Personal connections can be reinforced through real-time personalisation and “micro-moments” or selling opportunities that exist just for a few seconds.
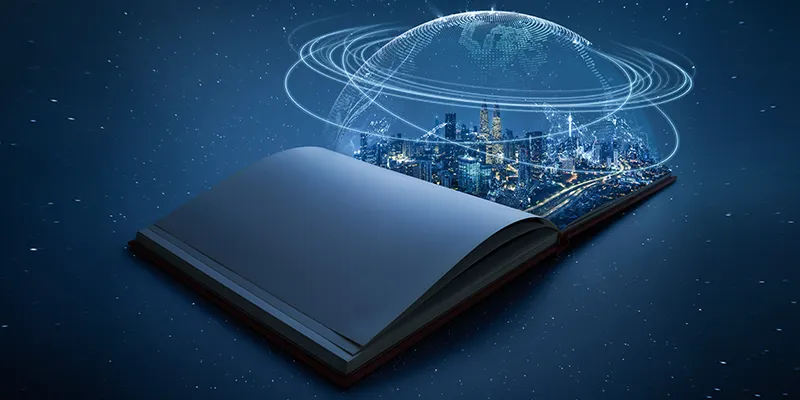
Intelligent services
Tech companies led the service-based shift through the SaaS business model, and other sectors have followed suit. Amazon, Google, Netflix, Uber, and Spotify are exemplars of starting with simple services and then adding layers of new and improved services through data insights and strategy.
Such service transformation includes drone delivery, VR platforms, traffic prediction, on-demand content, user-generated content, personalisation, subscription services, and automatic generation of content. Some platforms for other providers have also observed customer patterns and generated their own offerings.
Bernard cites a range of examples such as Candy (washing machine on subscription), TikTok (determining preferences via AI instead of customer clicks), Royal Bank of Canada (customised savings tips), and Moneybox (stock investments).
Other examples are Vitality Health (app for fitness goals and tracking), Babylon (medical consultation on subscription), RPA Virtual Hospital (with home visits), Affinity (matching clothes to body measurements), Stylumia (apparel trends), and InVia Robotics (subscription for robots).
In fact, AI itself is being offered as a service by tech giants, using their data or proprietary assets. “Every company is a tech company now,” Bernard explains.
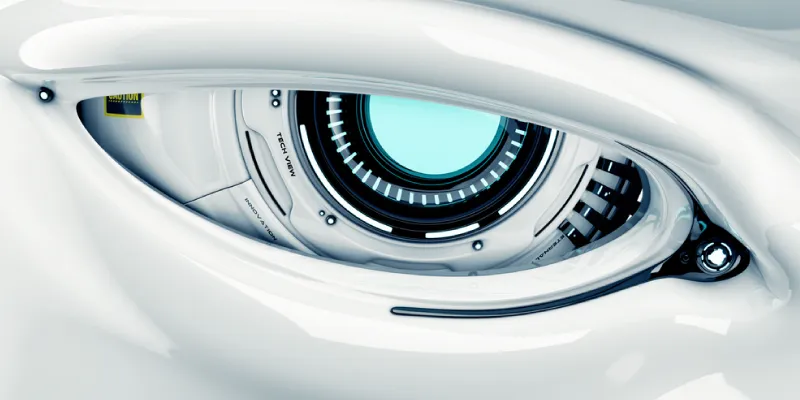
Intelligent products
Smart products can leverage data insights to learn, either through cloud computing or at the edge. The generated intelligence improves customer experience and unlocks new revenue streams.
Such products with data collection and network capabilities have been deployed in smart homes, smart highways, and smart cities. With edge computing, they can learn on their own as well.
Bernard cites a range of examples such as Amazon (Alexa), John Deere (data capture and insights), Volocopter (autonomous drone taxi), Philips (Hue lighting), Samsung (Family Hub fridge), and LG (wine refrigerator).
Other cited products are by Toto (smart toilet with fluid analysis), Netatmo (facial recognition for home security), Medtronic (smart insulin pump), Fanuc (smart robots), Siemens (Mindsphere operating system), and TE Connectivity (smart basketball court).
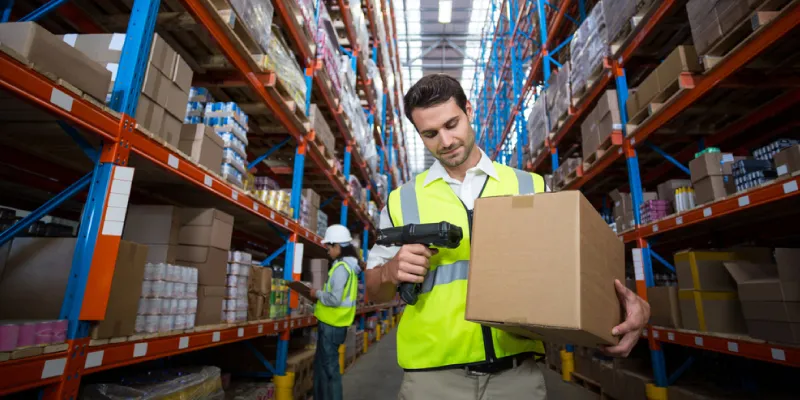
Business processes
Data insights can focus on optimising internal operations as well, to improve productivity and reduce risk and waste. Automation can reduce resources spent on repetitive and non-strategic tasks.
Bernard cites several examples of data strategies from marketing, logistics, manufacturing, finance and HR. Machines and algorithms can automatically collect, analyse and act on data.
Modelling through digital twins can spot bottlenecks and improve performance. Other applications are dynamic pricing, content generation, automation, efficient machine utilisation, predictive maintenance, product development, and resume analysis.
Specific examples include Macy’s (optimised pricing), Amazon (recommendation engine, optimised warehouses), Alibaba (product descriptions), Marks & Spencer (customer chatbots), Otto (reducing customer returns), Autodesk (generative design), Bridgestone (tyre twins), Ford (cobots), Xero (cash flow forecasting), and Unilever (onboarding bot).
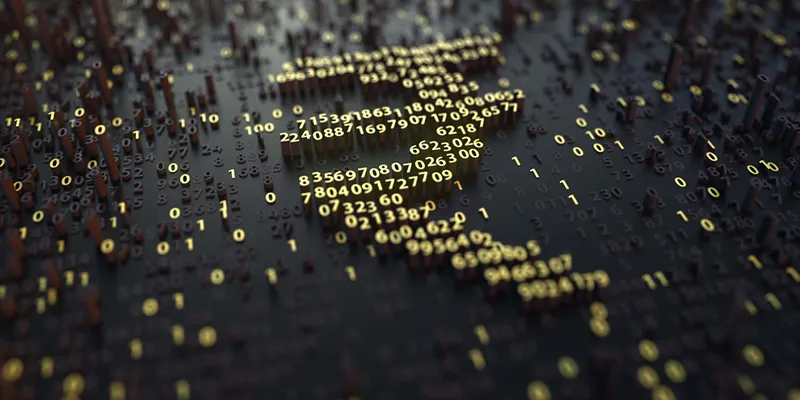
Revenue from data
Companies more advanced in their data strategy also realise that they can sell their data or provide access to it for others. Data strategy drives the business models of the most valuable listed companies of the world, Bernard observes.
Value extraction applies not just to traditional data brokers such as Acxiom, Experian, and Nielsen. The need to get valuable data insights has led to several corporate acquisitions as well, such as Whole Foods (by Amazon), Slack (by Salesforce), LinkedIn (by Microsoft), Nest and DeepMind (by Google).
A number of startups and data-centric entrepreneurs have entered this space. Examples include Dunnhumby (loyalty card programmes for Tesco), Cosmos AI (real-time mobility of people, for Gucci and Walmart), and Talkwalker (audio content listening). Ozone AI even lets customers sell their data to businesses, sidestepping the tech giants.
John Deere sells machine, soil and crop data back to farmers. Apple has partnered with IBM Watson Health for real-time biometric analytics. Amex sells anonymised data on spending patterns, Uber sells traffic and travel data to hotels and municipalities, while Nest sells home energy data to energy providers.
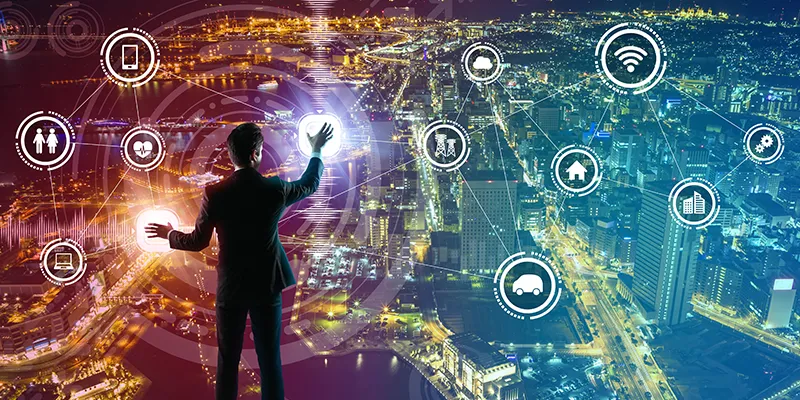
Data strategy
Once data insights for the key business questions and use cases are gathered, Bernard recommends that all stakeholders work together and brainstorm on strategies for implementing each use case so that overlaps and synergies can be optimised.
He presents a tool called the data strategy template, which maps use case scenarios into five categories of activity: data requirements, governance, technology, skills, and implementation. Opportunities can be classified into quick wins or major transformations.
Use case owners, target customers, and success metrics should be worked out. Working together will help come up with an organisation-wide data governance policy.
Bernard provides a wide classification of data: structured/unstructured, internal/external, sensor, transaction/conversation, and text/video. Data governance must cover issues of ethics, privacy, security, ownership, transparency, quality, and bias.
High-quality data is consistent, accurate, unique, valid, complete, and timely. Awareness and action are needed to address potential bias in data and algorithms, and issues like protection or non-solicitation of data about children.
Practices of user consent and compliance with guidelines like GDPR should be enforced. Bernard advises companies to set up an ethics council to address the broader ramifications of their data strategy. This helps safeguard and promote a responsible and proactive data culture in the company.
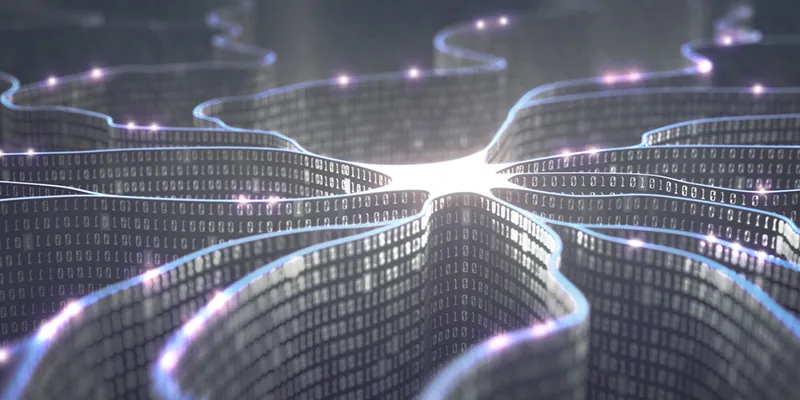
One chapter covers a range of analytics approaches in ML (supervised, unsupervised, reinforcement learning). Deep learning builds layers of weighted neural networks. Such techniques can be applied for text, voice, and sentiment analysis.
Cloud, SaaS and no-code tools are democratising access to these kinds of AI and data. Examples include Salesforce Einstein, and Amazon’s IoT SiteWise.
The four steps to convert data into insights are collection, storage, processing and communication. Examples of specialist platforms include IBM Cognos, John Deere’s Farmsight, and Microsoft's Sports Performance Platform. Snowflake and Datatrade have created data marketplaces.
An important part of the insights phase is data storytelling and visualisation. Tools like Tableau, QlikView, and Lexio are useful as well.
Bernard advises companies to build the six essential data science skills: business, analytics, computing, statistics, creativity, and communication. New talent can be expensive to acquire, and approaches like pairing can work for cross-disciplinary transfer of skills.
Consultants can bridge some of the data science talent gap, and crowdsourcing via platforms like Kaggle also help. Kaggle was tapped during the COVID-19 pandemic as well.
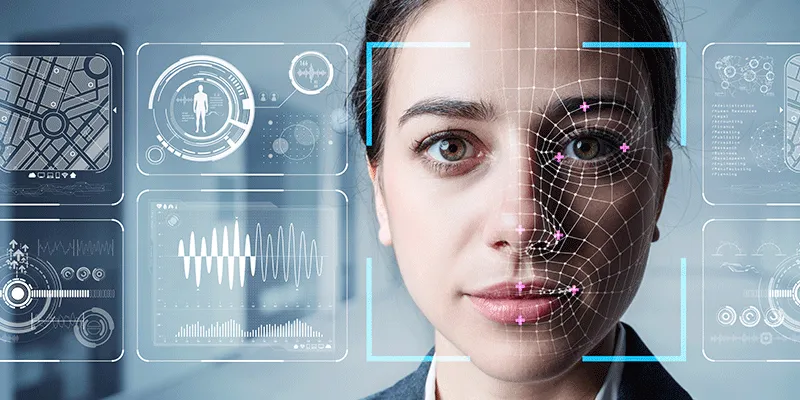
The road ahead
Data strategies should be regularly assessed and updated. Unfortunately, some of them fail due to a lack of clear ownership, communication, alignment, skills, or partner management.
Companies should have a data-first attitude, and not just operate by gut instinct or outdated traditional approaches. Data champions and data advocates play a key role here, Bernard affirms.
New business models can also evolve, but will take courage to implement if there is a shift in direction. Tech trends to watch are edge analytics, quantum computing, and LiFi in terms of data speed and capabilities.
“We’re moving into an era where computers can enhance human knowledge in entirely new ways,” he adds.
“We are facing a range of new emerging technologies that combine the physical, digital and biological worlds,” Bernard observes, pointing to XR, genomics, and blockchain. This impacts all disciplines and will even challenge what it means to be human.
Bernard wraps up with some observations on how data strategies can effectively assist with many of the Sustainable Development Goals (SDGs). This includes ways to improve health, reduce hunger, enhance education, and increase water security and clean energy.
Specific use cases could include improving crop yield, providing remote education, tracking illegal logging and conflict zones, smart and green transport, and predicting weather patterns.
Tech advances should contribute to global human needs rather than just profits. There are also worries about digital feudalism and dominance by the tech elite, Bernard cautions.
The tech community have to take on the roles of responsible custodians as well. “It’s an exciting time for businesses of all shapes and sizes, and data is at the heart of it,” Bernard sums up.
YourStory has also published the pocketbook ‘Proverbs and Quotes for Entrepreneurs: A World of Inspiration for Startups’ as a creative and motivational guide for innovators (downloadable as apps here: Apple, Android).
Edited by Kanishk Singh